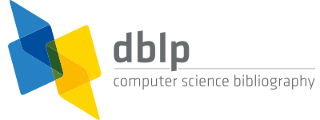


default search action
Michael Biehl
Person information
- affiliation: University of Groningen, Netherlands
Refine list

refinements active!
zoomed in on ?? of ?? records
view refined list in
export refined list as
2020 – today
- 2025
- [j54]Elina L. van den Brandhof, Inge Tuitert, A. M. Madelein van der Stouwe, Jan W. J. Elting, Jelle R. Dalenberg, Tatiana Svorenova, Marrit R. Klamer, Ramesh S. Marapin, Michael Biehl, Marina A. J. Tijssen:
Explainable machine learning for movement disorders - Classification of tremor and myoclonus. Comput. Biol. Medicine 192: 110180 (2025) - [j53]Sreejita Ghosh
, Elizabeth Sarah Baranowski
, Michael Biehl
, Wiebke Arlt
, Peter Tiño
, Kerstin Bunte
:
Interpretable modelling and visualization of biomedical data. Neurocomputing 626: 129405 (2025) - [i24]Marco Canducci, Lida Abdi, Alessandro Prete, Roland J. Veen, Michael Biehl, Wiebke Arlt, Peter Tino:
Discriminative Subspace Emersion from learning feature relevances across different populations. CoRR abs/2504.00176 (2025) - 2024
- [j52]Rick van Veen
, Neha Rajendra Bari Tamboli
, Sofie Lövdal
, Sanne K. Meles, Remco J. Renken
, Gert-Jan de Vries
, Dario Arnaldi
, Silvia Morbelli, Pedro Clavero, José A. Obeso, Maria C. Rodriguez Oroz, Klaus Leonard Leenders, Thomas Villmann, Michael Biehl
:
Subspace corrected relevance learning with application in neuroimaging. Artif. Intell. Medicine 149: 102786 (2024) - [j51]Sofie Lövdal
, Michael Biehl
:
Iterated Relevance Matrix Analysis (IRMA) for the identification of class-discriminative subspaces. Neurocomputing 577: 127367 (2024) - [c86]Frederieke Richert, Otavio Citton, Michael Biehl:
On-line Learning Dynamics in Layered Neural Networks with Arbitrary Activation Functions. ESANN 2024 - [c85]Roland Veen, Christodoulos Hadjichristodoulou, Michael Biehl:
Interpreting Hybrid AI through Autodecoded Latent Space Entities. ESANN 2024 - [c84]Luca Barillaro
, Marianna Milano
, Maria Eugenia Caligiuri
, Jelle R. Dalenberg
, Giuseppe Agapito
, Michael Biehl
, Mario Cannataro
:
A Graph-Theory Based fMRI Analysis. ICCS (6) 2024: 72-85 - [c83]Michael Biehl
, David Pavlov, Alice J. Sitch
, Alessandro Prete
, Wiebke Arlt
:
Interpretable Machine Learning in Endocrinology: A Diagnostic Tool in Primary Aldosteronism. WSOM+ 2024: 96-105 - [i23]Sofie Lövdal, Michael Biehl:
Iterated Relevance Matrix Analysis (IRMA) for the identification of class-discriminative subspaces. CoRR abs/2401.12842 (2024) - [i22]Filip Ilievski, Barbara Hammer, Frank van Harmelen, Benjamin Paassen, Sascha Saralajew, Ute Schmid, Michael Biehl, Marianna Bolognesi, Xin Luna Dong, Kiril Gashteovski, Pascal Hitzler, Giuseppe Marra, Pasquale Minervini, Martin Mundt, Axel-Cyrille Ngonga Ngomo, Alessandro Oltramari, Gabriella Pasi, Zeynep G. Saribatur, Luciano Serafini, John Shawe-Taylor, Vered Shwartz, Gabriella Skitalinskaya, Clemens Stachl, Gido M. van de Ven, Thomas Villmann:
Aligning Generalisation Between Humans and Machines. CoRR abs/2411.15626 (2024) - 2023
- [c82]Michael Biehl, Sofie Lövdal:
Improved Interpretation of Feature Relevances: Iterated Relevance Matrix Analysis (IRMA). ESANN 2023 - [c81]Frederieke Richert, Michiel Straat, Elisa Oostwal, Michael Biehl:
Layered Neural Networks with GELU Activation, a Statistical Mechanics Analysis. ESANN 2023 - 2022
- [j50]Rick van Veen
, Sanne K. Meles, Remco J. Renken
, Fransje E. Reesink, Wolfgang H. Oertel, Annette Janzen, Gert-Jan de Vries, Klaus Leonard Leenders, Michael Biehl
:
FDG-PET combined with learning vector quantization allows classification of neurodegenerative diseases and reveals the trajectory of idiopathic REM sleep behavior disorder. Comput. Methods Programs Biomed. 225: 107042 (2022) - [j49]Michiel Straat, Fthi Abadi, Zhuoyun Kan, Christina Göpfert
, Barbara Hammer
, Michael Biehl
:
Supervised learning in the presence of concept drift: a modelling framework. Neural Comput. Appl. 34(1): 101-118 (2022) - [c80]Thomas Villmann
, Daniel Staps, Jensun Ravichandran
, Sascha Saralajew
, Michael Biehl
, Marika Kaden
:
A Learning Vector Quantization Architecture for Transfer Learning Based Classification in Case of Multiple Sources by Means of Null-Space Evaluation. IDA 2022: 354-364 - [i21]Sreejita Ghosh
, Elizabeth Sarah Baranowski, Michael Biehl, Wiebke Arlt, Peter Tino, Kerstin Bunte:
Interpretable Models Capable of Handling Systematic Missingness in Imbalanced Classes and Heterogeneous Datasets. CoRR abs/2206.02056 (2022) - [i20]Samira Rezaei, John P. McKean, Michael Biehl, W. de Roo, A. Lafontaine:
A machine learning based approach to gravitational lens identification with the International LOFAR Telescope. CoRR abs/2207.10698 (2022) - 2021
- [j48]Godliver Owomugisha
, Friedrich Melchert
, Ernest Mwebaze
, John A. Quinn
, Michael Biehl
:
Matrix Relevance Learning From Spectral Data for Diagnosing Cassava Diseases. IEEE Access 9: 83355-83363 (2021) - [j47]Rick van Veen, Michael Biehl, Gert-Jan de Vries:
sklvq: Scikit Learning Vector Quantization. J. Mach. Learn. Res. 22: 231:1-231:6 (2021) - [i19]Samira Rezaei, John P. McKean, Michael Biehl, Amir Javadpour:
DECORAS: detection and characterization of radio-astronomical sources using deep learning. CoRR abs/2109.09077 (2021) - 2020
- [j46]Rick van Veen
, Vita Gurvits, Rosalie V. Kogan
, Sanne K. Meles, Gert-Jan de Vries, Remco J. Renken
, Maria C. Rodriguez-Oroz
, Rafael Rodriguez-Rojas
, Dario Arnaldi, Stefano Raffa, Bauke M. de Jong
, Klaus Leonard Leenders
, Michael Biehl
:
An application of generalized matrix learning vector quantization in neuroimaging. Comput. Methods Programs Biomed. 197: 105708 (2020) - [j45]Maximilian Münch, Christoph Raab, Michael Biehl
, Frank-Michael Schleif
:
Data-Driven Supervised Learning for Life Science Data. Frontiers Appl. Math. Stat. 6: 553000 (2020) - [j44]Lukas Pfannschmidt
, Jonathan Jakob, Fabian Hinder, Michael Biehl
, Peter Tiño
, Barbara Hammer
:
Feature relevance determination for ordinal regression in the context of feature redundancies and privileged information. Neurocomputing 416: 266-279 (2020) - [j43]Michiel Straat, Marika Kaden, Matthias Gay, Thomas Villmann, Alexander Lampe, Udo Seiffert
, Michael Biehl
, Friedrich Melchert
:
Learning vector quantization and relevances in complex coefficient space. Neural Comput. Appl. 32(24): 18085-18099 (2020) - [j42]Friedrich Melchert
, Gabriele Bani, Udo Seiffert
, Michael Biehl
:
Adaptive basis functions for prototype-based classification of functional data. Neural Comput. Appl. 32(24): 18213-18223 (2020) - [c79]Godliver Owomugisha, Ephraim Nuwamanya
, John A. Quinn, Michael Biehl
, Ernest Mwebaze:
Early detection of plant diseases using spectral data. APPIS 2020: 26:1-26:6 - [c78]Godliver Owomugisha, Pius K. B. Mugagga, Friedrich Melchert, Ernest Mwebaze, John A. Quinn, Michael Biehl
:
A low-cost 3-D printed smartphone add-on spectrometer for diagnosis of crop diseases in field. COMPASS 2020: 331-332 - [c77]Maximilian Münch, Christoph Raab, Michael Biehl
, Frank-Michael Schleif
:
Structure Preserving Encoding of Non-euclidean Similarity Data. ICPRAM 2020: 43-51 - [c76]Maximilian Münch
, Michiel Straat
, Michael Biehl
, Frank-Michael Schleif
:
Complex-Valued Embeddings of Generic Proximity Data. S+SSPR 2020: 14-23 - [i18]Michiel Straat, Fthi Abadi, Zhuoyun Kan, Christina Göpfert, Barbara Hammer, Michael Biehl
:
Supervised Learning in the Presence of Concept Drift: A modelling framework. CoRR abs/2005.10531 (2020) - [i17]Maximilian Münch, Michiel Straat, Michael Biehl
, Frank-Michael Schleif:
Complex-valued embeddings of generic proximity data. CoRR abs/2008.13454 (2020)
2010 – 2019
- 2019
- [j41]Aleke Nolte, Lingyu Wang, Maciej Bilicki, Benne Holwerda, Michael Biehl
:
Galaxy classification: A machine learning analysis of GAMA catalogue data. Neurocomputing 342: 172-190 (2019) - [c75]Michael Biehl
:
The Statistical Physics of Learning Revisited: Typical Learning Curves in Model Scenarios. BrainComp 2019: 128-142 - [c74]Amey Bhole
, Owen Falzon, Michael Biehl
, George Azzopardi
:
A Computer Vision Pipeline that Uses Thermal and RGB Images for the Recognition of Holstein Cattle. CAIP (2) 2019: 108-119 - [c73]Michael Biehl, Nestor Caticha, Manfred Opper, Thomas Villmann:
Statistical physics of learning and inference. ESANN 2019 - [c72]Lukas Pfannschmidt, Jonathan Jakob, Michael Biehl, Peter Tiño, Barbara Hammer:
Feature relevance bounds for ordinal regression. ESANN 2019 - [c71]Michiel Straat, Michael Biehl:
On-line learning dynamics of ReLU neural networks using statistical physics techniques. ESANN 2019 - [c70]Michael Biehl
, Fthi Abadi, Christina Göpfert
, Barbara Hammer
:
Prototype-Based Classifiers in the Presence of Concept Drift: A Modelling Framework. WSOM+ 2019: 210-221 - [i16]Lukas Pfannschmidt, Jonathan Jakob, Michael Biehl
, Peter Tiño, Barbara Hammer
:
Feature Relevance Bounds for Ordinal Regression. CoRR abs/1902.07662 (2019) - [i15]Michael Biehl
, Fthi Abadi, Christina Göpfert, Barbara Hammer:
Prototype-based classifiers in the presence of concept drift: A modelling framework. CoRR abs/1903.07273 (2019) - [i14]Michiel Straat, Michael Biehl
:
On-line learning dynamics of ReLU neural networks using statistical physics techniques. CoRR abs/1903.07378 (2019) - [i13]Aleke Nolte, Lingyu Wang, Maciej Bilicki, Benne Holwerda, Michael Biehl:
Galaxy classification: A machine learning analysis of GAMA catalogue data. CoRR abs/1903.07749 (2019) - [i12]Elisa Oostwal, Michiel Straat, Michael Biehl
:
Hidden Unit Specialization in Layered Neural Networks: ReLU vs. Sigmoidal Activation. CoRR abs/1910.07476 (2019) - [i11]Lukas Pfannschmidt, Jonathan Jakob, Fabian Hinder, Michael Biehl
, Peter Tiño, Barbara Hammer
:
Feature Relevance Determination for Ordinal Regression in the Context of Feature Redundancies and Privileged Information. CoRR abs/1912.04832 (2019) - 2018
- [j40]Thomas Villmann, Marika Kaden, Wieland Hermann, Michael Biehl
:
Learning vector quantization classifiers for ROC-optimization. Comput. Stat. 33(3): 1173-1194 (2018) - [j39]Michiel Straat
, Fthi Abadi, Christina Göpfert
, Barbara Hammer
, Michael Biehl
:
Statistical Mechanics of On-Line Learning Under Concept Drift. Entropy 20(10): 775 (2018) - [j38]Fabio Aiolli, Michael Biehl
, Luca Oneto
:
Advances in artificial neural networks, machine learning and computational intelligence. Neurocomputing 298: 1-3 (2018) - [c69]Rick van Veen
, Lidia Talavera-Martínez
, Rosalie V. Kogan
, Sanne K. Meles, Deborah Mudali, Jos B. T. M. Roerdink, Federico Massa
, M. Grazzini, Jose A. Obeso, Maria C. Rodriguez-Oroz, Klaus Leonard Leenders, Remco J. Renken
, J. J. G. de Vries, Michael Biehl
:
Machine Learning Based Analysis of FDG-PET Image Data for the Diagnosis of Neurodegenerative Diseases. APPIS 2018: 280-289 - [c68]Michael Biehl, Kerstin Bunte, Giuseppe Longo, Peter Tiño:
Machine learning and data analysis in astroinformatics. ESANN 2018 - [c67]Aleke Nolte, Lingyu Wang, Michael Biehl:
Prototype-based analysis of GAMA galaxy catalogue data. ESANN 2018 - 2017
- [c66]Michael Biehl
:
Biomedical Applications of Prototype Based Classifiers and Relevance Learning. AlCoB 2017: 3-23 - [c65]Gyan Bhanot, Michael Biehl, Thomas Villmann, Dietlind Zühlke:
Biomedical data analysis in translational research: integration of expert knowledge and interpretable models. ESANN 2017 - [c64]Sreejita Ghosh, Elizabeth Sarah Baranowski, Rick van Veen, Gert-Jan de Vries, Michael Biehl, Wiebke Arlt, Peter Tiño, Kerstin Bunte:
Comparison of strategies to learn from imbalanced classes for computer aided diagnosis of inborn steroidogenic disorders. ESANN 2017 - [c63]Mohammad Mohammadi, Michael Biehl
, Andrea Villmann, Thomas Villmann:
Sequence Learning in Unsupervised and Supervised Vector Quantization Using Hankel Matrices. ICAISC (1) 2017: 131-142 - [c62]Andreas C. Neocleous, Costas Neocleous, Christos N. Schizas, Michael Biehl
, Nicolai Petkov:
Marker selection for the detection of trisomy 21 using generalized matrix learning vector quantization. IJCNN 2017: 3704-3708 - [c61]Thomas Villmann, Michael Biehl
, Andrea Villmann, Sascha Saralajew:
Fusion of deep learning architectures, multilayer feedforward networks and learning vector quantizers for deep classification learning. WSOM 2017: 69-76 - [c60]Michiel Straat
, Marika Kaden, Matthias Gay, Thomas Villmann, Alexander Lampe, Udo Seiffert
, Michael Biehl
, Friedrich Melchert:
Prototypes and matrix relevance learning in complex fourier space. WSOM 2017: 139-144 - [c59]Gabriele Bani, Udo Seiffert
, Michael Biehl
, Friedrich Melchert:
Adaptive basis functions for prototype-based classification of functional data. WSOM 2017: 145-152 - [c58]Michael LeKander, Michael Biehl
, Harm de Vries:
Empirical evaluation of gradient methods for matrix learning vector quantization. WSOM 2017: 197-204 - 2016
- [j37]Frank-Michael Schleif
, Barbara Hammer
, Javier Gonzalez Monroy
, Javier González Jiménez, José-Luis Blanco-Claraco
, Michael Biehl
, Nicolai Petkov:
Odor recognition in robotics applications by discriminative time-series modeling. Pattern Anal. Appl. 19(1): 207-220 (2016) - [c57]Michael Biehl
, Barbara Hammer
, Thomas Villmann:
Prototype-based Models for the Supervised Learning of Classification Schemes. Astroinformatics 2016: 129-138 - [c56]Gargi Mukherjee, Gyan Bhanot, Kevin Raines, Srikanth Sastry
, Sebastian Doniach, Michael Biehl
:
Predicting recurrence in clear cell Renal Cell Carcinoma: Analysis of TCGA data using outlier analysis and generalized matrix LVQ. CEC 2016: 656-661 - [c55]Deborah Mudali, Michael Biehl
, Klaus Leonard Leenders, Jos B. T. M. Roerdink:
LVQ and SVM Classification of FDG-PET Brain Data. WSOM 2016: 205-215 - [c54]Matthias Gay, Marika Kaden, Michael Biehl
, Alexander Lampe, Thomas Villmann:
Complex Variants of GLVQ Based on Wirtinger's Calculus. WSOM 2016: 293-303 - [c53]Friedrich Melchert, Udo Seiffert
, Michael Biehl
:
Functional Representation of Prototypes in LVQ and Relevance Learning. WSOM 2016: 317-327 - [c52]Ernest Mwebaze, Michael Biehl
:
Prototype-Based Classification for Image Analysis and Its Application to Crop Disease Diagnosis. WSOM 2016: 329-339 - [i10]Gyan Bhanot, Michael Biehl
, Thomas Villmann, Dietlind Zühlke
:
Integration of Expert Knowledge for Interpretable Models in Biomedical Data Analysis (Dagstuhl Seminar 16261). Dagstuhl Reports 6(6): 88-110 (2016) - 2015
- [j36]Michael Biehl
, Peter J. Sadowski
, Gyan Bhanot, Erhan Bilal
, Adel Dayarian, Pablo Meyer, Raquel Norel
, Kahn Rhrissorrakrai, Michael D. Zeller, Sahand Hormoz:
Inter-species prediction of protein phosphorylation in the sbv IMPROVER species translation challenge. Bioinform. 31(4): 453-461 (2015) - [j35]Adel Dayarian, Roberto Romero
, Zhiming Wang
, Michael Biehl
, Erhan Bilal
, Sahand Hormoz, Pablo Meyer, Raquel Norel
, Kahn Rhrissorrakrai, Gyan Bhanot, Feng Luo, Adi L. Tarca:
Predicting protein phosphorylation from gene expression: top methods from the IMPROVER Species Translation Challenge. Bioinform. 31(4): 462-470 (2015) - [j34]Sahand Hormoz, Gyan Bhanot, Michael Biehl
, Erhan Bilal
, Pablo Meyer, Raquel Norel
, Kahn Rhrissorrakrai, Adel Dayarian:
Inter-species inference of gene set enrichment in lung epithelial cells from proteomic and large transcriptomic datasets. Bioinform. 31(4): 492-500 (2015) - [j33]Ioannis Giotis, Nynke Molders, Sander Land
, Michael Biehl
, Marcel F. Jonkman, Nicolai Petkov:
MED-NODE: A computer-assisted melanoma diagnosis system using non-dermoscopic images. Expert Syst. Appl. 42(19): 6578-6585 (2015) - [j32]Oltman Ottes de Wiljes, Ronald A. J. van Elburg
, Michael Biehl
, Fred Keijzer:
Modeling spontaneous activity across an excitable epithelium: Support for a coordination scenario of early neural evolution. Frontiers Comput. Neurosci. 9: 110 (2015) - [j31]Mandy Lange, Michael Biehl
, Thomas Villmann:
Non-Euclidean principal component analysis by Hebbian learning. Neurocomputing 147: 107-119 (2015) - [j30]Gert-Jan de Vries, Steffen C. Pauws, Michael Biehl
:
Insightful stress detection from physiology modalities using Learning Vector Quantization. Neurocomputing 151: 873-882 (2015) - [j29]Michael Biehl
, Alessandro Ghio, Frank-Michael Schleif
:
Developments in computational intelligence and machine learning. Neurocomputing 169: 185-186 (2015) - [j28]Gert-Jan de Vries, Paul Lemmens, Dirk Brokken, Steffen Pauws, Michael Biehl
:
Towards Emotion Classification Using Appraisal Modeling. Int. J. Synth. Emot. 6(1): 40-59 (2015) - [c51]Michael Biehl
, Deborah Mudali, Klaus Leonard Leenders, Jos B. T. M. Roerdink:
Classification of FDG-PET Brain Data by Generalized Matrix Relevance LVQ. BrainComp 2015: 131-141 - [c50]Gert-Jan de Vries, Steffen Pauws, Michael Biehl
:
Facial Expression Recognition Using Learning Vector Quantization. CAIP (2) 2015: 760-771 - [c49]Thomas Villmann, Marika Kaden, David Nebel, Michael Biehl
:
Learning Vector Quantization with Adaptive Cost-Based Outlier-Rejection. CAIP (2) 2015: 772-782 - [c48]Ernest Mwebaze, Gjalt Bearda, Michael Biehl, Dietlind Zühlke:
Combining dissimilarity measures for prototype-based classification. ESANN 2015 - [c47]Michael Biehl
, Barbara Hammer
, Frank-Michael Schleif
, Petra Schneider, Thomas Villmann:
Stationarity of Matrix Relevance LVQ. IJCNN 2015: 1-8 - [c46]Alexander Schulz
, Bassam Mokbel, Michael Biehl
, Barbara Hammer
:
Inferring Feature Relevances From Metric Learning. SSCI 2015: 1599-1606 - 2014
- [j27]Ioannis Giotis, Kerstin Bunte
, Nicolai Petkov, Michael Biehl
:
Erratum to: Adaptive Matrices and Filters for Color Texture Classification. J. Math. Imaging Vis. 48(1): 202 (2014) - [c45]Benoît Frénay
, Daniela Hofmann, Alexander Schulz
, Michael Biehl
, Barbara Hammer
:
Valid interpretation of feature relevance for linear data mappings. CIDM 2014: 149-156 - [c44]Herbert Teun Kruitbosch, Ioannis Giotis, Michael Biehl:
Segmented shape-symbolic time series representation. ESANN 2014 - [c43]Michael Biehl
:
Prototype-Based Classifiers and Their Application in the Life Sciences. WSOM 2014: 121 - 2013
- [j26]Enrique Alegre
, Michael Biehl
, Nicolai Petkov, Lidia Sánchez
:
Assessment of acrosome state in boar spermatozoa heads using n-contours descriptor and RLVQ. Comput. Methods Programs Biomed. 111(3): 525-536 (2013) - [j25]Ioannis Giotis, Kerstin Bunte
, Nicolai Petkov, Michael Biehl
:
Adaptive Matrices and Filters for Color Texture Classification. J. Math. Imaging Vis. 47(1-2): 79-92 (2013) - [c42]Michael Biehl
, Barbara Hammer
, Thomas Villmann:
Distance Measures for Prototype Based Classification. BrainComp 2013: 100-116 - [c41]Marc Strickert, Barbara Hammer
, Thomas Villmann, Michael Biehl
:
Regularization and improved interpretation of linear data mappings and adaptive distance measures. CIDM 2013: 10-17 - [c40]Mandy Lange, Michael Biehl, Thomas Villmann:
Non-Euclidean independent component analysis and Oja's learning. ESANN 2013 - 2012
- [j24]Markus B. Huber, Kerstin Bunte
, Mahesh B. Nagarajan, Michael Biehl
, Lawrence A. Ray, Axel Wismüller:
Texture feature ranking with relevance learning to classify interstitial lung disease patterns. Artif. Intell. Medicine 56(2): 91-97 (2012) - [j23]Kerstin Bunte
, Sven Haase, Michael Biehl
, Thomas Villmann:
Stochastic neighbor embedding (SNE) for dimension reduction and visualization using arbitrary divergences. Neurocomputing 90: 23-45 (2012) - [j22]Marika Kästner, Barbara Hammer
, Michael Biehl
, Thomas Villmann:
Functional relevance learning in generalized learning vector quantization. Neurocomputing 90: 85-95 (2012) - [j21]Michael Biehl
:
Admire LVQ - Adaptive Distance Measures in Relevance Learning Vector Quantization. Künstliche Intell. 26(4): 391-395 (2012) - [j20]Kerstin Bunte
, Michael Biehl
, Barbara Hammer
:
A General Framework for Dimensionality-Reducing Data Visualization Mapping. Neural Comput. 24(3): 771-804 (2012) - [j19]Kerstin Bunte
, Petra Schneider, Barbara Hammer
, Frank-Michael Schleif
, Thomas Villmann, Michael Biehl
:
Limited Rank Matrix Learning, discriminative dimension reduction and visualization. Neural Networks 26: 159-173 (2012) - [c39]Michael Biehl, Petra Schneider, David Smith, Han Stiekema, Angela Taylor, Beverly Hughes, Cedric Shackleton, Paul Stewart, Wiebke Arlt:
Matrix relevance LVQ in steroid metabolomics based classification of adrenal tumors. ESANN 2012 - [c38]Kerstin Bunte, Frank-Michael Schleif
, Michael Biehl:
Adaptive learning for complex-valued data. ESANN 2012 - [c37]Bassam Mokbel, Wouter Lueks, Andrej Gisbrecht, Michael Biehl, Barbara Hammer:
Visualizing the quality of dimensionality reduction. ESANN 2012 - [c36]Marika Kästner, David Nebel, Martin Riedel, Michael Biehl
, Thomas Villmann:
Differentiable Kernels in Generalized Matrix Learning Vector Quantization. ICMLA (1) 2012: 132-137 - [c35]Michael Biehl
, Kerstin Bunte
, Frank-Michael Schleif
, Petra Schneider, Thomas Villmann:
Large margin linear discriminative visualization by Matrix Relevance Learning. IJCNN 2012: 1-8 - [c34]Gabriele Peters, Kerstin Bunte
, Marc Strickert, Michael Biehl
, Thomas Villmann:
Visualization of processes in self-learning systems. PST 2012: 244-249 - [c33]Michael Biehl
, Marika Kästner, Mandy Lange, Thomas Villmann:
Non-Euclidean Principal Component Analysis and Oja's Learning Rule - Theoretical Aspects. WSOM 2012: 23-33 - 2011
- [j18]Kerstin Bunte
, Barbara Hammer
, Thomas Villmann, Michael Biehl
, Axel Wismüller:
Neighbor embedding XOM for dimension reduction and visualization. Neurocomputing 74(9): 1340-1350 (2011) - [j17]Ernest Mwebaze, Petra Schneider, Frank-Michael Schleif
, Jennifer R. Aduwo, John A. Quinn, Sven Haase, Thomas Villmann, Michael Biehl
:
Divergence-based classification in learning vector quantization. Neurocomputing 74(9): 1429-1435 (2011) - [j16]Kerstin Bunte
, Michael Biehl
, Marcel F. Jonkman, Nicolai Petkov:
Learning effective color features for content based image retrieval in dermatology. Pattern Recognit. 44(9): 1892-1902 (2011) - [c32]Kerstin Bunte
, Ioannis Giotis, Nicolai Petkov, Michael Biehl
:
Adaptive Matrices for Color Texture Classification. CAIP (2) 2011: 489-497 - [c31]Kerstin Bunte
, Michael Biehl
, Barbara Hammer
:
Dimensionality reduction mappings. CIDM 2011: 349-356 - [c30]Kerstin Bunte, Michael Biehl, Barbara Hammer:
Supervised dimension reduction mappings. ESANN 2011 - [c29]Marika Kästner, Barbara Hammer, Michael Biehl, Thomas Villmann:
Generalized functional relevance learning vector quantization. ESANN 2011 - [c28]Ernest Mwebaze, John A. Quinn, Michael Biehl:
Causal relevance learning for robust classification under interventions. ESANN 2011 - [c27]John A. Quinn, Joris M. Mooij, Tom Heskes, Michael Biehl:
Learning of causal relations. ESANN 2011 - [c26]Petra Schneider, Tina Geweniger, Frank-Michael Schleif
, Michael Biehl, Thomas Villmann:
Multivariate class labeling in Robust Soft LVQ. ESANN 2011 - [c25]Markus B. Huber, Kerstin Bunte
, Mahesh B. Nagarajan, Michael Biehl
, Lawrence A. Ray, Axel Wismüller:
Texture feature selection with relevance learning to classify interstitial lung disease patterns. Computer-Aided Diagnosis 2011: 796318 - [c24]Barbara Hammer
, Michael Biehl
, Kerstin Bunte
, Bassam Mokbel:
A General Framework for Dimensionality Reduction for Large Data Sets. WSOM 2011: 277-287 - [i9]Wouter Lueks, Bassam Mokbel, Michael Biehl, Barbara Hammer:
How to Evaluate Dimensionality Reduction? - Improving the Co-ranking Matrix. CoRR abs/1110.3917 (2011) - [i8]Michael Biehl
, Barbara Hammer, Erzsébet Merényi, Alessandro Sperduti, Thomas Villmann:
Learning in the context of very high dimensional data (Dagstuhl Seminar 11341). Dagstuhl Reports 1(8): 67-95 (2011) - 2010
- [j15]Kerstin Bunte
, Barbara Hammer
, Axel Wismüller, Michael Biehl
:
Adaptive local dissimilarity measures for discriminative dimension reduction of labeled data. Neurocomputing 73(7-9): 1074-1092 (2010) - [j14]Petra Schneider, Michael Biehl
, Barbara Hammer
:
Hyperparameter learning in probabilistic prototype-based models. Neurocomputing 73(7-9): 1117-1124 (2010) - [j13]Aree Witoelar, Anarta Ghosh, J. J. G. de Vries, Barbara Hammer
, Michael Biehl
:
Window-Based Example Selection in Learning Vector Quantization. Neural Comput. 22(11): 2924-2961 (2010) - [j12]Petra Schneider, Kerstin Bunte
, Han Stiekema, Barbara Hammer
, Thomas Villmann, Michael Biehl
:
Regularization in matrix relevance learning. IEEE Trans. Neural Networks 21(5): 831-840 (2010) - [c23]Ot de Wiljes, Ronald A. J. van Elburg, Michael Biehl, Fred Keijzer:
Early Nervous Systems - Theoretical Background and a Preliminary Model of Neuronal Processes. ALIFE 2010: 239-240 - [c22]Thomas Villmann, Sven Haase, Frank-Michael Schleif
, Barbara Hammer
, Michael Biehl
:
The Mathematics of Divergence Based Online Learning in Vector Quantization. ANNPR 2010: 108-119 - [c21]Kerstin Bunte, Barbara Hammer, Thomas Villmann, Michael Biehl, Axel Wismüller:
Exploratory Observation Machine (XOM) with Kullback-Leibler Divergence for Dimensionality Reduction and Visualization. ESANN 2010 - [c20]Ernest Mwebaze, Petra Schneider, Frank-Michael Schleif
, Sven Haase, Thomas Villmann, Michael Biehl:
Divergence based Learning Vector Quantization. ESANN 2010 - [c19]Frank-Michael Schleif
, Thomas Villmann, Barbara Hammer
, Petra Schneider, Michael Biehl
:
Generalized Derivative Based Kernelized Learning Vector Quantization. IDEAL 2010: 21-28 - [i7]Barbara Hammer, Kerstin Bunte, Michael Biehl:
Some steps towards a general principle for dimensionality reduction mappings. Learning paradigms in dynamic environments 2010
2000 – 2009
- 2009
- [j11]Frank-Michael Schleif
, Michael Biehl
, Alfredo Vellido:
Advances in machine learning and computational intelligence. Neurocomputing 72(7-9): 1377-1378 (2009) - [j10]Aree Witoelar, Michael Biehl
:
Phase transitions in vector quantization and neural gas. Neurocomputing 72(7-9): 1390-1397 (2009) - [j9]Petra Schneider, Michael Biehl
, Barbara Hammer
:
Distance Learning in Discriminative Vector Quantization. Neural Comput. 21(10): 2942-2969 (2009) - [j8]Petra Schneider, Michael Biehl
, Barbara Hammer
:
Adaptive Relevance Matrices in Learning Vector Quantization. Neural Comput. 21(12): 3532-3561 (2009) - [c18]Kerstin Bunte
, Barbara Hammer
, Michael Biehl
:
Nonlinear Dimension Reduction and Visualization of Labeled Data. CAIP 2009: 1162-1170 - [c17]Michael Biehl
, Nestor Caticha, Peter Riegler
:
Statistical Mechanics of On-line Learning. Similarity-Based Clustering 2009: 1-22 - [c16]Thomas Villmann, Barbara Hammer
, Michael Biehl
:
Some Theoretical Aspects of the Neural Gas Vector Quantizer. Similarity-Based Clustering 2009: 23-34 - [c15]Kerstin Bunte, Michael Biehl, Nicolai Petkov, Marcel F. Jonkman:
Adaptive Metrics for Content Based Image Retrieval in Dermatology. ESANN 2009 - [c14]Kerstin Bunte, Barbara Hammer, Petra Schneider, Michael Biehl:
Nonlinear Discriminative Data Visualization. ESANN 2009 - [c13]Petra Schneider, Michael Biehl, Barbara Hammer:
Hyperparameter Learning in Robust Soft LVQ. ESANN 2009 - [c12]Aree Witoelar, Michael Biehl, Barbara Hammer:
Equilibrium properties of off-line LVQ. ESANN 2009 - [c11]Marc Strickert, Jens Keilwagen
, Frank-Michael Schleif
, Thomas Villmann, Michael Biehl
:
Matrix Metric Adaptation Linear Discriminant Analysis of Biomedical Data. IWANN (1) 2009: 933-940 - [p1]Michael Biehl
, Barbara Hammer
, Petra Schneider, Thomas Villmann:
Metric Learning for Prototype-Based Classification. Innovations in Neural Information Paradigms and Applications 2009: 183-199 - [e3]Michael Biehl
, Barbara Hammer
, Michel Verleysen, Thomas Villmann:
Similarity-Based Clustering, Recent Developments and Biomedical Applications [outcome of a Dagstuhl Seminar]. Lecture Notes in Computer Science 5400, Springer 2009, ISBN 978-3-642-01804-6 [contents] - [e2]Michael Biehl, Barbara Hammer, Sepp Hochreiter, Stefan C. Kremer, Thomas Villmann:
Similarity-based learning on structures, 15.02. - 20.02.2009. Dagstuhl Seminar Proceedings 09081, Schloss Dagstuhl - Leibniz-Zentrum für Informatik, Germany 2009 [contents] - [i6]Michael Biehl, Barbara Hammer, Sepp Hochreiter, Stefan C. Kremer, Thomas Villmann:
09081 Abstracts Collection - Similarity-based learning on structures. Similarity-based learning on structures 2009 - [i5]Michael Biehl, Barbara Hammer, Sepp Hochreiter, Stefan C. Kremer, Thomas Villmann:
09081 Summary - Similarity-based learning on structures. Similarity-based learning on structures 2009 - [i4]Gert-Jan de Vries, Michael Biehl:
Analysis of Robust Soft Learning Vector Quantization and an application to Facial Expression Recognition. Similarity-based learning on structures 2009 - 2008
- [j7]Enrique Alegre
, Michael Biehl
, Nicolai Petkov, Lidia Sánchez
:
Automatic classification of the acrosome status of boar spermatozoa using digital image processing and LVQ. Comput. Biol. Medicine 38(4): 461-468 (2008) - [j6]Fabrice Rossi
, Michael Biehl
, Cecilio Angulo Bahón
:
Progress in modeling, theory, and application of computational intelligence. Neurocomputing 71(7-9): 1117-1119 (2008) - [j5]Aree Witoelar, Michael Biehl
, Anarta Ghosh, Barbara Hammer
:
Learning dynamics and robustness of vector quantization and neural gas. Neurocomputing 71(7-9): 1210-1219 (2008) - [c10]Marc Strickert, Petra Schneider, Jens Keilwagen
, Thomas Villmann, Michael Biehl
, Barbara Hammer
:
Discriminatory Data Mapping by Matrix-Based Supervised Learning Metrics. ANNPR 2008: 78-89 - [c9]Aree Witoelar, Anarta Ghosh, Michael Biehl:
Phase transitions in Vector Quantization. ESANN 2008: 221-226 - [c8]Petra Schneider, Frank-Michael Schleif
, Thomas Villmann, Michael Biehl:
Generalized matrix learning vector quantizer for the analysis of spectral data. ESANN 2008: 451-456 - 2007
- [j4]Michael Biehl
, Erzsébet Merényi, Fabrice Rossi
:
Advances in computational intelligence and learning. Neurocomputing 70(7-9): 1117-1119 (2007) - [j3]Michael Biehl, Anarta Ghosh, Barbara Hammer:
Dynamics and Generalization Ability of LVQ Algorithms. J. Mach. Learn. Res. 8: 323-360 (2007) - [c7]Petra Schneider, Michael Biehl, Barbara Hammer:
Relevance matrices in LVQ. ESANN 2007: 37-42 - [c6]Aree Witoelar, Michael Biehl, Anarta Ghosh, Barbara Hammer:
On the dynamics of Vector Quantization and Neural Gas. ESANN 2007: 127-132 - [c5]Michael Biehl
, Rainer Breitling
, Yang Li
:
Analysis of Tiling Microarray Data by Learning Vector Quantization and Relevance Learning. IDEAL 2007: 880-889 - [e1]Michael Biehl, Barbara Hammer, Michel Verleysen, Thomas Villmann:
Similarity-based Clustering and its Application to Medicine and Biology, 25.03. - 30.03.2007. Dagstuhl Seminar Proceedings 07131, Internationales Begegnungs- und Forschungszentrum fuer Informatik (IBFI), Schloss Dagstuhl, Germany 2007 [contents] - [i3]Michael Biehl, Barbara Hammer, Michel Verleysen, Thomas Villmann:
07131 Summary -- Similarity-based Clustering and its Application to Medicine and Biology. Similarity-based Clustering and its Application to Medicine and Biology 2007 - [i2]Michael Biehl, Barbara Hammer, Michel Verleysen, Thomas Villmann:
07131 Abstracts Collection -- Similarity-based Clustering and its Application to Medicine and Biology. Similarity-based Clustering and its Application to Medicine and Biology 2007 - [i1]Aree Witoelar, Michael Biehl, Barbara Hammer:
Learning Vector Quantization: generalization ability and dynamics of competing prototypes. Similarity-based Clustering and its Application to Medicine and Biology 2007 - 2006
- [j2]Michael Biehl
, Anarta Ghosh, Barbara Hammer
:
Learning vector quantization: The dynamics of winner-takes-all algorithms. Neurocomputing 69(7-9): 660-670 (2006) - [j1]Anarta Ghosh, Michael Biehl
, Barbara Hammer
:
Performance analysis of LVQ algorithms: A statistical physics approach. Neural Networks 19(6-7): 817-829 (2006) - [c4]Nikolai Petkov, Enrique Alegre, Michael Biehl, Lidia Sánchez:
LVQ acrosome integrity assessment of boar sperm cells. CompIMAGE 2006: 337-342 - [c3]Michael Biehl, Piter Pasma, Marten Pijl, Lidia Sánchez, Nicolai Petkov:
Classification of Boar Sperm Head Images using Learning Vector Quantization. ESANN 2006: 545-550 - 2005
- [c2]Michael Biehl, Anarta Ghosh, Barbara Hammer:
The dynamics of Learning Vector Quantization. ESANN 2005: 13-18 - 2002
- [c1]Christoph Bunzmann, Michael Biehl, Robert Urbanczik:
Supervised learning in committee machines by PCA. ESANN 2002: 125-130
Coauthor Index
aka: Nikolai Petkov
aka: J. J. G. de Vries

manage site settings
To protect your privacy, all features that rely on external API calls from your browser are turned off by default. You need to opt-in for them to become active. All settings here will be stored as cookies with your web browser. For more information see our F.A.Q.
Unpaywalled article links
Add open access links from to the list of external document links (if available).
Privacy notice: By enabling the option above, your browser will contact the API of unpaywall.org to load hyperlinks to open access articles. Although we do not have any reason to believe that your call will be tracked, we do not have any control over how the remote server uses your data. So please proceed with care and consider checking the Unpaywall privacy policy.
Archived links via Wayback Machine
For web page which are no longer available, try to retrieve content from the of the Internet Archive (if available).
Privacy notice: By enabling the option above, your browser will contact the API of archive.org to check for archived content of web pages that are no longer available. Although we do not have any reason to believe that your call will be tracked, we do not have any control over how the remote server uses your data. So please proceed with care and consider checking the Internet Archive privacy policy.
Reference lists
Add a list of references from ,
, and
to record detail pages.
load references from crossref.org and opencitations.net
Privacy notice: By enabling the option above, your browser will contact the APIs of crossref.org, opencitations.net, and semanticscholar.org to load article reference information. Although we do not have any reason to believe that your call will be tracked, we do not have any control over how the remote server uses your data. So please proceed with care and consider checking the Crossref privacy policy and the OpenCitations privacy policy, as well as the AI2 Privacy Policy covering Semantic Scholar.
Citation data
Add a list of citing articles from and
to record detail pages.
load citations from opencitations.net
Privacy notice: By enabling the option above, your browser will contact the API of opencitations.net and semanticscholar.org to load citation information. Although we do not have any reason to believe that your call will be tracked, we do not have any control over how the remote server uses your data. So please proceed with care and consider checking the OpenCitations privacy policy as well as the AI2 Privacy Policy covering Semantic Scholar.
OpenAlex data
Load additional information about publications from .
Privacy notice: By enabling the option above, your browser will contact the API of openalex.org to load additional information. Although we do not have any reason to believe that your call will be tracked, we do not have any control over how the remote server uses your data. So please proceed with care and consider checking the information given by OpenAlex.
last updated on 2025-05-19 22:16 CEST by the dblp team
all metadata released as open data under CC0 1.0 license
see also: Terms of Use | Privacy Policy | Imprint