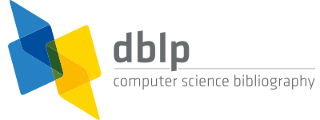


default search action
Matus Telgarsky
Person information
- affiliation: University of Illinois at Urbana-Champaign, Department of Computer Science, IL, USA
- affiliation: Carnegie Mellon University, Machine Learning Department, Pittsburgh, PA, USA
Refine list

refinements active!
zoomed in on ?? of ?? records
view refined list in
export refined list as
Books and Theses
- 2013
- [b1]Matus Telgarsky:
Duality and Data Dependence in Boosting /. University of California, San Diego, USA, 2013
Journal Articles
- 2014
- [j2]Animashree Anandkumar, Rong Ge, Daniel J. Hsu, Sham M. Kakade, Matus Telgarsky:
Tensor decompositions for learning latent variable models. J. Mach. Learn. Res. 15(1): 2773-2832 (2014) - 2012
- [j1]Matus Telgarsky:
A Primal-Dual Convergence Analysis of Boosting. J. Mach. Learn. Res. 13: 561-606 (2012)
Conference and Workshop Papers
- 2024
- [c36]Ali Ebrahimpour Boroojeny, Matus Telgarsky, Hari Sundaram:
Spectrum Extraction and Clipping for Implicitly Linear Layers. AISTATS 2024: 2971-2979 - [c35]Jingfeng Wu, Peter L. Bartlett, Matus Telgarsky, Bin Yu:
Large Stepsize Gradient Descent for Logistic Loss: Non-Monotonicity of the Loss Improves Optimization Efficiency. COLT 2024: 5019-5073 - [c34]Clayton Sanford, Daniel Hsu, Matus Telgarsky:
Transformers, parallel computation, and logarithmic depth. ICML 2024 - 2023
- [c33]Justin D. Li, Matus Telgarsky:
On Achieving Optimal Adversarial Test Error. ICLR 2023 - [c32]Matus Telgarsky:
Feature selection and low test error in shallow low-rotation ReLU networks. ICLR 2023 - [c31]Clayton Sanford, Daniel J. Hsu, Matus Telgarsky:
Representational Strengths and Limitations of Transformers. NeurIPS 2023 - 2022
- [c30]Matus Telgarsky:
Stochastic linear optimization never overfits with quadratically-bounded losses on general data. COLT 2022: 5453-5488 - [c29]Yuzheng Hu, Ziwei Ji, Matus Telgarsky:
Actor-critic is implicitly biased towards high entropy optimal policies. ICLR 2022 - 2021
- [c28]Ziwei Ji, Matus Telgarsky:
Characterizing the implicit bias via a primal-dual analysis. ALT 2021: 772-804 - [c27]Daniel Hsu, Ziwei Ji, Matus Telgarsky, Lan Wang:
Generalization bounds via distillation. ICLR 2021 - [c26]Ziwei Ji, Nathan Srebro, Matus Telgarsky:
Fast margin maximization via dual acceleration. ICML 2021: 4860-4869 - [c25]Ziwei Ji, Justin D. Li, Matus Telgarsky:
Early-stopped neural networks are consistent. NeurIPS 2021: 1805-1817 - 2020
- [c24]Ziwei Ji, Miroslav Dudík, Robert E. Schapire, Matus Telgarsky:
Gradient descent follows the regularization path for general losses. COLT 2020: 2109-2136 - [c23]Ziwei Ji, Matus Telgarsky:
Polylogarithmic width suffices for gradient descent to achieve arbitrarily small test error with shallow ReLU networks. ICLR 2020 - [c22]Ziwei Ji, Matus Telgarsky, Ruicheng Xian:
Neural tangent kernels, transportation mappings, and universal approximation. ICLR 2020 - [c21]Ziwei Ji, Matus Telgarsky:
Directional convergence and alignment in deep learning. NeurIPS 2020 - 2019
- [c20]Ziwei Ji, Matus Telgarsky:
The implicit bias of gradient descent on nonseparable data. COLT 2019: 1772-1798 - [c19]Ziwei Ji, Matus Telgarsky:
Gradient descent aligns the layers of deep linear networks. ICLR (Poster) 2019 - [c18]Yucheng Chen, Matus Telgarsky, Chao Zhang, Bolton Bailey, Daniel Hsu, Jian Peng:
A Gradual, Semi-Discrete Approach to Generative Network Training via Explicit Wasserstein Minimization. ICML 2019: 1071-1080 - 2018
- [c17]Bolton Bailey, Matus Telgarsky:
Size-Noise Tradeoffs in Generative Networks. NeurIPS 2018: 6490-6500 - [c16]Ziwei Ji, Ruta Mehta, Matus Telgarsky:
Social Welfare and Profit Maximization from Revealed Preferences. WINE 2018: 264-281 - 2017
- [c15]Maxim Raginsky, Alexander Rakhlin, Matus Telgarsky:
Non-convex learning via Stochastic Gradient Langevin Dynamics: a nonasymptotic analysis. COLT 2017: 1674-1703 - [c14]Matus Telgarsky:
Neural Networks and Rational Functions. ICML 2017: 3387-3393 - [c13]Peter L. Bartlett, Dylan J. Foster, Matus Telgarsky:
Spectrally-normalized margin bounds for neural networks. NIPS 2017: 6240-6249 - 2016
- [c12]Matus Telgarsky:
benefits of depth in neural networks. COLT 2016: 1517-1539 - [c11]Jacob D. Abernethy, Sébastien Lahaie, Matus Telgarsky:
Rate of Price Discovery in Iterative Combinatorial Auctions. EC 2016: 809 - 2015
- [c10]Anima Anandkumar, Rong Ge, Daniel J. Hsu, Sham M. Kakade, Matus Telgarsky:
Tensor Decompositions for Learning Latent Variable Models (A Survey for ALT). ALT 2015: 19-38 - [c9]Matus Telgarsky, Miroslav Dudík:
Convex Risk Minimization and Conditional Probability Estimation. COLT 2015: 1629-1682 - 2014
- [c8]Alekh Agarwal, Alina Beygelzimer, Daniel J. Hsu, John Langford, Matus Telgarsky:
Scalable Non-linear Learning with Adaptive Polynomial Expansions. NIPS 2014: 2051-2059 - 2013
- [c7]Matus Telgarsky:
Boosting with the Logistic Loss is Consistent. COLT 2013: 911-965 - [c6]Matus Telgarsky:
Margins, Shrinkage, and Boosting. ICML (2) 2013: 307-315 - [c5]Matus Telgarsky, Sanjoy Dasgupta:
Moment-based Uniform Deviation Bounds for k-means and Friends. NIPS 2013: 2940-2948 - 2012
- [c4]Matus Telgarsky, Sanjoy Dasgupta:
Agglomerative Bregman Clustering. ICML 2012 - 2011
- [c3]Matus Telgarsky:
The Fast Convergence of Boosting. NIPS 2011: 1593-1601 - 2010
- [c2]Matus Telgarsky, Andrea Vattani:
Hartigan's Method: k-means Clustering without Voronoi. AISTATS 2010: 820-827 - 2007
- [c1]Matus Telgarsky, John D. Lafferty:
Signal Decomposition using Multiscale Admixture Models. ICASSP (2) 2007: 449-452
Informal and Other Publications
- 2024
- [i40]Clayton Sanford, Daniel Hsu, Matus Telgarsky:
Transformers, parallel computation, and logarithmic depth. CoRR abs/2402.09268 (2024) - [i39]Jingfeng Wu, Peter L. Bartlett, Matus Telgarsky, Bin Yu:
Large Stepsize Gradient Descent for Logistic Loss: Non-Monotonicity of the Loss Improves Optimization Efficiency. CoRR abs/2402.15926 (2024) - [i38]Ali Ebrahimpour Boroojeny, Matus Telgarsky, Hari Sundaram:
Spectrum Extraction and Clipping for Implicitly Linear Layers. CoRR abs/2402.16017 (2024) - [i37]Clayton Sanford, Daniel Hsu, Matus Telgarsky:
One-layer transformers fail to solve the induction heads task. CoRR abs/2408.14332 (2024) - 2023
- [i36]Clayton Sanford, Daniel Hsu, Matus Telgarsky:
Representational Strengths and Limitations of Transformers. CoRR abs/2306.02896 (2023) - [i35]Justin D. Li, Matus Telgarsky:
On Achieving Optimal Adversarial Test Error. CoRR abs/2306.07544 (2023) - 2022
- [i34]Matus Telgarsky:
Stochastic linear optimization never overfits with quadratically-bounded losses on general data. CoRR abs/2202.06915 (2022) - [i33]Miroslav Dudík, Ziwei Ji, Robert E. Schapire, Matus Telgarsky:
Convex Analysis at Infinity: An Introduction to Astral Space. CoRR abs/2205.03260 (2022) - [i32]Matus Telgarsky:
Feature selection with gradient descent on two-layer networks in low-rotation regimes. CoRR abs/2208.02789 (2022) - 2021
- [i31]Daniel Hsu, Ziwei Ji, Matus Telgarsky, Lan Wang:
Generalization bounds via distillation. CoRR abs/2104.05641 (2021) - [i30]Ziwei Ji, Justin D. Li, Matus Telgarsky:
Early-stopped neural networks are consistent. CoRR abs/2106.05932 (2021) - [i29]Ziwei Ji, Nathan Srebro, Matus Telgarsky:
Fast Margin Maximization via Dual Acceleration. CoRR abs/2107.00595 (2021) - [i28]Yuzheng Hu, Ziwei Ji, Matus Telgarsky:
Actor-critic is implicitly biased towards high entropy optimal policies. CoRR abs/2110.11280 (2021) - 2020
- [i27]Ziwei Ji, Matus Telgarsky:
Directional convergence and alignment in deep learning. CoRR abs/2006.06657 (2020) - [i26]Ziwei Ji, Miroslav Dudík, Robert E. Schapire, Matus Telgarsky:
Gradient descent follows the regularization path for general losses. CoRR abs/2006.11226 (2020) - 2019
- [i25]Yucheng Chen, Matus Telgarsky, Chao Zhang, Bolton Bailey, Daniel Hsu, Jian Peng:
A gradual, semi-discrete approach to generative network training via explicit Wasserstein minimization. CoRR abs/1906.03471 (2019) - [i24]Ziwei Ji, Matus Telgarsky:
A refined primal-dual analysis of the implicit bias. CoRR abs/1906.04540 (2019) - [i23]Ziwei Ji, Matus Telgarsky:
Polylogarithmic width suffices for gradient descent to achieve arbitrarily small test error with shallow ReLU networks. CoRR abs/1909.12292 (2019) - [i22]Ziwei Ji, Matus Telgarsky, Ruicheng Xian:
Neural tangent kernels, transportation mappings, and universal approximation. CoRR abs/1910.06956 (2019) - 2018
- [i21]Ziwei Ji, Matus Telgarsky:
Risk and parameter convergence of logistic regression. CoRR abs/1803.07300 (2018) - [i20]Ziwei Ji, Matus Telgarsky:
Gradient descent aligns the layers of deep linear networks. CoRR abs/1810.02032 (2018) - [i19]Bolton Bailey, Matus Telgarsky:
Size-Noise Tradeoffs in Generative Networks. CoRR abs/1810.11158 (2018) - 2017
- [i18]Maxim Raginsky, Alexander Rakhlin, Matus Telgarsky:
Non-convex learning via Stochastic Gradient Langevin Dynamics: a nonasymptotic analysis. CoRR abs/1702.03849 (2017) - [i17]Matus Telgarsky:
Neural networks and rational functions. CoRR abs/1706.03301 (2017) - [i16]Peter L. Bartlett, Dylan J. Foster, Matus Telgarsky:
Spectrally-normalized margin bounds for neural networks. CoRR abs/1706.08498 (2017) - [i15]Ziwei Ji, Ruta Mehta, Matus Telgarsky:
Social Welfare and Profit Maximization from Revealed Preferences. CoRR abs/1711.02211 (2017) - 2016
- [i14]Matus Telgarsky:
Benefits of depth in neural networks. CoRR abs/1602.04485 (2016) - [i13]Daniel J. Hsu, Matus Telgarsky:
Greedy bi-criteria approximations for k-medians and k-means. CoRR abs/1607.06203 (2016) - 2015
- [i12]Matus Telgarsky, Miroslav Dudík, Robert E. Schapire:
Convex Risk Minimization and Conditional Probability Estimation. CoRR abs/1506.04513 (2015) - [i11]Matus Telgarsky:
Representation Benefits of Deep Feedforward Networks. CoRR abs/1509.08101 (2015) - [i10]Jacob D. Abernethy, Sébastien Lahaie, Matus Telgarsky:
Rate of Price Discovery in Iterative Combinatorial Auctions. CoRR abs/1511.06017 (2015) - 2014
- [i9]Alekh Agarwal, Alina Beygelzimer, Daniel J. Hsu, John Langford, Matus Telgarsky:
Scalable Nonlinear Learning with Adaptive Polynomial Expansions. CoRR abs/1410.0440 (2014) - 2013
- [i8]Matus Telgarsky:
Dirichlet draws are sparse with high probability. CoRR abs/1301.4917 (2013) - [i7]Matus Telgarsky:
Margins, Shrinkage, and Boosting. CoRR abs/1303.4172 (2013) - [i6]Matus Telgarsky:
Boosting with the Logistic Loss is Consistent. CoRR abs/1305.2648 (2013) - [i5]Matus Telgarsky, Sanjoy Dasgupta:
Moment-based Uniform Deviation Bounds for $k$-means and Friends. CoRR abs/1311.1903 (2013) - 2012
- [i4]Matus Telgarsky:
Statistical Consistency of Finite-dimensional Unregularized Linear Classification. CoRR abs/1206.3072 (2012) - [i3]Anima Anandkumar, Rong Ge, Daniel J. Hsu, Sham M. Kakade, Matus Telgarsky:
Tensor decompositions for learning latent variable models. CoRR abs/1210.7559 (2012) - 2011
- [i2]Matus Telgarsky:
The Convergence Rate of AdaBoost and Friends. CoRR abs/1101.4752 (2011) - [i1]Matus Telgarsky:
Blackwell Approachability and Minimax Theory. CoRR abs/1110.1514 (2011)
Coauthor Index
aka: Daniel J. Hsu

manage site settings
To protect your privacy, all features that rely on external API calls from your browser are turned off by default. You need to opt-in for them to become active. All settings here will be stored as cookies with your web browser. For more information see our F.A.Q.
Unpaywalled article links
Add open access links from to the list of external document links (if available).
Privacy notice: By enabling the option above, your browser will contact the API of unpaywall.org to load hyperlinks to open access articles. Although we do not have any reason to believe that your call will be tracked, we do not have any control over how the remote server uses your data. So please proceed with care and consider checking the Unpaywall privacy policy.
Archived links via Wayback Machine
For web page which are no longer available, try to retrieve content from the of the Internet Archive (if available).
Privacy notice: By enabling the option above, your browser will contact the API of archive.org to check for archived content of web pages that are no longer available. Although we do not have any reason to believe that your call will be tracked, we do not have any control over how the remote server uses your data. So please proceed with care and consider checking the Internet Archive privacy policy.
Reference lists
Add a list of references from ,
, and
to record detail pages.
load references from crossref.org and opencitations.net
Privacy notice: By enabling the option above, your browser will contact the APIs of crossref.org, opencitations.net, and semanticscholar.org to load article reference information. Although we do not have any reason to believe that your call will be tracked, we do not have any control over how the remote server uses your data. So please proceed with care and consider checking the Crossref privacy policy and the OpenCitations privacy policy, as well as the AI2 Privacy Policy covering Semantic Scholar.
Citation data
Add a list of citing articles from and
to record detail pages.
load citations from opencitations.net
Privacy notice: By enabling the option above, your browser will contact the API of opencitations.net and semanticscholar.org to load citation information. Although we do not have any reason to believe that your call will be tracked, we do not have any control over how the remote server uses your data. So please proceed with care and consider checking the OpenCitations privacy policy as well as the AI2 Privacy Policy covering Semantic Scholar.
OpenAlex data
Load additional information about publications from .
Privacy notice: By enabling the option above, your browser will contact the API of openalex.org to load additional information. Although we do not have any reason to believe that your call will be tracked, we do not have any control over how the remote server uses your data. So please proceed with care and consider checking the information given by OpenAlex.
last updated on 2024-09-30 00:59 CEST by the dblp team
all metadata released as open data under CC0 1.0 license
see also: Terms of Use | Privacy Policy | Imprint