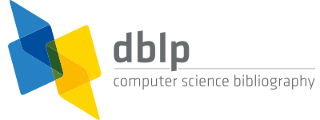


default search action
Philip M. Long
Person information
- affiliation: Google, Mountain View, CA, USA
Refine list

refinements active!
zoomed in on ?? of ?? records
view refined list in
export refined list as
2020 – today
- 2024
- [j64]Peter L. Bartlett, Philip M. Long:
Corrigendum to "Prediction, learning, uniform convergence, and scale-sensitive dimensions" [J. Comput. Syst. Sci. 56 (2) (1998) 174-190]. J. Comput. Syst. Sci. 140: 103465 (2024) - [j63]Philip M. Long, Peter L. Bartlett:
Sharpness-Aware Minimization and the Edge of Stability. J. Mach. Learn. Res. 25: 179:1-179:20 (2024) - 2023
- [j62]Niladri S. Chatterji, Philip M. Long:
Deep linear networks can benignly overfit when shallow ones do. J. Mach. Learn. Res. 24: 117:1-117:39 (2023) - [j61]Peter L. Bartlett, Philip M. Long, Olivier Bousquet:
The Dynamics of Sharpness-Aware Minimization: Bouncing Across Ravines and Drifting Towards Wide Minima. J. Mach. Learn. Res. 24: 316:1-316:36 (2023) - [i30]Peter L. Bartlett, Philip M. Long:
Prediction, Learning, Uniform Convergence, and Scale-sensitive Dimensions. CoRR abs/2304.11059 (2023) - [i29]Philip M. Long, Peter L. Bartlett:
Sharpness-Aware Minimization and the Edge of Stability. CoRR abs/2309.12488 (2023) - 2022
- [j60]Niladri S. Chatterji, Philip M. Long:
Foolish Crowds Support Benign Overfitting. J. Mach. Learn. Res. 23: 125:1-125:12 (2022) - [j59]Niladri S. Chatterji, Philip M. Long, Peter L. Bartlett:
The Interplay Between Implicit Bias and Benign Overfitting in Two-Layer Linear Networks. J. Mach. Learn. Res. 23: 263:1-263:48 (2022) - [j58]Philip M. Long, Rocco A. Servedio
:
The Perils of Being Unhinged: On the Accuracy of Classifiers Minimizing a Noise-Robust Convex Loss. Neural Comput. 34(6): 1488-1499 (2022) - [i28]Niladri S. Chatterji, Philip M. Long:
Deep Linear Networks can Benignly Overfit when Shallow Ones Do. CoRR abs/2209.09315 (2022) - [i27]Peter L. Bartlett, Philip M. Long, Olivier Bousquet:
The Dynamics of Sharpness-Aware Minimization: Bouncing Across Ravines and Drifting Towards Wide Minima. CoRR abs/2210.01513 (2022) - 2021
- [j57]Niladri S. Chatterji, Philip M. Long:
Finite-sample Analysis of Interpolating Linear Classifiers in the Overparameterized Regime. J. Mach. Learn. Res. 22: 129:1-129:30 (2021) - [j56]Niladri S. Chatterji, Philip M. Long, Peter L. Bartlett:
When Does Gradient Descent with Logistic Loss Find Interpolating Two-Layer Networks? J. Mach. Learn. Res. 22: 159:1-159:48 (2021) - [j55]Peter L. Bartlett, Philip M. Long:
Failures of Model-dependent Generalization Bounds for Least-norm Interpolation. J. Mach. Learn. Res. 22: 204:1-204:15 (2021) - [j54]Philip M. Long:
Superlinear Integrality Gaps for the Minimum Majority Problem. SIAM J. Discret. Math. 35(4): 3004-3016 (2021) - [c72]Niladri S. Chatterji, Philip M. Long, Peter L. Bartlett:
When does gradient descent with logistic loss interpolate using deep networks with smoothed ReLU activations? COLT 2021: 927-1027 - [i26]Niladri S. Chatterji, Philip M. Long, Peter L. Bartlett:
When does gradient descent with logistic loss interpolate using deep networks with smoothed ReLU activations? CoRR abs/2102.04998 (2021) - [i25]Philip M. Long:
Properties of the After Kernel. CoRR abs/2105.10585 (2021) - [i24]Niladri S. Chatterji, Philip M. Long, Peter L. Bartlett:
The Interplay Between Implicit Bias and Benign Overfitting in Two-Layer Linear Networks. CoRR abs/2108.11489 (2021) - [i23]Niladri S. Chatterji, Philip M. Long:
Foolish Crowds Support Benign Overfitting. CoRR abs/2110.02914 (2021) - [i22]Philip M. Long, Rocco A. Servedio:
The perils of being unhinged: On the accuracy of classifiers minimizing a noise-robust convex loss. CoRR abs/2112.04590 (2021) - 2020
- [j53]Philip M. Long:
New bounds on the price of bandit feedback for mistake-bounded online multiclass learning. Theor. Comput. Sci. 808: 159-163 (2020) - [c71]Philip M. Long, Raphael J. Long:
On the Complexity of Proper Distribution-Free Learning of Linear Classifiers. ALT 2020: 583-591 - [c70]Philip M. Long, Hanie Sedghi:
Generalization bounds for deep convolutional neural networks. ICLR 2020 - [c69]Difan Zou, Philip M. Long, Quanquan Gu:
On the Global Convergence of Training Deep Linear ResNets. ICLR 2020 - [i21]Niladri S. Chatterji, Peter L. Bartlett, Philip M. Long:
Oracle lower bounds for stochastic gradient sampling algorithms. CoRR abs/2002.00291 (2020) - [i20]Difan Zou, Philip M. Long, Quanquan Gu:
On the Global Convergence of Training Deep Linear ResNets. CoRR abs/2003.01094 (2020) - [i19]Niladri S. Chatterji, Philip M. Long:
Finite-sample analysis of interpolating linear classifiers in the overparameterized regime. CoRR abs/2004.12019 (2020) - [i18]Peter L. Bartlett, Philip M. Long:
Failures of model-dependent generalization bounds for least-norm interpolation. CoRR abs/2010.08479 (2020) - [i17]Niladri S. Chatterji, Philip M. Long, Peter L. Bartlett:
When does gradient descent with logistic loss find interpolating two-layer networks? CoRR abs/2012.02409 (2020)
2010 – 2019
- 2019
- [j52]Peter L. Bartlett, David P. Helmbold, Philip M. Long:
Gradient Descent with Identity Initialization Efficiently Learns Positive-Definite Linear Transformations by Deep Residual Networks. Neural Comput. 31(3) (2019) - [j51]Philip M. Long, Hanie Sedghi:
On the Effect of the Activation Function on the Distribution of Hidden Nodes in a Deep Network. Neural Comput. 31(12): 2562-2580 (2019) - [c68]Hanie Sedghi, Vineet Gupta, Philip M. Long:
The Singular Values of Convolutional Layers. ICLR (Poster) 2019 - [c67]Anindya De, Philip M. Long, Rocco A. Servedio
:
Density Estimation for Shift-Invariant Multidimensional Distributions. ITCS 2019: 28:1-28:20 - [i16]Philip M. Long, Hanie Sedghi:
On the effect of the activation function on the distribution of hidden nodes in a deep network. CoRR abs/1901.02104 (2019) - [i15]Philip M. Long, Hanie Sedghi:
Size-free generalization bounds for convolutional neural networks. CoRR abs/1905.12600 (2019) - [i14]Peter L. Bartlett, Philip M. Long, Gábor Lugosi, Alexander Tsigler:
Benign Overfitting in Linear Regression. CoRR abs/1906.11300 (2019) - 2018
- [c66]Anindya De, Philip M. Long, Rocco A. Servedio
:
Learning Sums of Independent Random Variables with Sparse Collective Support. FOCS 2018: 297-308 - [c65]Peter L. Bartlett, David P. Helmbold, Philip M. Long:
Gradient descent with identity initialization efficiently learns positive definite linear transformations. ICML 2018: 520-529 - [i13]Peter L. Bartlett, David P. Helmbold, Philip M. Long:
Gradient descent with identity initialization efficiently learns positive definite linear transformations by deep residual networks. CoRR abs/1802.06093 (2018) - [i12]Peter L. Bartlett, Steven N. Evans, Philip M. Long:
Representing smooth functions as compositions of near-identity functions with implications for deep network optimization. CoRR abs/1804.05012 (2018) - [i11]Hanie Sedghi, Vineet Gupta, Philip M. Long:
The Singular Values of Convolutional Layers. CoRR abs/1805.10408 (2018) - [i10]Anindya De, Philip M. Long, Rocco A. Servedio:
Learning Sums of Independent Random Variables with Sparse Collective Support. CoRR abs/1807.07013 (2018) - [i9]Anindya De, Philip M. Long, Rocco A. Servedio:
Density estimation for shift-invariant multidimensional distributions. CoRR abs/1811.03744 (2018) - 2017
- [j50]Pranjal Awasthi, Maria-Florina Balcan, Philip M. Long:
The Power of Localization for Efficiently Learning Linear Separators with Noise. J. ACM 63(6): 50:1-50:27 (2017) - [j49]David P. Helmbold, Philip M. Long:
Surprising properties of dropout in deep networks. J. Mach. Learn. Res. 18: 200:1-200:28 (2017) - [c64]Philip M. Long:
New bounds on the price of bandit feedback for mistake-bounded online multiclass learning. ALT 2017: 3-10 - [c63]David P. Helmbold, Philip M. Long:
Surprising properties of dropout in deep networks. COLT 2017: 1123-1146 - [c62]Risto Miikkulainen, Hormoz Shahrzad, Nigel Duffy, Philip M. Long:
How to select a winner in evolutionary optimization? SSCI 2017: 1-6 - 2016
- [i8]David P. Helmbold, Philip M. Long:
Dropout Versus Weight Decay for Deep Networks. CoRR abs/1602.04484 (2016) - 2015
- [j48]Avrim Blum, Philip M. Long:
Special Issue on New Theoretical Challenges in Machine Learning. Algorithmica 72(1): 191-192 (2015) - [j47]David P. Helmbold, Philip M. Long:
On the inductive bias of dropout. J. Mach. Learn. Res. 16: 3403-3454 (2015) - 2014
- [j46]Philip M. Long, Rocco A. Servedio
:
On the Weight of Halfspaces over Hamming Balls. SIAM J. Discret. Math. 28(3): 1035-1061 (2014) - [c61]Pranjal Awasthi, Maria-Florina Balcan, Philip M. Long:
The power of localization for efficiently learning linear separators with noise. STOC 2014: 449-458 - [c60]Anelia Angelova, Philip M. Long:
Benchmarking large-scale Fine-Grained Categorization. WACV 2014: 532-539 - [i7]David P. Helmbold, Philip M. Long:
On the Inductive Bias of Dropout. CoRR abs/1412.4736 (2014) - 2013
- [j45]Philip M. Long, Rocco A. Servedio:
Algorithms and hardness results for parallel large margin learning. J. Mach. Learn. Res. 14(1): 3105-3128 (2013) - [c59]Maria-Florina Balcan, Philip M. Long:
Active and passive learning of linear separators under log-concave distributions. COLT 2013: 288-316 - [c58]Philip M. Long, Rocco A. Servedio:
Consistency versus Realizable H-Consistency for Multiclass Classification. ICML (3) 2013: 801-809 - [c57]Philip M. Long, Rocco A. Servedio
:
Low-weight halfspaces for sparse boolean vectors. ITCS 2013: 21-36 - [i6]Pranjal Awasthi, Maria-Florina Balcan, Philip M. Long:
The Power of Localization for Efficiently Learning Linear Separators with Malicious Noise. CoRR abs/1307.8371 (2013) - 2012
- [j44]David P. Helmbold, Philip M. Long:
On the necessity of irrelevant variables. J. Mach. Learn. Res. 13: 2145-2170 (2012) - [j43]Nader H. Bshouty, Philip M. Long:
Linear classifiers are nearly optimal when hidden variables have diverse effects. Mach. Learn. 86(2): 209-231 (2012) - [c56]David P. Helmbold, Philip M. Long:
New Bounds for Learning Intervals with Implications for Semi-Supervised Learning. COLT 2012: 30.1-30.15 - [i5]David P. Helmbold, Philip M. Long:
On the Necessity of Irrelevant Variables. CoRR abs/1203.2557 (2012) - [i4]Maria-Florina Balcan, Philip M. Long:
Active and passive learning of linear separators under log-concave distributions. CoRR abs/1211.1082 (2012) - 2011
- [c55]David P. Helmbold, Philip M. Long:
On the Necessity of Irrelevant Variables. ICML 2011: 281-288 - [c54]Philip M. Long, Rocco A. Servedio:
Learning large-margin halfspaces with more malicious noise. NIPS 2011: 91-99 - [c53]Rocco A. Servedio, Philip M. Long:
Algorithms and hardness results for parallel large margin learning. NIPS 2011: 1314-1322 - 2010
- [j42]Philip M. Long, Rocco A. Servedio:
Random classification noise defeats all convex potential boosters. Mach. Learn. 78(3): 287-304 (2010) - [c52]Nader H. Bshouty, Philip M. Long:
Finding Planted Partitions in Nearly Linear Time using Arrested Spectral Clustering. ICML 2010: 135-142 - [c51]Philip M. Long, Rocco A. Servedio:
Restricted Boltzmann Machines are Hard to Approximately Evaluate or Simulate. ICML 2010: 703-710
2000 – 2009
- 2009
- [j41]Nader H. Bshouty, Yi Li, Philip M. Long:
Using the doubling dimension to analyze the generalization of learning algorithms. J. Comput. Syst. Sci. 75(6): 323-335 (2009) - [j40]Adam R. Klivans, Philip M. Long, Rocco A. Servedio:
Learning Halfspaces with Malicious Noise. J. Mach. Learn. Res. 10: 2715-2740 (2009) - [c50]Adam R. Klivans, Philip M. Long, Alex K. Tang:
Baum's Algorithm Learns Intersections of Halfspaces with Respect to Log-Concave Distributions. APPROX-RANDOM 2009: 588-600 - [c49]Nader H. Bshouty, Philip M. Long:
Linear Classifiers are Nearly Optimal When Hidden Variables Have Diverse Effect. COLT 2009 - [c48]Adam R. Klivans, Philip M. Long, Rocco A. Servedio:
Learning Halfspaces with Malicious Noise. ICALP (1) 2009: 609-621 - 2008
- [j39]Peter Auer
, Philip M. Long:
Guest editors' introduction: Special issue on learning theory. J. Comput. Syst. Sci. 74(8): 1227 (2008) - [j38]Philip M. Long, Frank Stephan
:
Preface. Theor. Comput. Sci. 405(3): 207-208 (2008) - [c47]Philip M. Long, Rocco A. Servedio:
Random classification noise defeats all convex potential boosters. ICML 2008: 608-615 - [c46]Philip M. Long, Rocco A. Servedio:
Adaptive Martingale Boosting. NIPS 2008: 977-984 - 2007
- [j37]Philip M. Long, Rocco A. Servedio, Hans Ulrich Simon
:
Discriminative learning can succeed where generative learning fails. Inf. Process. Lett. 103(4): 131-135 (2007) - [j36]Ofer Dekel, Philip M. Long, Yoram Singer:
Online Learning of Multiple Tasks with a Shared Loss. J. Mach. Learn. Res. 8: 2233-2264 (2007) - [c45]Zafer Barutçuoglu, Philip M. Long, Rocco A. Servedio:
One-Pass Boosting. NIPS 2007: 73-80 - [c44]Philip M. Long, Rocco A. Servedio:
Boosting the Area under the ROC Curve. NIPS 2007: 945-952 - 2006
- [c43]Philip Gross, Albert Boulanger, Marta Arias, David L. Waltz, Philip M. Long, Charles Lawson, Roger Anderson, Matthew Koenig, Mark Mastrocinque, William Fairechio, John A. Johnson, Serena Lee, Frank Doherty, Arthur Kressner:
Predicting Electricity Distribution Feeder Failures Using Machine Learning Susceptibility Analysis. AAAI 2006: 1705-1711 - [c42]José L. Balcázar, Philip M. Long, Frank Stephan:
Editors' Introduction. ALT 2006: 1-9 - [c41]Philip M. Long, Rocco A. Servedio:
Discriminative Learning Can Succeed Where Generative Learning Fails. COLT 2006: 319-334 - [c40]Ofer Dekel, Philip M. Long, Yoram Singer:
Online Multitask Learning. COLT 2006: 453-467 - [c39]Yi Li, Philip M. Long:
Learnability and the doubling dimension. NIPS 2006: 889-896 - [c38]Philip M. Long, Rocco A. Servedio:
Attribute-efficient learning of decision lists and linear threshold functions under unconcentrated distributions. NIPS 2006: 921-928 - [e2]José L. Balcázar, Philip M. Long, Frank Stephan:
Algorithmic Learning Theory, 17th International Conference, ALT 2006, Barcelona, Spain, October 7-10, 2006, Proceedings. Lecture Notes in Computer Science 4264, Springer 2006, ISBN 3-540-46649-5 [contents] - 2005
- [j35]Sanjoy Dasgupta, Philip M. Long:
Performance guarantees for hierarchical clustering. J. Comput. Syst. Sci. 70(4): 555-569 (2005) - [c37]Philip M. Long, Rocco A. Servedio:
Martingale Boosting. COLT 2005: 79-94 - [c36]Philip M. Long, Vinay Varadan, Sarah Gilman, Mark Treshock, Rocco A. Servedio:
Unsupervised evidence integration. ICML 2005: 521-528 - 2004
- [j34]Philip M. Long:
Efficient algorithms for learning functions with bounded variation. Inf. Comput. 188(1): 99-115 (2004) - [c35]Philip M. Long, Xinyu Wu:
Mistake Bounds for Maximum Entropy Discrimination. NIPS 2004: 833-840 - 2003
- [j33]Naoki Abe, Alan W. Biermann, Philip M. Long:
Reinforcement Learning with Immediate Rewards and Linear Hypotheses. Algorithmica 37(4): 263-293 (2003) - [j32]Philip M. Long:
An upper bound on the sample complexity of PAC-learning halfspaces with respect to the uniform distribution. Inf. Process. Lett. 87(5): 229-234 (2003) - [j31]Shai Ben-David, Nadav Eiron, Philip M. Long:
On the difficulty of approximately maximizing agreements. J. Comput. Syst. Sci. 66(3): 496-514 (2003) - [j30]Sanjoy Dasgupta, Wee Sun Lee, Philip M. Long:
A Theoretical Analysis of Query Selection for Collaborative Filtering. Mach. Learn. 51(3): 283-298 (2003) - [j29]Philip M. Long, Vinsensius Berlian Vega SN:
Boosting and Microarray Data. Mach. Learn. 52(1-2): 31-44 (2003) - [c34]Sanjoy Dasgupta, Philip M. Long:
Boosting with Diverse Base Classifiers. COLT 2003: 273-287 - 2002
- [j28]Yi Li, Philip M. Long:
The Relaxed Online Maximum Margin Algorithm. Mach. Learn. 46(1-3): 361-387 (2002) - [c33]Philip M. Long:
Minimum Majority Classification and Boosting. AAAI/IAAI 2002: 181-186 - 2001
- [j27]Yi Li, Philip M. Long, Aravind Srinivasan:
Improved Bounds on the Sample Complexity of Learning. J. Comput. Syst. Sci. 62(3): 516-527 (2001) - [j26]Yi Li, Philip M. Long, Aravind Srinivasan:
The one-inclusion graph algorithm is near-optimal for the prediction model of learning. IEEE Trans. Inf. Theory 47(3): 1257-1261 (2001) - [c32]Philip M. Long:
On Agnostic Learning with {0, *, 1}-Valued and Real-Valued Hypotheses. COLT/EuroCOLT 2001: 289-302 - [c31]Shai Ben-David, Philip M. Long, Yishay Mansour:
Agnostic Boosting. COLT/EuroCOLT 2001: 507-516 - [c30]Wee Sun Lee, Philip M. Long:
A Theoretical Analysis of Query Selection for Collaborative Filtering. COLT/EuroCOLT 2001: 517-528 - [c29]Philip M. Long:
Using the Pseudo-Dimension to Analyze Approximation Algorithms for Integer Programming. WADS 2001: 26-37 - 2000
- [j25]David P. Helmbold, Nick Littlestone, Philip M. Long:
Apple Tasting. Inf. Comput. 161(2): 85-139 (2000) - [j24]David P. Helmbold, Nick Littlestone, Philip M. Long:
On-Line Learning with Linear Loss Constraints. Inf. Comput. 161(2): 140-171 (2000) - [j23]Philip M. Long:
Improved bounds about on-line learning of smooth-functions of a single variable. Theor. Comput. Sci. 241(1-2): 25-35 (2000) - [c28]Shai Ben-David, Nadav Eiron, Philip M. Long:
On the Difficulty of Approximately Maximizing Agreements. COLT 2000: 266-274 - [c27]Yi Li, Philip M. Long, Aravind Srinivasan:
Improved bounds on the sample complexity of learning. SODA 2000: 309-318 - [i3]Peter Auer, Philip M. Long, Wolfgang Maass, Gerhard J. Woeginger:
On the Complexity of Function Learning. Electron. Colloquium Comput. Complex. TR00 (2000) - [i2]Peter Auer, Philip M. Long:
Simulating Access to Hidden Information while Learning. Electron. Colloquium Comput. Complex. TR00 (2000) - [i1]Peter Auer, Philip M. Long, Aravind Srinivasan:
Approximating Hyper-Rectangles: Learning and Pseudo-random Sets. Electron. Colloquium Comput. Complex. TR00 (2000)
1990 – 1999
- 1999
- [j22]P. Krishnan, Philip M. Long, Jeffrey Scott Vitter
:
Adaptive Disk Spindown via Optimal Rent-to-Buy in Probabilistic Environments. Algorithmica 23(1): 31-56 (1999) - [j21]Dzung T. Hoang, Philip M. Long, Jeffrey Scott Vitter
:
Dictionary Selection Using Partial Matching. Inf. Sci. 119(1-2): 57-72 (1999) - [j20]Peter Auer, Philip M. Long:
Structural Results About On-line Learning Models With and Without Queries. Mach. Learn. 36(3): 147-181 (1999) - [j19]Philip M. Long:
The Complexity of Learning According to Two Models of a Drifting Environment. Mach. Learn. 37(3): 337-354 (1999) - [j18]Philip M. Long, Apostol Natsev, Jeffrey Scott Vitter
:
Text compression via alphabet re-representation. Neural Networks 12(4-5): 755-765 (1999) - [c26]Naoki Abe, Philip M. Long:
Associative Reinforcement Learning using Linear Probabilistic Concepts. ICML 1999: 3-11 - [c25]Yi Li, Philip M. Long:
The Relaxed Online Maximum Margin Algorithm. NIPS 1999: 498-504 - [e1]Shai Ben-David, Philip M. Long:
Proceedings of the Twelfth Annual Conference on Computational Learning Theory, COLT 1999, Santa Cruz, CA, USA, July 7-9, 1999. ACM 1999, ISBN 1-58113-167-4 [contents] - 1998
- [j17]Peter L. Bartlett, Philip M. Long:
Prediction, Learning, Uniform Convergence, and Scale-Sensitive Dimensions. J. Comput. Syst. Sci. 56(2): 174-190 (1998) - [j16]Peter Auer, Philip M. Long, Aravind Srinivasan:
Approximating Hyper-Rectangles: Learning and Pseudorandom Sets. J. Comput. Syst. Sci. 57(3): 376-388 (1998) - [j15]Philip M. Long, Lei Tan:
PAC Learning Axis-aligned Rectangles with Respect to Product Distributions from Multiple-Instance Examples. Mach. Learn. 30(1): 7-21 (1998) - [j14]Dzung T. Hoang, Philip M. Long, Jeffrey Scott Vitter
:
Efficient cost measures for motion estimation at low bit rates. IEEE Trans. Circuits Syst. Video Technol. 8(4): 488-500 (1998) - [c24]Philip M. Long:
The complexity of learning according to two models of a drifting environment. COLT 1998: 116-125 - [c23]Philip M. Long:
On the Sample Complexity of Learning Functions with Bounded Variation. COLT 1998: 126-133 - 1997
- [j13]Rakesh D. Barve, Philip M. Long:
On the Complexity of Learning from Drifting Distributions. Inf. Comput. 138(2): 170-193 (1997) - [j12]Philip M. Long:
Guest Editor's Introduction. Mach. Learn. 27(1): 5 (1997) - [c22]Philip M. Long:
On-line Evaluation and Prediction using Linear Functions. COLT 1997: 21-31 - [c21]Philip M. Long, Apostol Natsev, Jeffrey Scott Vitter:
Text Compression Via Alphabet Re-Representation. Data Compression Conference 1997: 161-170 - [c20]Peter Auer, Philip M. Long, Aravind Srinivasan:
Approximating Hyper-Rectangles: Learning and Pseudo-Random Sets. STOC 1997: 314-323 - 1996
- [j11]Peter L. Bartlett
, Philip M. Long, Robert C. Williamson:
Fat-Shattering and the Learnability of Real-Valued Functions. J. Comput. Syst. Sci. 52(3): 434-452 (1996) - [j10]Nicolò Cesa-Bianchi, Philip M. Long, Manfred K. Warmuth:
Worst-case quadratic loss bounds for prediction using linear functions and gradient descent. IEEE Trans. Neural Networks 7(3): 604-619 (1996) - [c19]Philip M. Long:
Improved Bounds about On-line Learning of Smooth Functions of a Single Variable. ALT 1996: 26-36 - [c18]Rakesh D. Barve, Philip M. Long:
On the Complexity of Learning from Drifting Distributions. COLT 1996: 122-130 - [c17]Philip M. Long, Lei Tan:
PAC Learning Axis-Aligned Rectangles with Respect to Product Distributions from Multiple-Instance Examples. COLT 1996: 228-234 - [c16]Dzung T. Hoang, Philip M. Long, Jeffrey Scott Vitter:
Efficient Cost Measures for Motion Compensation at Low Bit Rates (Extended Abstract). Data Compression Conference 1996: 102-111 - 1995
- [j9]Nick Littlestone, Philip M. Long, Manfred K. Warmuth:
On-line Learning of Linear Functions. Comput. Complex. 5(1): 1-23 (1995) - [j8]Shai Ben-David, Nicolò Cesa-Bianchi, David Haussler, Philip M. Long:
Characterizations of Learnability for Classes of {0, ..., n}-Valued Functions. J. Comput. Syst. Sci. 50(1): 74-86 (1995) - [j7]David Haussler, Philip M. Long:
A Generalization of Sauer's Lemma. J. Comb. Theory A 71(2): 219-240 (1995) - [j6]Peter Auer, Philip M. Long, Wolfgang Maass, Gerhard J. Woeginger:
On the Complexity of Function Learning. Mach. Learn. 18(2-3): 187-230 (1995) - [j5]Don Kimber, Philip M. Long:
On-Line Learning of Smooth Functions of a Single Variable. Theor. Comput. Sci. 148(1): 141-156 (1995) - [j4]Philip M. Long:
On the sample complexity of PAC learning half-spaces against the uniform distribution. IEEE Trans. Neural Networks 6(6): 1556-1559 (1995) - [c15]Peter L. Bartlett, Philip M. Long:
More Theorems about Scale-sensitive Dimensions and Learning. COLT 1995: 392-401 - [c14]Dzung T. Hoang, Philip M. Long, Jeffrey Scott Vitter:
Multiple-Dictionary Coding Using Partial Matching. Data Compression Conference 1995: 272-281 - [c13]P. Krishnan, Philip M. Long, Jeffrey Scott Vitter:
Learning to Make Rent-to-Buy Decisions with Systems Applications. ICML 1995: 322-330 - 1994
- [j3]Philip M. Long, Manfred K. Warmuth:
Composite Geometric Concepts and Polynomial Predictability. Inf. Comput. 113(2): 230-252 (1994) - [j2]Philip M. Long:
Halfspace Learning, Linear Programming, and Nonmalicious Distributions. Inf. Process. Lett. 51(5): 245-250 (1994) - [j1]David P. Helmbold, Philip M. Long:
Tracking Drifting Concepts By Minimizing Disagreements. Mach. Learn. 14(1): 27-45 (1994) - [c12]Peter L. Bartlett, Philip M. Long, Robert C. Williamson:
Fat-Shattering and the Learnability of Real-Valued Functions. COLT 1994: 299-310 - [c11]Dzung T. Hoang, Philip M. Long, Jeffrey Scott Vitter:
Explicit Bit Minimization for Motion-Compensated Video Coding. Data Compression Conference 1994: 175-184 - [c10]Peter Auer, Philip M. Long:
Simulating access to hidden information while learning. STOC 1994: 263-272 - 1993
- [c9]Peter Auer, Philip M. Long, Wolfgang Maass, Gerhard J. Woeginger:
On the Complexity of Function Learning. COLT 1993: 392-401 - [c8]Nick Littlestone, Philip M. Long:
On-Line Learning with Linear Loss Constraints. COLT 1993: 412-421 - [c7]Nicolò Cesa-Bianchi, Philip M. Long, Manfred K. Warmuth:
Worst-Case Quadratic Loss Bounds for a Generalization of the Widrow-Hoff Rule. COLT 1993: 429-438 - 1992
- [c6]Don Kimber, Philip M. Long:
The Learning Complexity of Smooth Functions of a Single Variable. COLT 1992: 153-159 - [c5]Shai Ben-David, Nicolò Cesa-Bianchi, Philip M. Long:
Characterizations of Learnability for Classes of {O, ..., n}-Valued Functions. COLT 1992: 333-340 - [c4]David P. Helmbold, Nick Littlestone, Philip M. Long:
Apple Tasting and Nearly One-Sided Learning. FOCS 1992: 493-502 - 1991
- [c3]David P. Helmbold, Philip M. Long:
Tracking Drifting Concepts Using Random Examples. COLT 1991: 13-23 - [c2]Nick Littlestone, Philip M. Long, Manfred K. Warmuth:
On-Line Learning of Linear Functions. STOC 1991: 465-475 - 1990
- [c1]Philip M. Long, Manfred K. Warmuth:
Composite Geometric Concepts and Polynomial Predictability. COLT 1990: 273-287
Coauthor Index

manage site settings
To protect your privacy, all features that rely on external API calls from your browser are turned off by default. You need to opt-in for them to become active. All settings here will be stored as cookies with your web browser. For more information see our F.A.Q.
Unpaywalled article links
Add open access links from to the list of external document links (if available).
Privacy notice: By enabling the option above, your browser will contact the API of unpaywall.org to load hyperlinks to open access articles. Although we do not have any reason to believe that your call will be tracked, we do not have any control over how the remote server uses your data. So please proceed with care and consider checking the Unpaywall privacy policy.
Archived links via Wayback Machine
For web page which are no longer available, try to retrieve content from the of the Internet Archive (if available).
Privacy notice: By enabling the option above, your browser will contact the API of archive.org to check for archived content of web pages that are no longer available. Although we do not have any reason to believe that your call will be tracked, we do not have any control over how the remote server uses your data. So please proceed with care and consider checking the Internet Archive privacy policy.
Reference lists
Add a list of references from ,
, and
to record detail pages.
load references from crossref.org and opencitations.net
Privacy notice: By enabling the option above, your browser will contact the APIs of crossref.org, opencitations.net, and semanticscholar.org to load article reference information. Although we do not have any reason to believe that your call will be tracked, we do not have any control over how the remote server uses your data. So please proceed with care and consider checking the Crossref privacy policy and the OpenCitations privacy policy, as well as the AI2 Privacy Policy covering Semantic Scholar.
Citation data
Add a list of citing articles from and
to record detail pages.
load citations from opencitations.net
Privacy notice: By enabling the option above, your browser will contact the API of opencitations.net and semanticscholar.org to load citation information. Although we do not have any reason to believe that your call will be tracked, we do not have any control over how the remote server uses your data. So please proceed with care and consider checking the OpenCitations privacy policy as well as the AI2 Privacy Policy covering Semantic Scholar.
OpenAlex data
Load additional information about publications from .
Privacy notice: By enabling the option above, your browser will contact the API of openalex.org to load additional information. Although we do not have any reason to believe that your call will be tracked, we do not have any control over how the remote server uses your data. So please proceed with care and consider checking the information given by OpenAlex.
last updated on 2025-06-17 00:14 CEST by the dblp team
all metadata released as open data under CC0 1.0 license
see also: Terms of Use | Privacy Policy | Imprint