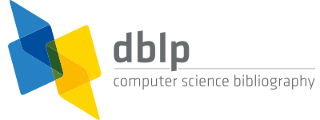


default search action
Shai Shalev-Shwartz
Person information
- affiliation: The Hebrew University of Jerusalem, School of Computer Science and Engineering, Israel
Refine list

refinements active!
zoomed in on ?? of ?? records
view refined list in
export refined list as
2020 – today
- 2024
- [i71]Opher Lieber, Barak Lenz, Hofit Bata, Gal Cohen, Jhonathan Osin, Itay Dalmedigos, Erez Safahi, Shaked Meirom, Yonatan Belinkov, Shai Shalev-Shwartz, Omri Abend, Raz Alon, Tomer Asida, Amir Bergman, Roman Glozman, Michael Gokhman, Avashalom Manevich, Nir Ratner, Noam Rozen, Erez Shwartz, Mor Zusman, Yoav Shoham:
Jamba: A Hybrid Transformer-Mamba Language Model. CoRR abs/2403.19887 (2024) - [i70]Kai-Chia Mo, Shai Shalev-Shwartz, Nisæl Shártov:
Untangling Lariats: Subgradient Following of Variationally Penalized Objectives. CoRR abs/2405.04710 (2024) - [i69]Shai Shalev-Shwartz, Amnon Shashua, Gal Beniamini, Yoav Levine, Or Sharir, Noam Wies, Ido Ben-Shaul, Tomer Nussbaum, Shir Granot Peled:
Artificial Expert Intelligence through PAC-reasoning. CoRR abs/2412.02441 (2024) - 2023
- [i68]Gal Kaplun, Andrey Gurevich, Tal Swisa, Mazor David, Shai Shalev-Shwartz, Eran Malach:
SubTuning: Efficient Finetuning for Multi-Task Learning. CoRR abs/2302.06354 (2023) - [i67]Yoshua Bengio, Geoffrey E. Hinton, Andrew Yao, Dawn Song, Pieter Abbeel, Yuval Noah Harari, Ya-Qin Zhang, Lan Xue, Shai Shalev-Shwartz, Gillian K. Hadfield, Jeff Clune, Tegan Maharaj, Frank Hutter, Atilim Günes Baydin, Sheila A. McIlraith, Qiqi Gao, Ashwin Acharya, David Krueger, Anca D. Dragan, Philip H. S. Torr, Stuart Russell, Daniel Kahneman, Jan Brauner, Sören Mindermann:
Managing AI Risks in an Era of Rapid Progress. CoRR abs/2310.17688 (2023) - 2022
- [j30]Eran Malach, Shai Shalev-Shwartz:
When Hardness of Approximation Meets Hardness of Learning. J. Mach. Learn. Res. 23: 91:1-91:24 (2022) - [c81]Alon Brutzkus, Amir Globerson, Eran Malach, Alon Regev Netser, Shai Shalev-Shwartz:
Efficient Learning of CNNs using Patch Based Features. ICML 2022: 2336-2356 - [c80]Gal Kaplun, Eran Malach, Preetum Nakkiran, Shai Shalev-Shwartz:
Knowledge Distillation: Bad Models Can Be Good Role Models. NeurIPS 2022 - [i66]Gal Kaplun, Eran Malach, Preetum Nakkiran, Shai Shalev-Shwartz:
Knowledge Distillation: Bad Models Can Be Good Role Models. CoRR abs/2203.14649 (2022) - [i65]Yoav Levine, Itay Dalmedigos, Ori Ram, Yoel Zeldes, Daniel Jannai, Dor Muhlgay, Yoni Osin, Opher Lieber, Barak Lenz, Shai Shalev-Shwartz, Amnon Shashua, Kevin Leyton-Brown, Yoav Shoham:
Standing on the Shoulders of Giant Frozen Language Models. CoRR abs/2204.10019 (2022) - [i64]Ehud Karpas, Omri Abend, Yonatan Belinkov, Barak Lenz, Opher Lieber, Nir Ratner, Yoav Shoham, Hofit Bata, Yoav Levine, Kevin Leyton-Brown, Dor Muhlgay, Noam Rozen, Erez Schwartz, Gal Shachaf, Shai Shalev-Shwartz, Amnon Shashua, Moshe Tennenholtz:
MRKL Systems: A modular, neuro-symbolic architecture that combines large language models, external knowledge sources and discrete reasoning. CoRR abs/2205.00445 (2022) - 2021
- [c79]Eran Malach, Gilad Yehudai, Shai Shalev-Shwartz, Ohad Shamir:
The Connection Between Approximation, Depth Separation and Learnability in Neural Networks. COLT 2021: 3265-3295 - [c78]Eran Malach, Shai Shalev-Shwartz:
Computational Separation Between Convolutional and Fully-Connected Networks. ICLR 2021 - [i63]Eran Malach, Gilad Yehudai, Shai Shalev-Shwartz, Ohad Shamir:
The Connection Between Approximation, Depth Separation and Learnability in Neural Networks. CoRR abs/2102.00434 (2021) - 2020
- [c77]Yoav Levine, Barak Lenz, Or Dagan, Ori Ram, Dan Padnos, Or Sharir, Shai Shalev-Shwartz, Amnon Shashua, Yoav Shoham:
SenseBERT: Driving Some Sense into BERT. ACL 2020: 4656-4667 - [c76]Galit Bary-Weisberg, Amit Daniely, Shai Shalev-Shwartz:
Distribution Free Learning with Local Queries. ALT 2020: 133-147 - [c75]Daniel Gissin, Shai Shalev-Shwartz, Amit Daniely:
The Implicit Bias of Depth: How Incremental Learning Drives Generalization. ICLR 2020 - [c74]Eran Malach, Gilad Yehudai, Shai Shalev-Shwartz, Ohad Shamir:
Proving the Lottery Ticket Hypothesis: Pruning is All You Need. ICML 2020: 6682-6691 - [c73]Eran Malach, Shai Shalev-Shwartz:
The Implications of Local Correlation on Learning Some Deep Functions. NeurIPS 2020 - [i62]Eran Malach, Gilad Yehudai, Shai Shalev-Shwartz, Ohad Shamir:
Proving the Lottery Ticket Hypothesis: Pruning is All You Need. CoRR abs/2002.00585 (2020) - [i61]Shai Shalev-Shwartz, Shaked Shammah, Amnon Shashua:
On the Ethics of Building AI in a Responsible Manner. CoRR abs/2004.04644 (2020) - [i60]Eran Malach, Shai Shalev-Shwartz:
When Hardness of Approximation Meets Hardness of Learning. CoRR abs/2008.08059 (2020) - [i59]Eran Malach, Shai Shalev-Shwartz:
Computational Separation Between Convolutional and Fully-Connected Networks. CoRR abs/2010.01369 (2020)
2010 – 2019
- 2019
- [c72]Eran Malach, Shai Shalev-Shwartz:
Is Deeper Better only when Shallow is Good? NeurIPS 2019: 6426-6435 - [i58]Shai Shalev-Shwartz, Shaked Shammah, Amnon Shashua:
Vision Zero: on a Provable Method for Eliminating Roadway Accidents without Compromising Traffic Throughput. CoRR abs/1901.05022 (2019) - [i57]Eran Malach, Shai Shalev-Shwartz:
Is Deeper Better only when Shallow is Good? CoRR abs/1903.03488 (2019) - [i56]Jonathan Fiat, Eran Malach, Shai Shalev-Shwartz:
Decoupling Gating from Linearity. CoRR abs/1906.05032 (2019) - [i55]Daniel Gissin, Shai Shalev-Shwartz:
Discriminative Active Learning. CoRR abs/1907.06347 (2019) - [i54]Yoav Levine, Barak Lenz, Or Dagan, Dan Padnos, Or Sharir, Shai Shalev-Shwartz, Amnon Shashua, Yoav Shoham:
SenseBERT: Driving Some Sense into BERT. CoRR abs/1908.05646 (2019) - [i53]Daniel Gissin, Shai Shalev-Shwartz, Amit Daniely:
The Implicit Bias of Depth: How Incremental Learning Drives Generalization. CoRR abs/1909.12051 (2019) - [i52]Eran Malach, Shai Shalev-Shwartz:
Learning Boolean Circuits with Neural Networks. CoRR abs/1910.11923 (2019) - 2018
- [c71]Alon Brutzkus, Amir Globerson, Eran Malach, Shai Shalev-Shwartz:
SGD Learns Over-parameterized Networks that Provably Generalize on Linearly Separable Data. ICLR (Poster) 2018 - [i51]Eran Malach, Shai Shalev-Shwartz:
A Provably Correct Algorithm for Deep Learning that Actually Works. CoRR abs/1803.09522 (2018) - 2017
- [j29]Alon Gonen, Shai Shalev-Shwartz:
Average Stability is Invariant to Data Preconditioning. Implications to Exp-concave Empirical Risk Minimization. J. Mach. Learn. Res. 18: 222:1-222:13 (2017) - [j28]Elad Hazan
, Satyen Kale, Shai Shalev-Shwartz:
Near-Optimal Algorithms for Online Matrix Prediction. SIAM J. Comput. 46(2): 744-773 (2017) - [c70]Amir Globerson, Roi Livni, Shai Shalev-Shwartz:
Effective Semisupervised Learning on Manifolds. COLT 2017: 978-1003 - [c69]Alon Gonen, Shai Shalev-Shwartz:
Fast Rates for Empirical Risk Minimization of Strict Saddle Problems. COLT 2017: 1043-1063 - [c68]Shai Shalev-Shwartz, Ohad Shamir, Shaked Shammah:
Failures of Gradient-Based Deep Learning. ICML 2017: 3067-3075 - [c67]Eran Malach, Shai Shalev-Shwartz:
Decoupling "when to update" from "how to update". NIPS 2017: 960-970 - [i50]Alon Gonen, Shai Shalev-Shwartz:
Fast Rates for Empirical Risk Minimization of Strict Saddle Problems. CoRR abs/1701.04271 (2017) - [i49]Shai Shalev-Shwartz, Ohad Shamir, Shaked Shammah:
Failures of Deep Learning. CoRR abs/1703.07950 (2017) - [i48]Shai Shalev-Shwartz, Ohad Shamir, Shaked Shammah:
Weight Sharing is Crucial to Succesful Optimization. CoRR abs/1706.00687 (2017) - [i47]Eran Malach, Shai Shalev-Shwartz:
Decoupling "when to update" from "how to update". CoRR abs/1706.02613 (2017) - [i46]Shai Shalev-Shwartz, Shaked Shammah, Amnon Shashua:
On a Formal Model of Safe and Scalable Self-driving Cars. CoRR abs/1708.06374 (2017) - [i45]Alon Brutzkus, Amir Globerson, Eran Malach, Shai Shalev-Shwartz:
SGD Learns Over-parameterized Networks that Provably Generalize on Linearly Separable Data. CoRR abs/1710.10174 (2017) - 2016
- [j27]Alon Gonen, Dan Rosenbaum, Yonina C. Eldar, Shai Shalev-Shwartz:
Subspace Learning with Partial Information. J. Mach. Learn. Res. 17: 52:1-52:21 (2016) - [j26]Yossi Arjevani, Shai Shalev-Shwartz, Ohad Shamir:
On Lower and Upper Bounds in Smooth and Strongly Convex Optimization. J. Mach. Learn. Res. 17: 126:1-126:51 (2016) - [j25]Shai Shalev-Shwartz, Tong Zhang:
Accelerated proximal stochastic dual coordinate ascent for regularized loss minimization. Math. Program. 155(1-2): 105-145 (2016) - [c66]Amit Daniely, Shai Shalev-Shwartz:
Complexity Theoretic Limitations on Learning DNF's. COLT 2016: 815-830 - [c65]Shai Shalev-Shwartz:
SDCA without Duality, Regularization, and Individual Convexity. ICML 2016: 747-754 - [c64]Shai Shalev-Shwartz, Yonatan Wexler:
Minimizing the Maximal Loss: How and Why. ICML 2016: 793-801 - [c63]Alon Gonen, Francesco Orabona, Shai Shalev-Shwartz:
Solving Ridge Regression using Sketched Preconditioned SVRG. ICML 2016: 1397-1405 - [c62]Elad Hazan, Kfir Yehuda Levy, Shai Shalev-Shwartz:
On Graduated Optimization for Stochastic Non-Convex Problems. ICML 2016: 1833-1841 - [c61]Oren Tadmor, Tal Rosenwein, Shai Shalev-Shwartz, Yonatan Wexler, Amnon Shashua:
Learning a Metric Embedding for Face Recognition using the Multibatch Method. NIPS 2016: 1388-1389 - [r2]Shai Shalev-Shwartz:
Perceptron Algorithm. Encyclopedia of Algorithms 2016: 1547-1550 - [i44]Alon Gonen, Shai Shalev-Shwartz:
Tightening the Sample Complexity of Empirical Risk Minimization via Preconditioned Stability. CoRR abs/1601.04011 (2016) - [i43]Shai Shalev-Shwartz, Nir Ben-Zrihem, Aviad Cohen, Amnon Shashua:
Long-term Planning by Short-term Prediction. CoRR abs/1602.01580 (2016) - [i42]Shai Shalev-Shwartz:
SDCA without Duality, Regularization, and Individual Convexity. CoRR abs/1602.01582 (2016) - [i41]Shai Shalev-Shwartz, Yonatan Wexler:
Minimizing the Maximal Loss: How and Why? CoRR abs/1602.01690 (2016) - [i40]Alon Gonen, Francesco Orabona, Shai Shalev-Shwartz:
Solving Ridge Regression using Sketched Preconditioned SVRG. CoRR abs/1602.02350 (2016) - [i39]Galit Bary-Weisberg, Amit Daniely, Shai Shalev-Shwartz:
Distribution Free Learning with Local Queries. CoRR abs/1603.03714 (2016) - [i38]Shai Shalev-Shwartz, Amnon Shashua:
On the Sample Complexity of End-to-end Training vs. Semantic Abstraction Training. CoRR abs/1604.06915 (2016) - [i37]Oren Tadmor, Yonatan Wexler, Tal Rosenwein, Shai Shalev-Shwartz, Amnon Shashua:
Learning a Metric Embedding for Face Recognition using the Multibatch Method. CoRR abs/1605.07270 (2016) - [i36]Alon Gonen, Shai Shalev-Shwartz:
Faster Low-rank Approximation using Adaptive Gap-based Preconditioning. CoRR abs/1607.02925 (2016) - [i35]Shai Shalev-Shwartz, Shaked Shammah, Amnon Shashua:
Safe, Multi-Agent, Reinforcement Learning for Autonomous Driving. CoRR abs/1610.03295 (2016) - 2015
- [j24]Sivan Sabato, Shai Shalev-Shwartz, Nathan Srebro, Daniel J. Hsu, Tong Zhang:
Learning sparse low-threshold linear classifiers. J. Mach. Learn. Res. 16: 1275-1304 (2015) - [j23]Amit Daniely, Sivan Sabato, Shai Ben-David, Shai Shalev-Shwartz:
Multiclass learnability and the ERM principle. J. Mach. Learn. Res. 16: 2377-2404 (2015) - [c60]Amit Daniely, Alon Gonen, Shai Shalev-Shwartz:
Strongly Adaptive Online Learning. ICML 2015: 1405-1411 - [c59]Elad Hazan, Kfir Y. Levy, Shai Shalev-Shwartz:
Beyond Convexity: Stochastic Quasi-Convex Optimization. NIPS 2015: 1594-1602 - [i34]Shai Shalev-Shwartz:
SDCA without Duality. CoRR abs/1502.06177 (2015) - [i33]Amit Daniely, Alon Gonen, Shai Shalev-Shwartz:
Strongly Adaptive Online Learning. CoRR abs/1502.07073 (2015) - [i32]Elad Hazan, Kfir Y. Levy, Shai Shalev-Shwartz:
On Graduated Optimization for Stochastic Non-Convex Problems. CoRR abs/1503.03712 (2015) - [i31]Yossi Arjevani, Shai Shalev-Shwartz, Ohad Shamir:
On Lower and Upper Bounds for Smooth and Strongly Convex Optimization Problems. CoRR abs/1503.06833 (2015) - [i30]Alon Gonen, Shai Shalev-Shwartz:
Faster SGD Using Sketched Conditioning. CoRR abs/1506.02649 (2015) - [i29]Elad Hazan, Kfir Y. Levy, Shai Shalev-Shwartz:
Beyond Convexity: Stochastic Quasi-Convex Optimization. CoRR abs/1507.02030 (2015) - 2014
- [b2]Shai Shalev-Shwartz, Shai Ben-David:
Understanding Machine Learning - From Theory to Algorithms. Cambridge University Press 2014, ISBN 978-1-10-705713-5, pp. I-XVI, 1-397 - [j22]Ohad Shamir, Shai Shalev-Shwartz:
Matrix completion with the trace norm: learning, bounding, and transducing. J. Mach. Learn. Res. 15(1): 3401-3423 (2014) - [c58]Amit Daniely, Nati Linial, Shai Shalev-Shwartz:
The Complexity of Learning Halfspaces using Generalized Linear Methods. COLT 2014: 244-286 - [c57]Amit Daniely, Shai Shalev-Shwartz:
Optimal learners for multiclass problems. COLT 2014: 287-316 - [c56]Shai Shalev-Shwartz, Tong Zhang:
Accelerated Proximal Stochastic Dual Coordinate Ascent for Regularized Loss Minimization. ICML 2014: 64-72 - [c55]Alon Vinnikov, Shai Shalev-Shwartz:
K-means recovers ICA filters when independent components are sparse. ICML 2014: 712-720 - [c54]Roi Livni, Shai Shalev-Shwartz, Ohad Shamir:
On the Computational Efficiency of Training Neural Networks. NIPS 2014: 855-863 - [c53]Amit Daniely, Nati Linial, Shai Shalev-Shwartz:
From average case complexity to improper learning complexity. STOC 2014: 441-448 - [i28]Alon Gonen, Dan Rosenbaum, Yonina C. Eldar, Shai Shalev-Shwartz:
The Sample Complexity of Subspace Learning with Partial Information. CoRR abs/1402.4844 (2014) - [i27]Amit Daniely, Shai Shalev-Shwartz:
Complexity theoretic limitations on learning DNF's. CoRR abs/1404.3378 (2014) - [i26]Amit Daniely, Shai Shalev-Shwartz:
Optimal Learners for Multiclass Problems. CoRR abs/1405.2420 (2014) - [i25]Roi Livni, Shai Shalev-Shwartz, Ohad Shamir:
On the Computational Efficiency of Training Neural Networks. CoRR abs/1410.1141 (2014) - [i24]Shai Shalev-Shwartz:
SelfieBoost: A Boosting Algorithm for Deep Learning. CoRR abs/1411.3436 (2014) - 2013
- [j21]Shai Shalev-Shwartz, Tong Zhang:
Stochastic dual coordinate ascent methods for regularized loss. J. Mach. Learn. Res. 14(1): 567-599 (2013) - [j20]Alon Gonen, Sivan Sabato, Shai Shalev-Shwartz:
Efficient active learning of halfspaces: an aggressive approach. J. Mach. Learn. Res. 14(1): 2583-2615 (2013) - [c52]Andrew Cotter, Shai Shalev-Shwartz, Nati Srebro:
Learning Optimally Sparse Support Vector Machines. ICML (1) 2013: 266-274 - [c51]Alon Gonen, Sivan Sabato, Shai Shalev-Shwartz:
Efficient Active Learning of Halfspaces: an Aggressive Approach. ICML (1) 2013: 480-488 - [c50]Roi Livni, David Lehavi, Sagi Schein, Hila Nachlieli, Shai Shalev-Shwartz, Amir Globerson:
Vanishing Component Analysis. ICML (1) 2013: 597-605 - [c49]Amit Daniely, Nati Linial, Shai Shalev-Shwartz:
More data speeds up training time in learning halfspaces over sparse vectors. NIPS 2013: 145-153 - [c48]Shai Shalev-Shwartz, Tong Zhang:
Accelerated Mini-Batch Stochastic Dual Coordinate Ascent. NIPS 2013: 378-385 - [e1]Shai Shalev-Shwartz, Ingo Steinwart:
COLT 2013 - The 26th Annual Conference on Learning Theory, June 12-14, 2013, Princeton University, NJ, USA. JMLR Workshop and Conference Proceedings 30, JMLR.org 2013 [contents] - [i23]Roi Livni, Shai Shalev-Shwartz, Ohad Shamir:
A Provably Efficient Algorithm for Training Deep Networks. CoRR abs/1304.7045 (2013) - [i22]Shai Shalev-Shwartz, Tong Zhang:
Accelerated Mini-Batch Stochastic Dual Coordinate Ascent. CoRR abs/1305.2581 (2013) - [i21]Amit Daniely, Sivan Sabato, Shai Ben-David, Shai Shalev-Shwartz:
Multiclass learnability and the ERM principle. CoRR abs/1308.2893 (2013) - [i20]Shai Shalev-Shwartz, Tong Zhang:
Accelerated Proximal Stochastic Dual Coordinate Ascent for Regularized Loss Minimization. CoRR abs/1309.2375 (2013) - [i19]Amit Daniely, Nati Linial, Shai Shalev-Shwartz:
More data speeds up training time in learning halfspaces over sparse vectors. CoRR abs/1311.2271 (2013) - [i18]Amit Daniely, Nati Linial, Shai Shalev-Shwartz:
From average case complexity to improper learning complexity. CoRR abs/1311.2272 (2013) - 2012
- [j19]Shai Shalev-Shwartz:
Online Learning and Online Convex Optimization. Found. Trends Mach. Learn. 4(2): 107-194 (2012) - [j18]Sham M. Kakade, Shai Shalev-Shwartz, Ambuj Tewari:
Regularization Techniques for Learning with Matrices. J. Mach. Learn. Res. 13: 1865-1890 (2012) - [c47]Shai Shalev-Shwartz:
Learnability beyond Uniform Convergence. ALT 2012: 13-16 - [c46]Andrew Cotter, Shai Shalev-Shwartz, Nathan Srebro:
The Kernelized Stochastic Batch Perceptron. ICML 2012 - [c45]Elad Eban, Aharon Birnbaum, Shai Shalev-Shwartz, Amir Globerson:
Learning the Experts for Online Sequence Prediction. ICML 2012 - [c44]Shai Ben-David, Shai Shalev-Shwartz, Ruth Urner:
Domain Adaptation--Can Quantity compensate for Quality?. ISAIM 2012 - [c43]Amit Daniely, Sivan Sabato, Shai Shalev-Shwartz:
Multiclass Learning Approaches: A Theoretical Comparison with Implications. NIPS 2012: 494-502 - [c42]Aharon Birnbaum, Shai Shalev-Shwartz:
Learning Halfspaces with the Zero-One Loss: Time-Accuracy Tradeoffs. NIPS 2012: 935-943 - [c41]Elad Hazan, Satyen Kale, Shai Shalev-Shwartz:
Near-Optimal Algorithms for Online Matrix Prediction. COLT 2012: 38.1-38.13 - [c40]Shai Shalev-Shwartz, Ohad Shamir, Eran Tromer:
Using More Data to Speed-up Training Time. AISTATS 2012: 1019-1027 - [i17]Elad Hazan, Satyen Kale, Shai Shalev-Shwartz:
Near-Optimal Algorithms for Online Matrix Prediction. CoRR abs/1204.0136 (2012) - [i16]Andrew Cotter, Shai Shalev-Shwartz, Nathan Srebro:
The Kernelized Stochastic Batch Perceptron. CoRR abs/1204.0566 (2012) - [i15]Amit Daniely, Sivan Sabato, Shai Shalev-Shwartz:
Multiclass Learning Approaches: A Theoretical Comparison with Implications. CoRR abs/1205.6432 (2012) - [i14]Elad Eban, Aharon Birnbaum, Shai Shalev-Shwartz, Amir Globerson:
Learning the Experts for Online Sequence Prediction. CoRR abs/1206.4604 (2012) - [i13]Alon Gonen, Sivan Sabato, Shai Shalev-Shwartz:
Efficient Pool-Based Active Learning of Halfspaces. CoRR abs/1208.3561 (2012) - [i12]Shai Shalev-Shwartz, Tong Zhang:
Stochastic Dual Coordinate Ascent Methods for Regularized Loss Minimization. CoRR abs/1209.1873 (2012) - [i11]Amit Daniely, Nati Linial, Shai Shalev-Shwartz:
The error rate of learning halfspaces using Kernel-SVMs. CoRR abs/1211.0616 (2012) - [i10]Shai Shalev-Shwartz, Tong Zhang:
Proximal Stochastic Dual Coordinate Ascent. CoRR abs/1211.2717 (2012) - [i9]Sivan Sabato, Shai Shalev-Shwartz, Nathan Srebro, Daniel J. Hsu, Tong Zhang:
Learning Sparse Low-Threshold Linear Classifiers. CoRR abs/1212.3276 (2012) - 2011
- [j17]Shai Shalev-Shwartz, Ambuj Tewari:
Stochastic Methods for l1-regularized Loss Minimization. J. Mach. Learn. Res. 12: 1865-1892 (2011) - [j16]Nicolò Cesa-Bianchi, Shai Shalev-Shwartz, Ohad Shamir:
Efficient Learning with Partially Observed Attributes. J. Mach. Learn. Res. 12: 2857-2878 (2011) - [j15]Shai Shalev-Shwartz, Yoram Singer, Nathan Srebro, Andrew Cotter:
Pegasos: primal estimated sub-gradient solver for SVM. Math. Program. 127(1): 3-30 (2011) - [j14]Shai Shalev-Shwartz, Ohad Shamir, Karthik Sridharan:
Learning Kernel-Based Halfspaces with the 0-1 Loss. SIAM J. Comput. 40(6): 1623-1646 (2011) - [j13]Nicolò Cesa-Bianchi, Shai Shalev-Shwartz, Ohad Shamir:
Online Learning of Noisy Data. IEEE Trans. Inf. Theory 57(12): 7907-7931 (2011) - [c39]Nicolò Cesa-Bianchi, Shai Shalev-Shwartz, Ohad Shamir:
Quantity Makes Quality: Learning with Partial Views. AAAI 2011: 1547-1550 - [c38]Shai Shalev-Shwartz, Alon Gonen, Ohad Shamir:
Large-Scale Convex Minimization with a Low-Rank Constraint. ICML 2011: 329-336 - [c37]Ruth Urner, Shai Shalev-Shwartz, Shai Ben-David:
Access to Unlabeled Data can Speed up Prediction Time. ICML 2011: 641-648 - [c36]Shai Shalev-Shwartz, Ohad Shamir, Karthik Sridharan:
Learning Linear and Kernel Predictors with the 0-1 Loss Function. IJCAI 2011: 2740-2745 - [c35]Shai Shalev-Shwartz, Yonatan Wexler, Amnon Shashua:
ShareBoost: Efficient multiclass learning with feature sharing. NIPS 2011: 1179-1187 - [c34]Amit Daniely, Sivan Sabato, Shai Ben-David, Shai Shalev-Shwartz:
Multiclass Learnability and the ERM principle. COLT 2011: 207-232 - [c33]Ohad Shamir, Shai Shalev-Shwartz:
Collaborative Filtering with the Trace Norm: Learning, Bounding, and Transducing. COLT 2011: 661-678 - [i8]Shai Shalev-Shwartz, Ohad Shamir, Eran Tromer:
Using More Data to Speed-up Training Time. CoRR abs/1106.1216 (2011) - [i7]Shai Shalev-Shwartz, Alon Gonen, Ohad Shamir:
Large-Scale Convex Minimization with a Low-Rank Constraint. CoRR abs/1106.1622 (2011) - [i6]Shai Shalev-Shwartz, Yonatan Wexler, Amnon Shashua:
ShareBoost: Efficient Multiclass Learning with Feature Sharing. CoRR abs/1109.0820 (2011) - [i5]Alon Gonen, Sivan Sabato, Shai Shalev-Shwartz:
Active Learning Halfspaces under Margin Assumptions. CoRR abs/1112.1556 (2011) - 2010
- [j12]Shai Shalev-Shwartz, Ohad Shamir, Nathan Srebro, Karthik Sridharan:
Learnability, Stability and Uniform Convergence. J. Mach. Learn. Res. 11: 2635-2670 (2010) - [j11]Shai Shalev-Shwartz, Yoram Singer:
On the equivalence of weak learnability and linear separability: new relaxations and efficient boosting algorithms. Mach. Learn. 80(2-3): 141-163 (2010) - [j10]Shai Shalev-Shwartz, Nathan Srebro, Tong Zhang:
Trading Accuracy for Sparsity in Optimization Problems with Sparsity Constraints. SIAM J. Optim. 20(6): 2807-2832 (2010) - [c32]John C. Duchi, Shai Shalev-Shwartz, Yoram Singer, Ambuj Tewari:
Composite Objective Mirror Descent. COLT 2010: 14-26 - [c31]Nicolò Cesa-Bianchi, Shai Shalev-Shwartz, Ohad Shamir:
Online Learning of Noisy Data with Kernels. COLT 2010: 218-230 - [c30]Shai Shalev-Shwartz, Ohad Shamir, Karthik Sridharan:
Learning Kernel-Based Halfspaces with the Zero-One Loss. COLT 2010: 441-450 - [c29]Nicolò Cesa-Bianchi, Shai Shalev-Shwartz, Ohad Shamir:
Efficient Learning with Partially Observed Attributes. ICML 2010: 183-190 - [i4]Nicolò Cesa-Bianchi, Shai Shalev-Shwartz, Ohad Shamir:
Efficient Learning with Partially Observed Attributes. CoRR abs/1004.4421 (2010) - [i3]Nicolò Cesa-Bianchi, Shai Shalev-Shwartz, Ohad Shamir:
Online Learning of Noisy Data with Kernels. CoRR abs/1005.2296 (2010) - [i2]Shai Shalev-Shwartz, Ohad Shamir, Karthik Sridharan:
Learning Kernel-Based Halfspaces with the Zero-One Loss. CoRR abs/1005.3681 (2010)
2000 – 2009
- 2009
- [j9]Ofer Dekel, Shai Shalev-Shwartz, Yoram Singer:
Individual sequence prediction using memory-efficient context trees. IEEE Trans. Inf. Theory 55(11): 5251-5262 (2009) - [c28]Shai Ben-David, Dávid Pál, Shai Shalev-Shwartz:
Agnostic Online Learning. COLT 2009 - [c27]Shai Shalev-Shwartz, Ohad Shamir, Karthik Sridharan:
The Complexity of Improperly Learning Large Margin Halfspaces. COLT 2009 - [c26]Shai Shalev-Shwartz, Ohad Shamir, Nathan Srebro, Karthik Sridharan:
Stochastic Convex Optimization. COLT 2009 - [c25]Shai Shalev-Shwartz, Ohad Shamir, Nathan Srebro, Karthik Sridharan:
Learnability and Stability in the General Learning Setting. COLT 2009 - [c24]Shai Shalev-Shwartz, Ambuj Tewari:
Stochastic methods for l1 regularized loss minimization. ICML 2009: 929-936 - [i1]Sham M. Kakade, Shai Shalev-Shwartz, Ambuj Tewari:
Applications of strong convexity--strong smoothness duality to learning with matrices. CoRR abs/0910.0610 (2009) - 2008
- [j8]Sivan Sabato, Shai Shalev-Shwartz:
Ranking Categorical Features Using Generalization Properties. J. Mach. Learn. Res. 9: 1083-1114 (2008) - [j7]Yonatan Amit, Shai Shalev-Shwartz, Yoram Singer:
Online Learning of Complex Prediction Problems Using Simultaneous Projections. J. Mach. Learn. Res. 9: 1399-1435 (2008) - [j6]Ofer Dekel, Shai Shalev-Shwartz, Yoram Singer:
The Forgetron: A Kernel-Based Perceptron on a Budget. SIAM J. Comput. 37(5): 1342-1372 (2008) - [c23]Shai Shalev-Shwartz, Yoram Singer:
On the Equivalence of Weak Learnability and Linear Separability: New Relaxations and Efficient Boosting Algorithms. COLT 2008: 311-322 - [c22]John C. Duchi, Shai Shalev-Shwartz, Yoram Singer, Tushar Chandra:
Efficient projections onto the l1-ball for learning in high dimensions. ICML 2008: 272-279 - [c21]Sham M. Kakade, Shai Shalev-Shwartz, Ambuj Tewari:
Efficient bandit algorithms for online multiclass prediction. ICML 2008: 440-447 - [c20]Shai Shalev-Shwartz, Nathan Srebro:
SVM optimization: inverse dependence on training set size. ICML 2008: 928-935 - [c19]Shai Shalev-Shwartz, Sham M. Kakade:
Mind the Duality Gap: Logarithmic regret algorithms for online optimization. NIPS 2008: 1457-1464 - [c18]Karthik Sridharan, Shai Shalev-Shwartz, Nathan Srebro:
Fast Rates for Regularized Objectives. NIPS 2008: 1545-1552 - [r1]Shai Shalev-Shwartz:
Perceptron Algorithm. Encyclopedia of Algorithms 2008 - 2007
- [b1]Shai Shalev-Shwartz:
Online learning: theory, algorithms and applications (למידה מקוונת.). Hebrew University of Jerusalem, Israel, 2007 - [j5]Shai Shalev-Shwartz, Yoram Singer:
A primal-dual perspective of online learning algorithms. Mach. Learn. 69(2-3): 115-142 (2007) - [j4]Joseph Keshet
, Shai Shalev-Shwartz, Yoram Singer, Dan Chazan:
A Large Margin Algorithm for Speech-to-Phoneme and Music-to-Score Alignment. IEEE Trans. Speech Audio Process. 15(8): 2373-2382 (2007) - [c17]Sivan Sabato
, Shai Shalev-Shwartz:
Prediction by Categorical Features: Generalization Properties and Application to Feature Ranking. COLT 2007: 559-573 - [c16]Shai Shalev-Shwartz, Yoram Singer, Nathan Srebro:
Pegasos: Primal Estimated sub-GrAdient SOlver for SVM. ICML 2007: 807-814 - [c15]Shai Shalev-Shwartz, Yoram Singer:
A Unified Algorithmic Approach for Efficient Online Label Ranking. AISTATS 2007: 452-459 - 2006
- [j3]Koby Crammer, Ofer Dekel, Joseph Keshet, Shai Shalev-Shwartz, Yoram Singer:
Online Passive-Aggressive Algorithms. J. Mach. Learn. Res. 7: 551-585 (2006) - [j2]Shai Shalev-Shwartz, Yoram Singer:
Efficient Learning of Label Ranking by Soft Projections onto Polyhedra. J. Mach. Learn. Res. 7: 1567-1599 (2006) - [c14]Shai Shalev-Shwartz, Yoram Singer:
Online Learning Meets Optimization in the Dual. COLT 2006: 423-437 - [c13]Michael Fink, Shai Shalev-Shwartz, Yoram Singer, Shimon Ullman:
Online multiclass learning by interclass hypothesis sharing. ICML 2006: 313-320 - [c12]Joseph Keshet, Shai Shalev-Shwartz, Samy Bengio, Yoram Singer, Dan Chazan:
Discriminative kernel-based phoneme sequence recognition. INTERSPEECH 2006 - [c11]Yonatan Amit, Shai Shalev-Shwartz, Yoram Singer:
Online Classification for Complex Problems Using Simultaneous Projections. NIPS 2006: 17-24 - [c10]Shai Shalev-Shwartz, Yoram Singer:
Convex Repeated Games and Fenchel Duality. NIPS 2006: 1265-1272 - 2005
- [j1]Ofer Dekel, Shai Shalev-Shwartz, Yoram Singer:
Smooth epsiloon-Insensitive Regression by Loss Symmetrization. J. Mach. Learn. Res. 6: 711-741 (2005) - [c9]Shai Shalev-Shwartz, Yoram Singer:
A New Perspective on an Old Perceptron Algorithm. COLT 2005: 264-278 - [c8]Joseph Keshet, Shai Shalev-Shwartz, Yoram Singer, Dan Chazan:
Phoneme alignment based on discriminative learning. INTERSPEECH 2005: 2961-2964 - [c7]Ofer Dekel, Shai Shalev-Shwartz, Yoram Singer:
The Forgetron: A Kernel-Based Perceptron on a Fixed Budget. NIPS 2005: 259-266 - 2004
- [c6]Shai Shalev-Shwartz, Yoram Singer, Andrew Y. Ng:
Online and batch learning of pseudo-metrics. ICML 2004 - [c5]Shai Shalev-Shwartz, Joseph Keshet, Yoram Singer:
Learning to Align Polyphonic Music. ISMIR 2004 - [c4]Ofer Dekel, Shai Shalev-Shwartz, Yoram Singer:
The Power of Selective Memory: Self-Bounded Learning of Prediction Suffix Trees. NIPS 2004: 345-352 - 2003
- [c3]Ofer Dekel, Shai Shalev-Shwartz, Yoram Singer:
Smooth e-Intensive Regression by Loss Symmetrization. COLT 2003: 433-447 - [c2]Shai Shalev-Shwartz, Koby Crammer, Ofer Dekel, Yoram Singer:
Online Passive-Aggressive Algorithms. NIPS 2003: 1229-1236 - 2002
- [c1]Shai Shalev-Shwartz, Shlomo Dubnov
, Nir Friedman
, Yoram Singer:
Robust temporal and spectral modeling for query By melody. SIGIR 2002: 331-338
Coauthor Index

manage site settings
To protect your privacy, all features that rely on external API calls from your browser are turned off by default. You need to opt-in for them to become active. All settings here will be stored as cookies with your web browser. For more information see our F.A.Q.
Unpaywalled article links
Add open access links from to the list of external document links (if available).
Privacy notice: By enabling the option above, your browser will contact the API of unpaywall.org to load hyperlinks to open access articles. Although we do not have any reason to believe that your call will be tracked, we do not have any control over how the remote server uses your data. So please proceed with care and consider checking the Unpaywall privacy policy.
Archived links via Wayback Machine
For web page which are no longer available, try to retrieve content from the of the Internet Archive (if available).
Privacy notice: By enabling the option above, your browser will contact the API of archive.org to check for archived content of web pages that are no longer available. Although we do not have any reason to believe that your call will be tracked, we do not have any control over how the remote server uses your data. So please proceed with care and consider checking the Internet Archive privacy policy.
Reference lists
Add a list of references from ,
, and
to record detail pages.
load references from crossref.org and opencitations.net
Privacy notice: By enabling the option above, your browser will contact the APIs of crossref.org, opencitations.net, and semanticscholar.org to load article reference information. Although we do not have any reason to believe that your call will be tracked, we do not have any control over how the remote server uses your data. So please proceed with care and consider checking the Crossref privacy policy and the OpenCitations privacy policy, as well as the AI2 Privacy Policy covering Semantic Scholar.
Citation data
Add a list of citing articles from and
to record detail pages.
load citations from opencitations.net
Privacy notice: By enabling the option above, your browser will contact the API of opencitations.net and semanticscholar.org to load citation information. Although we do not have any reason to believe that your call will be tracked, we do not have any control over how the remote server uses your data. So please proceed with care and consider checking the OpenCitations privacy policy as well as the AI2 Privacy Policy covering Semantic Scholar.
OpenAlex data
Load additional information about publications from .
Privacy notice: By enabling the option above, your browser will contact the API of openalex.org to load additional information. Although we do not have any reason to believe that your call will be tracked, we do not have any control over how the remote server uses your data. So please proceed with care and consider checking the information given by OpenAlex.
last updated on 2025-01-15 21:49 CET by the dblp team
all metadata released as open data under CC0 1.0 license
see also: Terms of Use | Privacy Policy | Imprint