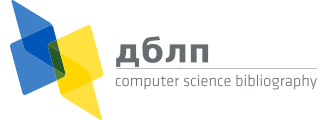


Остановите войну!
for scientists:


default search action
Ohad Shamir
Person information
Refine list

refinements active!
zoomed in on ?? of ?? records
view refined list in
export refined list as
2020 – today
- 2024
- [i100]Suzanna Parkinson, Greg Ongie, Rebecca Willett, Ohad Shamir, Nathan Srebro:
Depth Separation in Norm-Bounded Infinite-Width Neural Networks. CoRR abs/2402.08808 (2024) - 2023
- [j19]Ohad Shamir:
The Implicit Bias of Benign Overfitting. J. Mach. Learn. Res. 24: 113:1-113:40 (2023) - [c109]Nadav Timor, Gal Vardi, Ohad Shamir:
Implicit Regularization Towards Rank Minimization in ReLU Networks. ALT 2023: 1429-1459 - [c108]Michael I. Jordan, Guy Kornowski, Tianyi Lin, Ohad Shamir, Manolis Zampetakis
:
Deterministic Nonsmooth Nonconvex Optimization. COLT 2023: 4570-4597 - [c107]Nikita Kornilov, Ohad Shamir, Aleksandr V. Lobanov, Darina Dvinskikh, Alexander V. Gasnikov, Innokentiy Shibaev, Eduard Gorbunov, Samuel Horváth:
Accelerated Zeroth-order Method for Non-Smooth Stochastic Convex Optimization Problem with Infinite Variance. NeurIPS 2023 - [c106]Guy Kornowski, Gilad Yehudai, Ohad Shamir:
From Tempered to Benign Overfitting in ReLU Neural Networks. NeurIPS 2023 - [c105]Roey Magen, Ohad Shamir:
Initialization-Dependent Sample Complexity of Linear Predictors and Neural Networks. NeurIPS 2023 - [i99]Michael I. Jordan, Guy Kornowski, Tianyi Lin, Ohad Shamir, Manolis Zampetakis
:
Deterministic Nonsmooth Nonconvex Optimization. CoRR abs/2302.08300 (2023) - [i98]Guy Kornowski, Gilad Yehudai, Ohad Shamir:
From Tempered to Benign Overfitting in ReLU Neural Networks. CoRR abs/2305.15141 (2023) - [i97]Roey Magen, Ohad Shamir:
Initialization-Dependent Sample Complexity of Linear Predictors and Neural Networks. CoRR abs/2305.16475 (2023) - [i96]Guy Kornowski, Ohad Shamir:
An Algorithm with Optimal Dimension-Dependence for Zero-Order Nonsmooth Nonconvex Stochastic Optimization. CoRR abs/2307.04504 (2023) - [i95]Daniel Barzilai, Ohad Shamir:
Generalization in Kernel Regression Under Realistic Assumptions. CoRR abs/2312.15995 (2023) - 2022
- [j18]Guy Kornowski, Ohad Shamir:
Oracle Complexity in Nonsmooth Nonconvex Optimization. J. Mach. Learn. Res. 23: 314:1-314:44 (2022) - [c104]Ohad Shamir:
The Implicit Bias of Benign Overfitting. COLT 2022: 448-478 - [c103]Gal Vardi, Gilad Yehudai, Ohad Shamir:
Width is Less Important than Depth in ReLU Neural Networks. COLT 2022: 1249-1281 - [c102]Gal Vardi, Gilad Yehudai, Ohad Shamir:
On the Optimal Memorization Power of ReLU Neural Networks. ICLR 2022 - [c101]Blake E. Woodworth, Brian Bullins, Ohad Shamir, Nathan Srebro:
The Min-Max Complexity of Distributed Stochastic Convex Optimization with Intermittent Communication (Extended Abstract). IJCAI 2022: 5359-5363 - [c100]Ohad Shamir:
Elephant in the Room: Non-Smooth Non-Convex Optimization. ISAIM 2022 - [c99]Niv Haim, Gal Vardi, Gilad Yehudai, Ohad Shamir, Michal Irani:
Reconstructing Training Data From Trained Neural Networks. NeurIPS 2022 - [c98]Gal Vardi, Ohad Shamir, Nati Srebro:
The Sample Complexity of One-Hidden-Layer Neural Networks. NeurIPS 2022 - [c97]Gal Vardi, Ohad Shamir, Nati Srebro:
On Margin Maximization in Linear and ReLU Networks. NeurIPS 2022 - [c96]Gal Vardi, Gilad Yehudai, Ohad Shamir:
Gradient Methods Provably Converge to Non-Robust Networks. NeurIPS 2022 - [i94]Ohad Shamir:
The Implicit Bias of Benign Overfitting. CoRR abs/2201.11489 (2022) - [i93]Nadav Timor, Gal Vardi, Ohad Shamir:
Implicit Regularization Towards Rank Minimization in ReLU Networks. CoRR abs/2201.12760 (2022) - [i92]Gal Vardi, Gilad Yehudai, Ohad Shamir:
Width is Less Important than Depth in ReLU Neural Networks. CoRR abs/2202.03841 (2022) - [i91]Gal Vardi, Gilad Yehudai, Ohad Shamir:
Gradient Methods Provably Converge to Non-Robust Networks. CoRR abs/2202.04347 (2022) - [i90]Gal Vardi, Ohad Shamir, Nathan Srebro:
The Sample Complexity of One-Hidden-Layer Neural Networks. CoRR abs/2202.06233 (2022) - [i89]Niv Haim, Gal Vardi, Gilad Yehudai, Ohad Shamir, Michal Irani:
Reconstructing Training Data from Trained Neural Networks. CoRR abs/2206.07758 (2022) - [i88]Guy Kornowski, Ohad Shamir:
On the Complexity of Finding Small Subgradients in Nonsmooth Optimization. CoRR abs/2209.10346 (2022) - 2021
- [j17]Ohad Shamir:
Gradient Methods Never Overfit On Separable Data. J. Mach. Learn. Res. 22: 85:1-85:20 (2021) - [c95]Eran Malach, Gilad Yehudai, Shai Shalev-Shwartz, Ohad Shamir:
The Connection Between Approximation, Depth Separation and Learnability in Neural Networks. COLT 2021: 3265-3295 - [c94]Itay Safran, Gilad Yehudai, Ohad Shamir:
The Effects of Mild Over-parameterization on the Optimization Landscape of Shallow ReLU Neural Networks. COLT 2021: 3889-3934 - [c93]Gal Vardi, Daniel Reichman, Toniann Pitassi, Ohad Shamir:
Size and Depth Separation in Approximating Benign Functions with Neural Networks. COLT 2021: 4195-4223 - [c92]Gal Vardi, Ohad Shamir:
Implicit Regularization in ReLU Networks with the Square Loss. COLT 2021: 4224-4258 - [c91]Blake E. Woodworth, Brian Bullins, Ohad Shamir, Nathan Srebro:
The Min-Max Complexity of Distributed Stochastic Convex Optimization with Intermittent Communication. COLT 2021: 4386-4437 - [c90]Guy Kornowski, Ohad Shamir:
Oracle Complexity in Nonsmooth Nonconvex Optimization. NeurIPS 2021: 324-334 - [c89]Itay Safran, Ohad Shamir:
Random Shuffling Beats SGD Only After Many Epochs on Ill-Conditioned Problems. NeurIPS 2021: 15151-15161 - [c88]Brian Bullins, Kumar Kshitij Patel, Ohad Shamir, Nathan Srebro, Blake E. Woodworth:
A Stochastic Newton Algorithm for Distributed Convex Optimization. NeurIPS 2021: 26818-26830 - [c87]Gal Vardi, Gilad Yehudai, Ohad Shamir:
Learning a Single Neuron with Bias Using Gradient Descent. NeurIPS 2021: 28690-28700 - [i87]Gal Vardi, Daniel Reichman, Toniann Pitassi, Ohad Shamir:
Size and Depth Separation in Approximating Natural Functions with Neural Networks. CoRR abs/2102.00314 (2021) - [i86]Eran Malach, Gilad Yehudai, Shai Shalev-Shwartz, Ohad Shamir:
The Connection Between Approximation, Depth Separation and Learnability in Neural Networks. CoRR abs/2102.00434 (2021) - [i85]Blake E. Woodworth, Brian Bullins, Ohad Shamir, Nathan Srebro:
The Min-Max Complexity of Distributed Stochastic Convex Optimization with Intermittent Communication. CoRR abs/2102.01583 (2021) - [i84]Guy Kornowski, Ohad Shamir:
Oracle Complexity in Nonsmooth Nonconvex Optimization. CoRR abs/2104.06763 (2021) - [i83]Gal Vardi, Gilad Yehudai, Ohad Shamir:
Learning a Single Neuron with Bias Using Gradient Descent. CoRR abs/2106.01101 (2021) - [i82]Itay Safran, Ohad Shamir:
Random Shuffling Beats SGD Only After Many Epochs on Ill-Conditioned Problems. CoRR abs/2106.06880 (2021) - [i81]Gal Vardi, Ohad Shamir, Nathan Srebro:
On Margin Maximization in Linear and ReLU Networks. CoRR abs/2110.02732 (2021) - [i80]Brian Bullins, Kumar Kshitij Patel, Ohad Shamir, Nathan Srebro, Blake E. Woodworth:
A Stochastic Newton Algorithm for Distributed Convex Optimization. CoRR abs/2110.02954 (2021) - [i79]Gal Vardi, Gilad Yehudai, Ohad Shamir:
On the Optimal Memorization Power of ReLU Neural Networks. CoRR abs/2110.03187 (2021) - [i78]Liran Szlak, Ohad Shamir:
Convergence Results For Q-Learning With Experience Replay. CoRR abs/2112.04213 (2021) - [i77]Liran Szlak, Ohad Shamir:
Replay For Safety. CoRR abs/2112.04229 (2021) - 2020
- [c86]Yossi Arjevani, Ohad Shamir, Nathan Srebro:
A Tight Convergence Analysis for Stochastic Gradient Descent with Delayed Updates. ALT 2020: 111-132 - [c85]Itay Safran, Ohad Shamir:
How Good is SGD with Random Shuffling? COLT 2020: 3250-3284 - [c84]Gilad Yehudai, Ohad Shamir:
Learning a Single Neuron with Gradient Methods. COLT 2020: 3756-3786 - [c83]Yoel Drori, Ohad Shamir:
The Complexity of Finding Stationary Points with Stochastic Gradient Descent. ICML 2020: 2658-2667 - [c82]Eran Malach, Gilad Yehudai, Shai Shalev-Shwartz, Ohad Shamir:
Proving the Lottery Ticket Hypothesis: Pruning is All You Need. ICML 2020: 6682-6691 - [c81]Blake E. Woodworth, Kumar Kshitij Patel, Sebastian U. Stich, Zhen Dai, Brian Bullins, H. Brendan McMahan, Ohad Shamir, Nathan Srebro:
Is Local SGD Better than Minibatch SGD? ICML 2020: 10334-10343 - [c80]Gal Vardi, Ohad Shamir:
Neural Networks with Small Weights and Depth-Separation Barriers. NeurIPS 2020 - [i76]Gilad Yehudai, Ohad Shamir:
Learning a Single Neuron with Gradient Methods. CoRR abs/2001.05205 (2020) - [i75]Eran Malach, Gilad Yehudai, Shai Shalev-Shwartz, Ohad Shamir:
Proving the Lottery Ticket Hypothesis: Pruning is All You Need. CoRR abs/2002.00585 (2020) - [i74]Blake E. Woodworth, Kumar Kshitij Patel
, Sebastian U. Stich, Zhen Dai, Brian Bullins, H. Brendan McMahan, Ohad Shamir, Nathan Srebro:
Is Local SGD Better than Minibatch SGD? CoRR abs/2002.07839 (2020) - [i73]Ohad Shamir:
Can We Find Near-Approximately-Stationary Points of Nonsmooth Nonconvex Functions? CoRR abs/2002.11962 (2020) - [i72]Gal Vardi, Ohad Shamir:
Neural Networks with Small Weights and Depth-Separation Barriers. CoRR abs/2006.00625 (2020) - [i71]Itay Safran, Gilad Yehudai, Ohad Shamir:
The Effects of Mild Over-parameterization on the Optimization Landscape of Shallow ReLU Neural Networks. CoRR abs/2006.01005 (2020) - [i70]Ohad Shamir:
Gradient Methods Never Overfit On Separable Data. CoRR abs/2007.00028 (2020) - [i69]Guy Kornowski, Ohad Shamir:
High-Order Oracle Complexity of Smooth and Strongly Convex Optimization. CoRR abs/2010.06642 (2020) - [i68]Gal Vardi, Ohad Shamir:
Implicit Regularization in ReLU Networks with the Square Loss. CoRR abs/2012.05156 (2020) - [i67]Gal Vardi, Ohad Shamir:
Neural Networks with Small Weights and Depth-Separation Barriers. Electron. Colloquium Comput. Complex. TR20 (2020)
2010 – 2019
- 2019
- [j16]Yossi Arjevani, Ohad Shamir, Ron Shiff
:
Oracle complexity of second-order methods for smooth convex optimization. Math. Program. 178(1-2): 327-360 (2019) - [c79]Yuval Dagan, Gil Kur, Ohad Shamir:
Space lower bounds for linear prediction in the streaming model. COLT 2019: 929-954 - [c78]Dylan J. Foster, Ayush Sekhari, Ohad Shamir, Nathan Srebro, Karthik Sridharan, Blake E. Woodworth:
The Complexity of Making the Gradient Small in Stochastic Convex Optimization. COLT 2019: 1319-1345 - [c77]Itay Safran, Ronen Eldan, Ohad Shamir:
Depth Separations in Neural Networks: What is Actually Being Separated? COLT 2019: 2664-2666 - [c76]Ohad Shamir:
Exponential Convergence Time of Gradient Descent for One-Dimensional Deep Linear Neural Networks. COLT 2019: 2691-2713 - [c75]Gilad Yehudai, Ohad Shamir:
On the Power and Limitations of Random Features for Understanding Neural Networks. NeurIPS 2019: 6594-6604 - [i66]Yuval Dagan, Gil Kur, Ohad Shamir:
Space lower bounds for linear prediction. CoRR abs/1902.03498 (2019) - [i65]Dylan J. Foster, Ayush Sekhari, Ohad Shamir, Nathan Srebro, Karthik Sridharan, Blake E. Woodworth:
The Complexity of Making the Gradient Small in Stochastic Convex Optimization. CoRR abs/1902.04686 (2019) - [i64]Gilad Yehudai, Ohad Shamir:
On the Power and Limitations of Random Features for Understanding Neural Networks. CoRR abs/1904.00687 (2019) - [i63]Itay Safran, Ronen Eldan, Ohad Shamir:
Depth Separations in Neural Networks: What is Actually Being Separated? CoRR abs/1904.06984 (2019) - [i62]Itay Safran, Ohad Shamir:
How Good is SGD with Random Shuffling? CoRR abs/1908.00045 (2019) - [i61]Yoel Drori, Ohad Shamir:
The Complexity of Finding Stationary Points with Stochastic Gradient Descent. CoRR abs/1910.01845 (2019) - 2018
- [j15]Ohad Shamir:
Distribution-Specific Hardness of Learning Neural Networks. J. Mach. Learn. Res. 19: 32:1-32:29 (2018) - [c74]Nicolò Cesa-Bianchi, Ohad Shamir:
Bandit Regret Scaling with the Effective Loss Range. ALT 2018: 128-151 - [c73]Noah Golowich, Alexander Rakhlin, Ohad Shamir:
Size-Independent Sample Complexity of Neural Networks. COLT 2018: 297-299 - [c72]Yuval Dagan, Ohad Shamir:
Detecting Correlations with Little Memory and Communication. COLT 2018: 1145-1198 - [c71]Itay Safran, Ohad Shamir:
Spurious Local Minima are Common in Two-Layer ReLU Neural Networks. ICML 2018: 4430-4438 - [c70]Ohad Shamir:
Are ResNets Provably Better than Linear Predictors? NeurIPS 2018: 505-514 - [c69]Murat A. Erdogdu, Lester Mackey, Ohad Shamir:
Global Non-convex Optimization with Discretized Diffusions. NeurIPS 2018: 9694-9703 - [i60]Yuval Dagan, Ohad Shamir:
Detecting Correlations with Little Memory and Communication. CoRR abs/1803.01420 (2018) - [i59]Ohad Shamir:
Are ResNets Provably Better than Linear Predictors? CoRR abs/1804.06739 (2018) - [i58]Yossi Arjevani, Ohad Shamir, Nathan Srebro:
A Tight Convergence Analysis for Stochastic Gradient Descent with Delayed Updates. CoRR abs/1806.10188 (2018) - [i57]Ohad Shamir:
Exponential Convergence Time of Gradient Descent for One-Dimensional Deep Linear Neural Networks. CoRR abs/1809.08587 (2018) - [i56]Murat A. Erdogdu, Lester Mackey, Ohad Shamir:
Global Non-convex Optimization with Discretized Diffusions. CoRR abs/1810.12361 (2018) - 2017
- [j14]Ohad Shamir:
An Optimal Algorithm for Bandit and Zero-Order Convex Optimization with Two-Point Feedback. J. Mach. Learn. Res. 18: 52:1-52:11 (2017) - [j13]Noga Alon, Nicolò Cesa-Bianchi, Claudio Gentile, Shie Mannor
, Yishay Mansour, Ohad Shamir:
Nonstochastic Multi-Armed Bandits with Graph-Structured Feedback. SIAM J. Comput. 46(6): 1785-1826 (2017) - [c68]Satyen Kale, Ohad Shamir:
Preface: Conference on Learning Theory (COLT), 2017. COLT 2017: 1-3 - [c67]Yossi Arjevani, Ohad Shamir:
Oracle Complexity of Second-Order Methods for Finite-Sum Problems. ICML 2017: 205-213 - [c66]Dan Garber, Ohad Shamir, Nathan Srebro:
Communication-efficient Algorithms for Distributed Stochastic Principal Component Analysis. ICML 2017: 1203-1212 - [c65]Itay Safran, Ohad Shamir:
Depth-Width Tradeoffs in Approximating Natural Functions with Neural Networks. ICML 2017: 2979-2987 - [c64]Shai Shalev-Shwartz, Ohad Shamir, Shaked Shammah:
Failures of Gradient-Based Deep Learning. ICML 2017: 3067-3075 - [c63]Ohad Shamir, Liran Szlak:
Online Learning with Local Permutations and Delayed Feedback. ICML 2017: 3086-3094 - [e2]Satyen Kale, Ohad Shamir:
Proceedings of the 30th Conference on Learning Theory, COLT 2017, Amsterdam, The Netherlands, 7-10 July 2017. Proceedings of Machine Learning Research 65, PMLR 2017 [contents] - [i55]Dan Garber, Ohad Shamir, Nathan Srebro:
Communication-efficient Algorithms for Distributed Stochastic Principal Component Analysis. CoRR abs/1702.08169 (2017) - [i54]Ohad Shamir, Liran Szlak:
Online Learning with Local Permutations and Delayed Feedback. CoRR abs/1703.04274 (2017) - [i53]Shai Shalev-Shwartz, Ohad Shamir, Shaked Shammah:
Failures of Deep Learning. CoRR abs/1703.07950 (2017) - [i52]Nicolò Cesa-Bianchi, Ohad Shamir:
Bandit Regret Scaling with the Effective Loss Range. CoRR abs/1705.05091 (2017) - [i51]Shai Shalev-Shwartz, Ohad Shamir, Shaked Shammah:
Weight Sharing is Crucial to Succesful Optimization. CoRR abs/1706.00687 (2017) - [i50]Noah Golowich, Alexander Rakhlin, Ohad Shamir:
Size-Independent Sample Complexity of Neural Networks. CoRR abs/1712.06541 (2017) - [i49]Itay Safran, Ohad Shamir:
Spurious Local Minima are Common in Two-Layer ReLU Neural Networks. CoRR abs/1712.08968 (2017) - 2016
- [j12]Yossi Arjevani, Shai Shalev-Shwartz, Ohad Shamir:
On Lower and Upper Bounds in Smooth and Strongly Convex Optimization. J. Mach. Learn. Res. 17: 126:1-126:51 (2016) - [j11]Niv Buchbinder
, Shahar Chen, Joseph Naor, Ohad Shamir:
Unified Algorithms for Online Learning and Competitive Analysis. Math. Oper. Res. 41(2): 612-625 (2016) - [c62]Ronen Eldan, Ohad Shamir:
The Power of Depth for Feedforward Neural Networks. COLT 2016: 907-940 - [c61]Jonathan Rosenski, Ohad Shamir, Liran Szlak:
Multi-Player Bandits - a Musical Chairs Approach. ICML 2016: 155-163 - [c60]Ohad Shamir:
Fast Stochastic Algorithms for SVD and PCA: Convergence Properties and Convexity. ICML 2016: 248-256 - [c59]Ohad Shamir:
Convergence of Stochastic Gradient Descent for PCA. ICML 2016: 257-265 - [c58]Itay Safran, Ohad Shamir:
On the Quality of the Initial Basin in Overspecified Neural Networks. ICML 2016: 774-782 - [c57]Yossi Arjevani, Ohad Shamir:
On the Iteration Complexity of Oblivious First-Order Optimization Algorithms. ICML 2016: 908-916 - [c56]Ohad Shamir:
Without-Replacement Sampling for Stochastic Gradient Methods. NIPS 2016: 46-54 - [c55]Yossi Arjevani, Ohad Shamir:
Dimension-Free Iteration Complexity of Finite Sum Optimization Problems. NIPS 2016: 3540-3548 - [e1]Vitaly Feldman, Alexander Rakhlin, Ohad Shamir:
Proceedings of the 29th Conference on Learning Theory, COLT 2016, New York, USA, June 23-26, 2016. JMLR Workshop and Conference Proceedings 49, JMLR.org 2016 [contents] - [i48]Ohad Shamir:
Without-Replacement Sampling for Stochastic Gradient Methods: Convergence Results and Application to Distributed Optimization. CoRR abs/1603.00570 (2016) - [i47]Yossi Arjevani, Ohad Shamir:
On the Iteration Complexity of Oblivious First-Order Optimization Algorithms. CoRR abs/1605.03529 (2016) - [i46]Yossi Arjevani, Ohad Shamir:
Dimension-Free Iteration Complexity of Finite Sum Optimization Problems. CoRR abs/1606.09333 (2016) - [i45]Ohad Shamir:
Distribution-Specific Hardness of Learning Neural Networks. CoRR abs/1609.01037 (2016) - [i44]Itay Safran, Ohad Shamir:
Depth Separation in ReLU Networks for Approximating Smooth Non-Linear Functions. CoRR abs/1610.09887 (2016) - [i43]Yossi Arjevani, Ohad Shamir:
Oracle Complexity of Second-Order Methods for Finite-Sum Problems. CoRR abs/1611.04982 (2016) - 2015
- [j10]Ohad Shamir:
The sample complexity of learning linear predictors with the squared loss. J. Mach. Learn. Res. 16: 3475-3486 (2015) - [c54]Ethan Fetaya, Ohad Shamir, Shimon Ullman:
Graph Approximation and Clustering on a Budget. AISTATS 2015 - [c53]Nicolò Cesa-Bianchi, Yishay Mansour, Ohad Shamir:
On the Complexity of Learning with Kernels. COLT 2015: 297-325 - [c52]Ohad Shamir:
On the Complexity of Bandit Linear Optimization. COLT 2015: 1523-1551 - [c51]Ohad Shamir:
A Stochastic PCA and SVD Algorithm with an Exponential Convergence Rate. ICML 2015: 144-152 - [c50]Doron Kukliansky, Ohad Shamir:
Attribute Efficient Linear Regression with Distribution-Dependent Sampling. ICML 2015: 153-161 - [c49]Yossi Arjevani, Ohad Shamir:
Communication Complexity of Distributed Convex Learning and Optimization. NIPS 2015: 1756-1764 - [i42]Yossi Arjevani, Shai Shalev-Shwartz, Ohad Shamir:
On Lower and Upper Bounds for Smooth and Strongly Convex Optimization Problems. CoRR abs/1503.06833 (2015) - [i41]Yossi Arjevani, Ohad Shamir:
Communication Complexity of Distributed Convex Learning and Optimization. CoRR abs/1506.01900 (2015) - [i40]Ohad Shamir:
An Optimal Algorithm for Bandit and Zero-Order Convex Optimization with Two-Point Feedback. CoRR abs/1507.08752 (2015) - [i39]Ohad Shamir:
Fast Stochastic Algorithms for SVD and PCA: Convergence Properties and Convexity. CoRR abs/1507.08788 (2015) - [i38]Ohad Shamir:
Convergence of Stochastic Gradient Descent for PCA. CoRR abs/1509.09002 (2015) - [i37]Itay Safran, Ohad Shamir:
On the Quality of the Initial Basin in Overspecified Neural Networks. CoRR abs/1511.04210 (2015) - [i36]Jonathan Rosenski, Ohad Shamir, Liran Szlak:
Multi-Player Bandits - a Musical Chairs Approach. CoRR abs/1512.02866 (2015) - [i35]Ronen Eldan, Ohad Shamir:
The Power of Depth for Feedforward Neural Networks. CoRR abs/1512.03965 (2015) - 2014
- [j9]Ohad Shamir, Shai Shalev-Shwartz:
Matrix completion with the trace norm: learning, bounding, and transducing. J. Mach. Learn. Res. 15(1): 3401-3423 (2014) - [c48]Ohad Shamir, Nathan Srebro:
Distributed stochastic optimization and learning. Allerton 2014: 850-857 - [c47]Ishai Menache, Ohad Shamir, Navendu Jain:
On-demand, Spot, or Both: Dynamic Resource Allocation for Executing Batch Jobs in the Cloud. ICAC 2014: 177-187 - [c46]Ohad Shamir, Nathan Srebro, Tong Zhang:
Communication-Efficient Distributed Optimization using an Approximate Newton-type Method. ICML 2014: 1000-1008 - [c45]Ohad Shamir:
Fundamental Limits of Online and Distributed Algorithms for Statistical Learning and Estimation. NIPS 2014: 163-171 - [c44]Roi Livni, Shai Shalev-Shwartz, Ohad Shamir:
On the Computational Efficiency of Training Neural Networks. NIPS 2014: 855-863 - [i34]Ethan Fetaya, Ohad Shamir, Shimon Ullman:
Graph Approximation and Clustering on a Budget. CoRR abs/1406.2602 (2014) - [i33]Ohad Shamir:
The Sample Complexity of Learning Linear Predictors with the Squared Loss. CoRR abs/1406.5143 (2014) - [i32]Ohad Shamir:
On the Complexity of Bandit Linear Optimization. CoRR abs/1408.2368 (2014) - [i31]Ohad Shamir:
A Stochastic PCA Algorithm with an Exponential Convergence Rate. CoRR abs/1409.2848 (2014) - [i30]Noga Alon, Nicolò Cesa-Bianchi, Claudio Gentile, Shie Mannor, Yishay Mansour, Ohad Shamir:
Nonstochastic Multi-Armed Bandits with Graph-Structured Feedback. CoRR abs/1409.8428 (2014) - [i29]Roi Livni, Shai Shalev-Shwartz, Ohad Shamir:
On the Computational Efficiency of Training Neural Networks. CoRR abs/1410.1141 (2014) - [i28]Doron Kukliansky, Ohad Shamir:
Attribute Efficient Linear Regression with Data-Dependent Sampling. CoRR abs/1410.6382 (2014) - [i27]Nicolò Cesa-Bianchi, Yishay Mansour, Ohad Shamir:
On the Complexity of Learning with Kernels. CoRR abs/1411.1158 (2014) - 2013
- [c43]Alexander Rakhlin, Ohad Shamir, Karthik Sridharan:
Localization and Adaptation in Online Learning. AISTATS 2013: 516-526 - [c42]Nicolò Cesa-Bianchi, Ohad Shamir:
Efficient Transductive Online Learning via Randomized Rounding. Empirical Inference 2013: 177-194 - [c41]Ohad Shamir:
On the Complexity of Bandit and Derivative-Free Stochastic Convex Optimization. COLT 2013: 3-24 - [c40]Oren Anava, Elad Hazan, Shie Mannor, Ohad Shamir:
Online Learning for Time Series Prediction. COLT 2013: 172-184 - [c39]Baoyuan Liu, Fereshteh Sadeghi, Marshall F. Tappen, Ohad Shamir, Ce Liu:
Probabilistic Label Trees for Efficient Large Scale Image Classification. CVPR 2013: 843-850 - [c38]Ohad Shamir, Tong Zhang:
Stochastic Gradient Descent for Non-smooth Optimization: Convergence Results and Optimal Averaging Schemes. ICML (1) 2013: 71-79 - [c37]Nicolò Cesa-Bianchi, Ofer Dekel, Ohad Shamir:
Online Learning with Switching Costs and Other Adaptive Adversaries. NIPS 2013: 1160-1168 - [c36]Or Zuk, Amnon Amir, Amit Zeisel, Ohad Shamir, Noam Shental:
Accurate Profiling of Microbial Communities from Massively Parallel Sequencing Using Convex Optimization. SPIRE 2013: 279-297 - [i26]Nicolò Cesa-Bianchi, Ofer Dekel, Ohad Shamir:
Online Learning with Switching Costs and Other Adaptive Adversaries. CoRR abs/1302.4387 (2013) - [i25]Oren Anava, Elad Hazan, Shie Mannor, Ohad Shamir:
Online Learning for Time Series Prediction. CoRR abs/1302.6927 (2013) - [i24]Roi Livni, Shai Shalev-Shwartz, Ohad Shamir:
A Provably Efficient Algorithm for Training Deep Networks. CoRR abs/1304.7045 (2013) - [i23]Or Zuk, Amnon Amir, Amit Zeisel, Ohad Shamir, Noam Shental:
Accurate Profiling of Microbial Communities from Massively Parallel Sequencing using Convex Optimization. CoRR abs/1309.6919 (2013) - [i22]Ohad Shamir:
Fundamental Limits of Online and Distributed Algorithms for Statistical Learning and Estimation. CoRR abs/1311.3494 (2013) - [i21]Ohad Shamir, Nathan Srebro, Tong Zhang:
Communication Efficient Distributed Optimization using an Approximate Newton-type Method. CoRR abs/1312.7853 (2013) - 2012
- [j8]Ofer Dekel, Ran Gilad-Bachrach, Ohad Shamir, Lin Xiao:
Optimal Distributed Online Prediction Using Mini-Batches. J. Mach. Learn. Res. 13: 165-202 (2012) - [c35]Orly Avner, Shie Mannor, Ohad Shamir:
Decoupling Exploration and Exploitation in Multi-Armed Bandits. ICML 2012 - [c34]Alexander Rakhlin, Ohad Shamir, Karthik Sridharan:
Making Gradient Descent Optimal for Strongly Convex Stochastic Optimization. ICML 2012 - [c33]Alexander Rakhlin, Ohad Shamir, Karthik Sridharan:
Relax and Randomize : From Value to Algorithms. NIPS 2012: 2150-2158 - [c32]Niv Buchbinder, Shahar Chen, Joseph Naor, Ohad Shamir:
Unified Algorithms for Online Learning and Competitive Analysis. COLT 2012: 5.1-5.18 - [c31]Ohad Shamir:
Open Problem: Is Averaging Needed for Strongly Convex Stochastic Gradient Descent? COLT 2012: 47.1-47.3 - [c30]Ofer Dekel, Ohad Shamir:
There's a Hole in My Data Space: Piecewise Predictors for Heterogeneous Learning Problems. AISTATS 2012: 291-298 - [c29]Shai Shalev-Shwartz, Ohad Shamir, Eran Tromer:
Using More Data to Speed-up Training Time. AISTATS 2012: 1019-1027 - [c28]Ruth Urner, Shai Ben-David, Ohad Shamir:
Learning from Weak Teachers. AISTATS 2012: 1252-1260 - [i20]Alexander Rakhlin, Ohad Shamir, Karthik Sridharan:
Relax and Localize: From Value to Algorithms. CoRR abs/1204.0870 (2012) - [i19]Orly Avner, Shie Mannor, Ohad Shamir:
Decoupling Exploration and Exploitation in Multi-Armed Bandits. CoRR abs/1205.2874 (2012) - [i18]Ohad Shamir:
On the Complexity of Bandit and Derivative-Free Stochastic Convex Optimization. CoRR abs/1209.2388 (2012) - [i17]Ohad Shamir, Tong Zhang:
Stochastic Gradient Descent for Non-smooth Optimization: Convergence Results and Optimal Averaging Schemes. CoRR abs/1212.1824 (2012) - 2011
- [j7]Nicolò Cesa-Bianchi, Shai Shalev-Shwartz, Ohad Shamir:
Efficient Learning with Partially Observed Attributes. J. Mach. Learn. Res. 12: 2857-2878 (2011) - [j6]Shai Shalev-Shwartz, Ohad Shamir, Karthik Sridharan:
Learning Kernel-Based Halfspaces with the 0-1 Loss. SIAM J. Comput. 40(6): 1623-1646 (2011) - [j5]Nicolò Cesa-Bianchi, Shai Shalev-Shwartz, Ohad Shamir:
Online Learning of Noisy Data. IEEE Trans. Inf. Theory 57(12): 7907-7931 (2011) - [c27]Nicolò Cesa-Bianchi, Shai Shalev-Shwartz, Ohad Shamir:
Quantity Makes Quality: Learning with Partial Views. AAAI 2011: 1547-1550 - [c26]Shai Shalev-Shwartz, Alon Gonen, Ohad Shamir:
Large-Scale Convex Minimization with a Low-Rank Constraint. ICML 2011: 329-336 - [c25]Omer Tamuz, Ce Liu, Serge J. Belongie, Ohad Shamir, Adam Kalai:
Adaptively Learning the Crowd Kernel. ICML 2011: 673-680 - [c24]Ofer Dekel, Ran Gilad-Bachrach, Ohad Shamir, Lin Xiao:
Optimal Distributed Online Prediction. ICML 2011: 713-720 - [c23]Shai Shalev-Shwartz, Ohad Shamir, Karthik Sridharan:
Learning Linear and Kernel Predictors with the 0-1 Loss Function. IJCAI 2011: 2740-2745 - [c22]Nicolò Cesa-Bianchi, Ohad Shamir:
Efficient Online Learning via Randomized Rounding. NIPS 2011: 343-351 - [c21]Shie Mannor, Ohad Shamir:
From Bandits to Experts: On the Value of Side-Observations. NIPS 2011: 684-692 - [c20]Sham M. Kakade, Adam Kalai, Varun Kanade, Ohad Shamir:
Efficient Learning of Generalized Linear and Single Index Models with Isotonic Regression. NIPS 2011: 927-935 - [c19]Andrew Cotter, Ohad Shamir, Nati Srebro, Karthik Sridharan:
Better Mini-Batch Algorithms via Accelerated Gradient Methods. NIPS 2011: 1647-1655 - [c18]Rina Foygel, Ruslan Salakhutdinov, Ohad Shamir, Nati Srebro:
Learning with the weighted trace-norm under arbitrary sampling distributions. NIPS 2011: 2133-2141 - [c17]Ohad Shamir, Shai Shalev-Shwartz:
Collaborative Filtering with the Trace Norm: Learning, Bounding, and Transducing. COLT 2011: 661-678 - [c16]Ohad Shamir, Naftali Tishby:
Spectral Clustering on a Budget. AISTATS 2011: 661-669 - [i16]Sham M. Kakade, Adam Tauman Kalai, Varun Kanade, Ohad Shamir:
Efficient Learning of Generalized Linear and Single Index Models with Isotonic Regression. CoRR abs/1104.2018 (2011) - [i15]Omer Tamuz, Ce Liu, Serge J. Belongie, Ohad Shamir, Adam Tauman Kalai:
Adaptively Learning the Crowd Kernel. CoRR abs/1105.1033 (2011) - [i14]Shai Shalev-Shwartz, Ohad Shamir, Eran Tromer:
Using More Data to Speed-up Training Time. CoRR abs/1106.1216 (2011) - [i13]Shai Shalev-Shwartz, Alon Gonen, Ohad Shamir:
Large-Scale Convex Minimization with a Low-Rank Constraint. CoRR abs/1106.1622 (2011) - [i12]Nicolò Cesa-Bianchi, Ohad Shamir:
Efficient Online Learning via Randomized Rounding. CoRR abs/1106.2429 (2011) - [i11]Shie Mannor, Ohad Shamir:
From Bandits to Experts: On the Value of Side-Observations. CoRR abs/1106.2436 (2011) - [i10]Rina Foygel, Ruslan Salakhutdinov, Ohad Shamir, Nathan Srebro:
Learning with the Weighted Trace-norm under Arbitrary Sampling Distributions. CoRR abs/1106.4251 (2011) - [i9]Andrew Cotter, Ohad Shamir, Nathan Srebro, Karthik Sridharan:
Better Mini-Batch Algorithms via Accelerated Gradient Methods. CoRR abs/1106.4574 (2011) - [i8]Ohad Shamir:
Making Gradient Descent Optimal for Strongly Convex Stochastic Optimization. CoRR abs/1109.5647 (2011) - [i7]Ohad Shamir:
A Variant of Azuma's Inequality for Martingales with Subgaussian Tails. CoRR abs/1110.2392 (2011) - 2010
- [b1]Ohad Shamir:
On stability in statistical machine learning (עם תקציר בעברית ושער נוסף: על יציבות בלמידה חישובית סטטיסטית.). Hebrew University of Jerusalem, Israel, 2010 - [j4]Shai Shalev-Shwartz, Ohad Shamir, Nathan Srebro, Karthik Sridharan:
Learnability, Stability and Uniform Convergence. J. Mach. Learn. Res. 11: 2635-2670 (2010) - [j3]Ohad Shamir, Naftali Tishby:
Stability and model selection in k-means clustering. Mach. Learn. 80(2-3): 213-243 (2010) - [j2]Ofer Dekel, Ohad Shamir, Lin Xiao:
Learning to classify with missing and corrupted features. Mach. Learn. 81(2): 149-178 (2010) - [j1]Ohad Shamir, Sivan Sabato
, Naftali Tishby:
Learning and generalization with the information bottleneck. Theor. Comput. Sci. 411(29-30): 2696-2711 (2010) - [c15]Nicolò Cesa-Bianchi, Shai Shalev-Shwartz, Ohad Shamir:
Online Learning of Noisy Data with Kernels. COLT 2010: 218-230 - [c14]Shai Shalev-Shwartz, Ohad Shamir, Karthik Sridharan:
Learning Kernel-Based Halfspaces with the Zero-One Loss. COLT 2010: 441-450 - [c13]Nicolò Cesa-Bianchi, Shai Shalev-Shwartz, Ohad Shamir:
Efficient Learning with Partially Observed Attributes. ICML 2010: 183-190 - [c12]Ofer Dekel, Ohad Shamir:
Multiclass-Multilabel Classification with More Classes than Examples. AISTATS 2010: 137-144 - [c11]Sham M. Kakade, Ohad Shamir, Karthik Sindharan, Ambuj Tewari:
Learning Exponential Families in High-Dimensions: Strong Convexity and Sparsity. AISTATS 2010: 381-388 - [i6]Nicolò Cesa-Bianchi, Shai Shalev-Shwartz, Ohad Shamir:
Efficient Learning with Partially Observed Attributes. CoRR abs/1004.4421 (2010) - [i5]Nicolò Cesa-Bianchi, Shai Shalev-Shwartz, Ohad Shamir:
Online Learning of Noisy Data with Kernels. CoRR abs/1005.2296 (2010) - [i4]Shai Shalev-Shwartz, Ohad Shamir, Karthik Sridharan:
Learning Kernel-Based Halfspaces with the Zero-One Loss. CoRR abs/1005.3681 (2010) - [i3]Ofer Dekel, Ran Gilad-Bachrach, Ohad Shamir, Lin Xiao:
Optimal Distributed Online Prediction using Mini-Batches. CoRR abs/1012.1367 (2010) - [i2]Ofer Dekel, Ran Gilad-Bachrach, Ohad Shamir, Lin Xiao:
Robust Distributed Online Prediction. CoRR abs/1012.1370 (2010)
2000 – 2009
- 2009
- [c10]Ofer Dekel, Ohad Shamir:
Vox Populi: Collecting High-Quality Labels from a Crowd. COLT 2009 - [c9]Shai Shalev-Shwartz, Ohad Shamir, Karthik Sridharan:
The Complexity of Improperly Learning Large Margin Halfspaces. COLT 2009 - [c8]Shai Shalev-Shwartz, Ohad Shamir, Nathan Srebro, Karthik Sridharan:
Stochastic Convex Optimization. COLT 2009 - [c7]Shai Shalev-Shwartz, Ohad Shamir, Nathan Srebro, Karthik Sridharan:
Learnability and Stability in the General Learning Setting. COLT 2009 - [c6]Ofer Dekel, Ohad Shamir:
Good learners for evil teachers. ICML 2009: 233-240 - [i1]Sham M. Kakade, Ohad Shamir, Karthik Sridharan, Ambuj Tewari:
Learning Exponential Families in High-Dimensions: Strong Convexity and Sparsity. CoRR abs/0911.0054 (2009) - 2008
- [c5]Ohad Shamir, Sivan Sabato
, Naftali Tishby:
Learning and Generalization with the Information Bottleneck. ALT 2008: 92-107 - [c4]Ohad Shamir, Naftali Tishby:
Model Selection and Stability in k-means Clustering. COLT 2008: 367-378 - [c3]Ofer Dekel, Ohad Shamir:
Learning to classify with missing and corrupted features. ICML 2008: 216-223 - [c2]Ohad Shamir, Naftali Tishby:
On the Reliability of Clustering Stability in the Large Sample Regime. NIPS 2008: 1465-1472 - 2007
- [c1]Ohad Shamir, Naftali Tishby:
Cluster Stability for Finite Samples. NIPS 2007: 1297-1304
Coauthor Index

manage site settings
To protect your privacy, all features that rely on external API calls from your browser are turned off by default. You need to opt-in for them to become active. All settings here will be stored as cookies with your web browser. For more information see our F.A.Q.
Unpaywalled article links
Add open access links from to the list of external document links (if available).
Privacy notice: By enabling the option above, your browser will contact the API of unpaywall.org to load hyperlinks to open access articles. Although we do not have any reason to believe that your call will be tracked, we do not have any control over how the remote server uses your data. So please proceed with care and consider checking the Unpaywall privacy policy.
Archived links via Wayback Machine
For web page which are no longer available, try to retrieve content from the of the Internet Archive (if available).
Privacy notice: By enabling the option above, your browser will contact the API of archive.org to check for archived content of web pages that are no longer available. Although we do not have any reason to believe that your call will be tracked, we do not have any control over how the remote server uses your data. So please proceed with care and consider checking the Internet Archive privacy policy.
Reference lists
Add a list of references from ,
, and
to record detail pages.
load references from crossref.org and opencitations.net
Privacy notice: By enabling the option above, your browser will contact the APIs of crossref.org, opencitations.net, and semanticscholar.org to load article reference information. Although we do not have any reason to believe that your call will be tracked, we do not have any control over how the remote server uses your data. So please proceed with care and consider checking the Crossref privacy policy and the OpenCitations privacy policy, as well as the AI2 Privacy Policy covering Semantic Scholar.
Citation data
Add a list of citing articles from and
to record detail pages.
load citations from opencitations.net
Privacy notice: By enabling the option above, your browser will contact the API of opencitations.net and semanticscholar.org to load citation information. Although we do not have any reason to believe that your call will be tracked, we do not have any control over how the remote server uses your data. So please proceed with care and consider checking the OpenCitations privacy policy as well as the AI2 Privacy Policy covering Semantic Scholar.
OpenAlex data
Load additional information about publications from .
Privacy notice: By enabling the option above, your browser will contact the API of openalex.org to load additional information. Although we do not have any reason to believe that your call will be tracked, we do not have any control over how the remote server uses your data. So please proceed with care and consider checking the information given by OpenAlex.
last updated on 2024-05-08 23:32 CEST by the dblp team
all metadata released as open data under CC0 1.0 license
see also: Terms of Use | Privacy Policy | Imprint