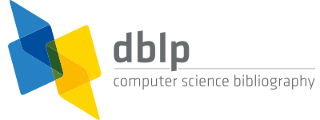


default search action
Martin Aumüller 0001
Person information
- affiliation: IT University of Copenhagen, Denmark
- affiliation (PhD 2015): TU Ilmenau, Germany
Other persons with the same name
- Martin Aumüller 0002
— Nokia (and 3 more)
Refine list

refinements active!
zoomed in on ?? of ?? records
view refined list in
export refined list as
2020 – today
- 2025
- [c27]Camilla Birch Okkels
, Martin Aumüller, Viktor Bello Thomsen, Arthur Zimek:
High-dimensional density-based clustering using locality-sensitive hashing. EDBT 2025: 694-706 - [c26]Martin Aumüller, Fabrizio Boninsegna, Francesco Silvestri:
Differentially Private High-Dimensional Approximate Range Counting, Revisited. FORC 2025: 15:1-15:24 - 2024
- [j12]Martin Aumüller, Christian Janos Lebeda, Boel Nelson
, Rasmus Pagh:
PLAN: Variance-Aware Private Mean Estimation. Proc. Priv. Enhancing Technol. 2024(3): 606-625 (2024) - [c25]Malte Helin Johnsen, Martin Aumüller
:
An Empirical Evaluation of Search Strategies for Locality-Sensitive Hashing: Lookup, Voting, and Natural Classifier Search. SISAP 2024: 155-169 - [c24]Camilla Birch Okkels
, Martin Aumüller
, Arthur Zimek
:
On the Design of Scalable Outlier Detection Methods Using Approximate Nearest Neighbor Graphs. SISAP 2024: 170-184 - [c23]Eric Sadit Tellez
, Martin Aumüller
, Vladimir Mic
:
Overview of the SISAP 2024 Indexing Challenge. SISAP 2024: 255-265 - [i22]Martin Aumüller, Fabrizio Boninsegna, Francesco Silvestri:
A Simple Linear Space Data Structure for ANN with Application in Differential Privacy. CoRR abs/2409.07187 (2024) - [i21]Harsha Vardhan Simhadri, Martin Aumüller, Amir Ingber, Matthijs Douze, George Williams, Magdalen Dobson Manohar, Dmitry Baranchuk, Edo Liberty, Frank Liu, Benjamin Landrum, Mazin Karjikar, Laxman Dhulipala, Meng Chen, Yue Chen, Rui Ma, Kai Zhang, Yuzheng Cai, Jiayang Shi, Yizhuo Chen, Weiguo Zheng, Zihao Wan, Jie Yin, Ben Huang:
Results of the Big ANN: NeurIPS'23 competition. CoRR abs/2409.17424 (2024) - 2023
- [j11]Martin Aumüller, Matteo Ceccarello:
Recent Approaches and Trends in Approximate Nearest Neighbor Search, with Remarks on Benchmarking. IEEE Data Eng. Bull. 47(3): 89-105 (2023) - [c22]Omar Shahbaz Khan
, Martin Aumüller
, Björn Þór Jónsson
:
Suitability of Nearest Neighbour Indexes for Multimedia Relevance Feedback. SISAP 2023: 133-147 - [c21]Martin Aumüller
, Matteo Ceccarello
:
Solving k-Closest Pairs in High-Dimensional Data. SISAP 2023: 200-214 - [c20]Eric Sadit Tellez
, Martin Aumüller
, Edgar Chávez
:
Overview of the SISAP 2023 Indexing Challenge. SISAP 2023: 255-264 - [i20]Martin Aumüller, Christian Janos Lebeda, Boel Nelson, Rasmus Pagh:
PLAN: Variance-Aware Private Mean Estimation. CoRR abs/2306.08745 (2023) - 2022
- [j10]Martin Aumüller, Sariel Har-Peled
, Sepideh Mahabadi, Rasmus Pagh
, Francesco Silvestri:
Sampling near neighbors in search for fairness. Commun. ACM 65(8): 83-90 (2022) - [j9]Christian Janos Lebeda, Martin Aumüller
, Rasmus Pagh
:
Representing Sparse Vectors with Differential Privacy, Low Error, Optimal Space, and Fast Access. J. Priv. Confidentiality 12(2) (2022) - [j8]Martin Aumüller
, Sariel Har-Peled
, Sepideh Mahabadi
, Rasmus Pagh
, Francesco Silvestri
:
Sampling a Near Neighbor in High Dimensions - Who is the Fairest of Them All? ACM Trans. Database Syst. 47(1): 4:1-4:40 (2022) - [c19]Matti Karppa, Martin Aumüller, Rasmus Pagh:
DEANN: Speeding up Kernel-Density Estimation using Approximate Nearest Neighbor Search. AISTATS 2022: 3108-3137 - [c18]Martin Aumüller, Matteo Ceccarello
:
Implementing Distributed Similarity Joins using Locality Sensitive Hashing. EDBT 2022: 1:78-1:90 - [i19]Harsha Vardhan Simhadri, George Williams, Martin Aumüller, Matthijs Douze, Artem Babenko, Dmitry Baranchuk, Qi Chen, Lucas Hosseini, Ravishankar Krishnaswamy, Gopal Srinivasa, Suhas Jayaram Subramanya, Jingdong Wang:
Results of the NeurIPS'21 Challenge on Billion-Scale Approximate Nearest Neighbor Search. CoRR abs/2205.03763 (2022) - 2021
- [j7]Martin Aumüller
, Matteo Ceccarello
:
The role of local dimensionality measures in benchmarking nearest neighbor search. Inf. Syst. 101: 101807 (2021) - [j6]Martin Aumüller, Sariel Har-Peled
, Sepideh Mahabadi, Rasmus Pagh
, Francesco Silvestri:
Fair near neighbor search via sampling. SIGMOD Rec. 50(1): 42-49 (2021) - [c17]Martin Aumüller, Christian Janos Lebeda, Rasmus Pagh
:
Differentially Private Sparse Vectors with Low Error, Optimal Space, and Fast Access. CCS 2021: 1223-1236 - [c16]Harsha Vardhan Simhadri, George Williams, Martin Aumüller, Matthijs Douze, Artem Babenko, Dmitry Baranchuk, Qi Chen, Lucas Hosseini, Ravishankar Krishnaswamy, Gopal Srinivasa, Suhas Jayaram Subramanya, Jingdong Wang:
Results of the NeurIPS'21 Challenge on Billion-Scale Approximate Nearest Neighbor Search. NeurIPS (Competition and Demos) 2021: 177-189 - [i18]Martin Aumüller, Sariel Har-Peled, Sepideh Mahabadi, Rasmus Pagh, Francesco Silvestri:
Sampling a Near Neighbor in High Dimensions - Who is the Fairest of Them All? CoRR abs/2101.10905 (2021) - [i17]Martin Aumüller, Christian Janos Lebeda, Rasmus Pagh:
Differentially private sparse vectors with low error, optimal space, and fast access. CoRR abs/2106.10068 (2021) - [i16]Matti Karppa, Martin Aumüller, Rasmus Pagh:
DEANN: Speeding up Kernel-Density Estimation using Approximate Nearest Neighbor Search. CoRR abs/2107.02736 (2021) - 2020
- [j5]Martin Aumüller, Erik Bernhardsson, Alexander John Faithfull:
ANN-Benchmarks: A benchmarking tool for approximate nearest neighbor algorithms. Inf. Syst. 87 (2020) - [c15]Quoc-Tuan Truong, Hady W. Lauw
, Martin Aumüller, Naoko Nitta:
Reproducibility Companion Paper: Visual Sentiment Analysis for Review Images with Item-Oriented and User-Oriented CNN. ACM Multimedia 2020: 4444-4447 - [c14]Martin Aumüller, Rasmus Pagh, Francesco Silvestri:
Fair Near Neighbor Search: Independent Range Sampling in High Dimensions. PODS 2020: 191-204 - [c13]Martin Aumüller
, Anders Bourgeat, Jana Schmurr:
Differentially Private Sketches for Jaccard Similarity Estimation. SISAP 2020: 18-32 - [c12]Martin Aumüller
, Matteo Ceccarello
:
Running Experiments with Confidence and Sanity. SISAP 2020: 387-395 - [c11]Martin Aumüller:
Algorithm Engineering for High-Dimensional Similarity Search Problems (Invited Talk). SEA 2020: 1:1-1:3 - [e1]Shin'ichi Satoh, Lucia Vadicamo
, Arthur Zimek
, Fabio Carrara
, Ilaria Bartolini
, Martin Aumüller
, Björn Þór Jónsson
, Rasmus Pagh
:
Similarity Search and Applications - 13th International Conference, SISAP 2020, Copenhagen, Denmark, September 30 - October 2, 2020, Proceedings. Lecture Notes in Computer Science 12440, Springer 2020, ISBN 978-3-030-60935-1 [contents] - [i15]Martin Aumüller, Anders Bourgeat, Jana Schmurr:
Differentially Private Sketches for Jaccard Similarity Estimation. CoRR abs/2008.08134 (2020)
2010 – 2019
- 2019
- [j4]Martin Aumüller, Martin Dietzfelbinger
, Clemens Heuberger, Daniel Krenn
, Helmut Prodinger:
Dual-Pivot Quicksort: Optimality, Analysis and Zeros of Associated Lattice Paths. Comb. Probab. Comput. 28(4): 485-518 (2019) - [c10]Martin Aumüller, Nikolaj Hass:
Simple and Fast BlockQuicksort using Lomuto's Partitioning Scheme. ALENEX 2019: 15-26 - [c9]Martin Aumüller, Tobias Christiani, Rasmus Pagh
, Michael Vesterli:
PUFFINN: Parameterless and Universally Fast FInding of Nearest Neighbors. ESA 2019: 10:1-10:16 - [c8]Martin Aumüller, Matteo Ceccarello:
Benchmarking Nearest Neighbor Search: Influence of Local Intrinsic Dimensionality and Result Diversity in Real-World Datasets. EDML@SDM 2019: 14-23 - [c7]Martin Aumüller
, Matteo Ceccarello
:
The Role of Local Intrinsic Dimensionality in Benchmarking Nearest Neighbor Search. SISAP 2019: 113-127 - [i14]Martin Aumüller, Rasmus Pagh, Francesco Silvestri:
Fair Near Neighbor Search: Independent Range Sampling in High Dimensions. CoRR abs/1906.01859 (2019) - [i13]Martin Aumüller, Tobias Christiani, Rasmus Pagh, Michael Vesterli:
PUFFINN: Parameterless and Universally Fast FInding of Nearest Neighbors. CoRR abs/1906.12211 (2019) - [i12]Martin Aumüller, Matteo Ceccarello:
The Role of Local Intrinsic Dimensionality in Benchmarking Nearest Neighbor Search. CoRR abs/1907.07387 (2019) - 2018
- [c6]Martin Aumüller, Tobias Christiani, Rasmus Pagh, Francesco Silvestri
:
Distance-Sensitive Hashing. PODS 2018: 89-104 - [i11]Martin Aumüller, Erik Bernhardsson, Alexander John Faithfull:
ANN-Benchmarks: A Benchmarking Tool for Approximate Nearest Neighbor Algorithms. CoRR abs/1807.05614 (2018) - [i10]Martin Aumüller, Nikolaj Hass:
Simple and Fast BlockQuicksort using Lomuto's Partitioning Scheme. CoRR abs/1810.12047 (2018) - 2017
- [c5]Martin Aumüller, Erik Bernhardsson, Alexander John Faithfull:
ANN-Benchmarks: A Benchmarking Tool for Approximate Nearest Neighbor Algorithms. SISAP 2017: 34-49 - [c4]Thomas D. Ahle, Martin Aumüller, Rasmus Pagh:
Parameter-free Locality Sensitive Hashing for Spherical Range Reporting. SODA 2017: 239-256 - [i9]Martin Aumüller, Tobias Christiani, Rasmus Pagh, Francesco Silvestri:
Distance-sensitive hashing. CoRR abs/1703.07867 (2017) - [i8]Martin Dietzfelbinger, Michael Mitzenmacher, Rasmus Pagh, David P. Woodruff, Martin Aumüller:
Theory and Applications of Hashing (Dagstuhl Seminar 17181). Dagstuhl Reports 7(5): 1-21 (2017) - 2016
- [j3]Martin Aumüller, Martin Dietzfelbinger
:
Optimal Partitioning for Dual-Pivot Quicksort. ACM Trans. Algorithms 12(2): 18:1-18:36 (2016) - [j2]Martin Aumüller, Martin Dietzfelbinger
, Pascal Klaue:
How Good Is Multi-Pivot Quicksort? ACM Trans. Algorithms 13(1): 8:1-8:47 (2016) - [i7]Martin Aumüller, Martin Dietzfelbinger, Clemens Heuberger, Daniel Krenn, Helmut Prodinger:
Counting Zeros in Random Walks on the Integers and Analysis of Optimal Dual-Pivot Quicksort. CoRR abs/1602.04031 (2016) - [i6]Thomas D. Ahle, Martin Aumüller, Rasmus Pagh:
High-dimensional Spherical Range Reporting by Output-Sensitive Multi-Probing LSH. CoRR abs/1605.02673 (2016) - [i5]Martin Aumüller, Martin Dietzfelbinger, Philipp Woelfel:
A Simple Hash Class with Strong Randomness Properties in Graphs and Hypergraphs. CoRR abs/1611.00029 (2016) - [i4]Martin Aumüller, Martin Dietzfelbinger, Clemens Heuberger, Daniel Krenn, Helmut Prodinger:
Dual-Pivot Quicksort: Optimality, Analysis and Zeros of Associated Lattice Paths. CoRR abs/1611.00258 (2016) - 2015
- [b1]Martin Aumüller:
On the Analysis of Two Fundamental Randomized Algorithms - Multi-Pivot Quicksort and Efficient Hash Functions. Technische Universität Ilmenau, Germany, 2015 - [i3]Martin Aumüller, Martin Dietzfelbinger, Pascal Klaue:
How Good is Multi-Pivot Quicksort? CoRR abs/1510.04676 (2015) - 2014
- [j1]Martin Aumüller, Martin Dietzfelbinger
, Philipp Woelfel:
Explicit and Efficient Hash Families Suffice for Cuckoo Hashing with a Stash. Algorithmica 70(3): 428-456 (2014) - 2013
- [c3]Martin Aumüller, Martin Dietzfelbinger:
Optimal Partitioning for Dual Pivot Quicksort - (Extended Abstract). ICALP (1) 2013: 33-44 - [i2]Martin Aumüller, Martin Dietzfelbinger:
Optimal Partitioning for Dual Pivot Quicksort. CoRR abs/1303.5217 (2013) - 2012
- [c2]Martin Aumüller, Martin Dietzfelbinger, Philipp Woelfel:
Explicit and Efficient Hash Families Suffice for Cuckoo Hashing with a Stash. ESA 2012: 108-120 - [i1]Martin Aumüller, Martin Dietzfelbinger, Philipp Woelfel:
Explicit and Efficient Hash Families Suffice for Cuckoo Hashing with a Stash. CoRR abs/1204.4431 (2012)
2000 – 2009
- 2009
- [c1]Martin Aumüller, Martin Dietzfelbinger
, Michael Rink:
Experimental Variations of a Theoretically Good Retrieval Data Structure. ESA 2009: 742-751
Coauthor Index

manage site settings
To protect your privacy, all features that rely on external API calls from your browser are turned off by default. You need to opt-in for them to become active. All settings here will be stored as cookies with your web browser. For more information see our F.A.Q.
Unpaywalled article links
Add open access links from to the list of external document links (if available).
Privacy notice: By enabling the option above, your browser will contact the API of unpaywall.org to load hyperlinks to open access articles. Although we do not have any reason to believe that your call will be tracked, we do not have any control over how the remote server uses your data. So please proceed with care and consider checking the Unpaywall privacy policy.
Archived links via Wayback Machine
For web page which are no longer available, try to retrieve content from the of the Internet Archive (if available).
Privacy notice: By enabling the option above, your browser will contact the API of archive.org to check for archived content of web pages that are no longer available. Although we do not have any reason to believe that your call will be tracked, we do not have any control over how the remote server uses your data. So please proceed with care and consider checking the Internet Archive privacy policy.
Reference lists
Add a list of references from ,
, and
to record detail pages.
load references from crossref.org and opencitations.net
Privacy notice: By enabling the option above, your browser will contact the APIs of crossref.org, opencitations.net, and semanticscholar.org to load article reference information. Although we do not have any reason to believe that your call will be tracked, we do not have any control over how the remote server uses your data. So please proceed with care and consider checking the Crossref privacy policy and the OpenCitations privacy policy, as well as the AI2 Privacy Policy covering Semantic Scholar.
Citation data
Add a list of citing articles from and
to record detail pages.
load citations from opencitations.net
Privacy notice: By enabling the option above, your browser will contact the API of opencitations.net and semanticscholar.org to load citation information. Although we do not have any reason to believe that your call will be tracked, we do not have any control over how the remote server uses your data. So please proceed with care and consider checking the OpenCitations privacy policy as well as the AI2 Privacy Policy covering Semantic Scholar.
OpenAlex data
Load additional information about publications from .
Privacy notice: By enabling the option above, your browser will contact the API of openalex.org to load additional information. Although we do not have any reason to believe that your call will be tracked, we do not have any control over how the remote server uses your data. So please proceed with care and consider checking the information given by OpenAlex.
last updated on 2025-06-04 22:04 CEST by the dblp team
all metadata released as open data under CC0 1.0 license
see also: Terms of Use | Privacy Policy | Imprint