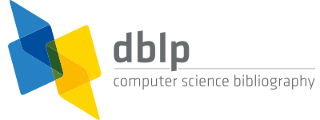


default search action
Willem Waegeman
Person information
Refine list

refinements active!
zoomed in on ?? of ?? records
view refined list in
export refined list as
2020 – today
- 2024
- [j36]Dimitrios Iliadis
, Marcel Wever
, Bernard De Baets
, Willem Waegeman
:
Hyperparameter optimization of two-branch neural networks in multi-target prediction. Appl. Soft Comput. 165: 111957 (2024) - [j35]Dimitrios Iliadis, Bernard De Baets, Tapio Pahikkala
, Willem Waegeman:
A comparison of embedding aggregation strategies in drug-target interaction prediction. BMC Bioinform. 25(1): 59 (2024) - [c28]Mira Jürgens, Nis Meinert, Viktor Bengs, Eyke Hüllermeier, Willem Waegeman:
Is Epistemic Uncertainty Faithfully Represented by Evidential Deep Learning Methods? ICML 2024 - [i22]Mira Jürgens, Nis Meinert, Viktor Bengs, Eyke Hüllermeier, Willem Waegeman:
Is Epistemic Uncertainty Faithfully Represented by Evidential Deep Learning Methods? CoRR abs/2402.09056 (2024) - [i21]Nina Schmid, David Fernandes del Pozo, Willem Waegeman, Jan Hasenauer:
Assessment of Uncertainty Quantification in Universal Differential Equations. CoRR abs/2406.08853 (2024) - 2023
- [j34]Nicolas Dewolf
, Bernard De Baets, Willem Waegeman:
Valid prediction intervals for regression problems. Artif. Intell. Rev. 56(1): 577-613 (2023) - [j33]Dimitrios Iliadis
, Bernard De Baets, Willem Waegeman:
DeepMTP: A Python-based deep learning framework for multi-target prediction. SoftwareX 23: 101516 (2023) - [c27]Thomas Mortier
, Viktor Bengs, Eyke Hüllermeier, Stijn Luca, Willem Waegeman:
On the Calibration of Probabilistic Classifier Sets. AISTATS 2023: 8857-8870 - [c26]Viktor Bengs, Eyke Hüllermeier, Willem Waegeman:
On Second-Order Scoring Rules for Epistemic Uncertainty Quantification. ICML 2023: 2078-2091 - [i20]Viktor Bengs, Eyke Hüllermeier, Willem Waegeman:
On Second-Order Scoring Rules for Epistemic Uncertainty Quantification. CoRR abs/2301.12736 (2023) - [i19]Nicolas Dewolf, Bernard De Baets, Willem Waegeman:
Heteroskedastic conformal regression. CoRR abs/2309.08313 (2023) - 2022
- [j32]Gaetan De Waele
, Jim Clauwaert
, Gerben Menschaert, Willem Waegeman
:
CpG Transformer for imputation of single-cell methylomes. Bioinform. 38(3): 597-603 (2022) - [j31]Dimitrios Iliadis
, Bernard De Baets, Willem Waegeman:
Multi-target prediction for dummies using two-branch neural networks. Mach. Learn. 111(2): 651-684 (2022) - [j30]Jim Clauwaert
, Willem Waegeman
:
Novel Transformer Networks for Improved Sequence Labeling in genomics. IEEE ACM Trans. Comput. Biol. Bioinform. 19(1): 97-106 (2022) - [c25]Viktor Bengs, Eyke Hüllermeier, Willem Waegeman:
Pitfalls of Epistemic Uncertainty Quantification through Loss Minimisation. NeurIPS 2022 - [c24]Thomas Mortier
, Eyke Hüllermeier, Krzysztof Dembczynski, Willem Waegeman:
Set-valued prediction in hierarchical classification with constrained representation complexity. UAI 2022: 1392-1401 - [i18]Viktor Bengs, Eyke Hüllermeier, Willem Waegeman:
On the Difficulty of Epistemic Uncertainty Quantification in Machine Learning: The Case of Direct Uncertainty Estimation through Loss Minimisation. CoRR abs/2203.06102 (2022) - [i17]Thomas Mortier, Eyke Hüllermeier, Krzysztof Dembczynski, Willem Waegeman:
Set-valued prediction in hierarchical classification with constrained representation complexity. CoRR abs/2203.06676 (2022) - [i16]Thomas Mortier, Viktor Bengs, Eyke Hüllermeier, Stijn Luca, Willem Waegeman:
On Calibration of Ensemble-Based Credal Predictors. CoRR abs/2205.10082 (2022) - [i15]Dimitrios Iliadis, Marcel Wever, Bernard De Baets, Willem Waegeman:
Hyperparameter optimization in deep multi-target prediction. CoRR abs/2211.04362 (2022) - 2021
- [j29]Jim Clauwaert
, Gerben Menschaert, Willem Waegeman:
Explainability in transformer models for functional genomics. Briefings Bioinform. 22(5) (2021) - [j28]Thomas Mortier
, Marek Wydmuch
, Krzysztof Dembczynski
, Eyke Hüllermeier, Willem Waegeman:
Efficient set-valued prediction in multi-class classification. Data Min. Knowl. Discov. 35(4): 1435-1469 (2021) - [j27]Eyke Hüllermeier
, Willem Waegeman:
Aleatoric and epistemic uncertainty in machine learning: an introduction to concepts and methods. Mach. Learn. 110(3): 457-506 (2021) - [i14]Dimitrios Iliadis, Bernard De Baets, Willem Waegeman:
Automated problem setting selection in multi-target prediction with AutoMTP. CoRR abs/2104.09967 (2021) - [i13]Nicolas Dewolf, Bernard De Baets, Willem Waegeman:
Well-calibrated prediction intervals for regression problems. CoRR abs/2107.00363 (2021) - 2020
- [j26]Michiel Stock, Tapio Pahikkala
, Antti Airola
, Willem Waegeman, Bernard De Baets:
Algebraic shortcuts for leave-one-out cross-validation in supervised network inference. Briefings Bioinform. 21(1): 262-271 (2020)
2010 – 2019
- 2019
- [j25]Mieke Deschepper
, Kristof Eeckloo, Dirk Vogelaers, Willem Waegeman:
A hospital wide predictive model for unplanned readmission using hierarchical ICD data. Comput. Methods Programs Biomed. 173: 177-183 (2019) - [j24]Willem Waegeman
, Krzysztof Dembczynski
, Eyke Hüllermeier:
Multi-target prediction: a unifying view on problems and methods. Data Min. Knowl. Discov. 33(2): 293-324 (2019) - [c23]Thomas Mortier, Marek Wydmuch, Krzysztof Dembczynski, Eyke Hüllermeier, Willem Waegeman:
Set-Valued Prediction in Multi-Class Classification. BNAIC/BENELEARN 2019 - [c22]Christina Papagiannopoulou, René Parchen, Willem Waegeman:
Investigating Time Series Classification Techniques for Rapid Pathogen Identification with Single-Cell MALDI-TOF Mass Spectrum Data. BNAIC/BENELEARN 2019 - [c21]Christina Papagiannopoulou, René Parchen, Willem Waegeman:
Investigating Time Series Classification Techniques for Rapid Pathogen Identification with Single-Cell MALDI-TOF Mass Spectrum Data. ECML/PKDD (3) 2019: 416-431 - [i12]Thomas Mortier, Marek Wydmuch, Eyke Hüllermeier, Krzysztof Dembczynski, Willem Waegeman:
Efficient Algorithms for Set-Valued Prediction in Multi-Class Classification. CoRR abs/1906.08129 (2019) - [i11]Eyke Hüllermeier, Willem Waegeman:
Aleatoric and Epistemic Uncertainty in Machine Learning: A Tutorial Introduction. CoRR abs/1910.09457 (2019) - 2018
- [j23]Michiel Stock, Tapio Pahikkala
, Antti Airola
, Bernard De Baets, Willem Waegeman:
A Comparative Study of Pairwise Learning Methods Based on Kernel Ridge Regression. Neural Comput. 30(8) (2018) - [c20]Stijn Decubber, Thomas Mortier
, Krzysztof Dembczynski
, Willem Waegeman:
Deep F-Measure Maximization in Multi-label Classification: A Comparative Study. ECML/PKDD (1) 2018: 290-305 - [i10]Michiel Stock, Tapio Pahikkala, Antti Airola, Bernard De Baets, Willem Waegeman:
A Comparative Study of Pairwise Learning Methods based on Kernel Ridge Regression. CoRR abs/1803.01575 (2018) - [i9]Willem Waegeman, Krzysztof Dembczynski, Eyke Hüllermeier:
Multi-Target Prediction: A Unifying View on Problems and Methods. CoRR abs/1809.02352 (2018) - 2017
- [c19]Brecht Martens, Diego G. Miralles
, Wouter A. Dorigo
, Willem Waegeman, Niko E. C. Verhoest:
Investigating the control of ocean-atmospheric oscillations over global terrestrial evaporation using a simple supervised learning method. MultiTemp 2017: 1-3 - [c18]Diego G. Miralles
, Matthias Demuzere, Niko E. C. Verhoest, Wouter A. Dorigo
, Christina Papagiannopoulou, Stijn Decubber, Willem Waegeman:
A non-linear data-driven approach to reveal global vegetation sensitivity to climate. MultiTemp 2017: 1-3 - [c17]Christina Papagiannopoulou, Stijn Decubber, Diego G. Miralles
, Matthias Demuzere, Niko E. C. Verhoest, Willem Waegeman:
Analyzing Granger Causality in Climate Data with Time Series Classification Methods. ECML/PKDD (3) 2017: 15-26 - 2016
- [j22]Michiel Stock, Krzysztof Dembczynski
, Bernard De Baets
, Willem Waegeman:
Exact and efficient top-K inference for multi-target prediction by querying separable linear relational models. Data Min. Knowl. Discov. 30(5): 1370-1394 (2016) - [c16]Krzysztof Dembczynski
, Wojciech Kotlowski, Willem Waegeman, Róbert Busa-Fekete, Eyke Hüllermeier:
Consistency of Probabilistic Classifier Trees. ECML/PKDD (2) 2016: 511-526 - [i8]Michiel Stock, Tapio Pahikkala, Antti Airola, Bernard De Baets, Willem Waegeman:
Efficient Pairwise Learning Using Kernel Ridge Regression: an Exact Two-Step Method. CoRR abs/1606.04275 (2016) - [i7]Michiel Stock, Krzysztof Dembczynski, Bernard De Baets, Willem Waegeman:
Exact and efficient top-K inference for multi-target prediction by querying separable linear relational models. CoRR abs/1606.04278 (2016) - 2015
- [j21]E. Colman, Willem Waegeman, Bernard De Baets
, Veerle Fievez
:
Prediction of subacute ruminal acidosis based on milk fatty acids: A comparison of linear discriminant and support vector machine approaches for model development. Comput. Electron. Agric. 111: 179-185 (2015) - [i6]Tapio Pahikkala
, Markus Viljanen, Antti Airola, Willem Waegeman:
Spectral Analysis of Symmetric and Anti-Symmetric Pairwise Kernels. CoRR abs/1506.05950 (2015) - 2014
- [j20]Jan Peters
, Willem Waegeman, Jasper Van doninck
, Els I. Ducheyne, Carlos Calvete
, J. Lucientes, Niko E. C. Verhoest, Bernard De Baets
:
Predicting spatio-temporal Culicoides imicola distributions in Spain based on environmental habitat characteristics and species dispersal. Ecol. Informatics 22: 69-80 (2014) - [j19]Willem Waegeman, Krzysztof Dembczynski, Arkadiusz Jachnik, Weiwei Cheng, Eyke Hüllermeier:
On the bayes-optimality of F-measure maximizers. J. Mach. Learn. Res. 15(1): 3333-3388 (2014) - [j18]Michiel Stock, Thomas Fober, Eyke Hüllermeier, Serghei Glinca
, Gerhard Klebe, Tapio Pahikkala
, Antti Airola
, Bernard De Baets
, Willem Waegeman:
Identification of Functionally Related Enzymes by Learning-to-Rank Methods. IEEE ACM Trans. Comput. Biol. Bioinform. 11(6): 1157-1169 (2014) - [c15]Tapio Pahikkala
, Michiel Stock, Antti Airola
, Tero Aittokallio
, Bernard De Baets
, Willem Waegeman:
A Two-Step Learning Approach for Solving Full and Almost Full Cold Start Problems in Dyadic Prediction. ECML/PKDD (2) 2014: 517-532 - [i5]Michiel Stock, Thomas Fober, Eyke Hüllermeier, Serghei Glinca, Gerhard Klebe, Tapio Pahikkala
, Antti Airola, Bernard De Baets, Willem Waegeman:
Identification of functionally related enzymes by learning-to-rank methods. CoRR abs/1405.4394 (2014) - [i4]Tapio Pahikkala, Michiel Stock, Antti Airola, Tero Aittokallio, Bernard De Baets, Willem Waegeman:
A two-step learning approach for solving full and almost full cold start problems in dyadic prediction. CoRR abs/1405.4423 (2014) - 2013
- [j17]Shinji Fukuda
, Bernard De Baets
, Willem Waegeman, Jan Verwaeren
, Ans M. Mouton:
Habitat prediction and knowledge extraction for spawning European grayling (Thymallus thymallus L.) using a broad range of species distribution models. Environ. Model. Softw. 47: 1-6 (2013) - [j16]Tapio Pahikkala
, Antti Airola
, Michiel Stock, Bernard De Baets
, Willem Waegeman:
Efficient regularized least-squares algorithms for conditional ranking on relational data. Mach. Learn. 93(2-3): 321-356 (2013) - [c14]Krzysztof Dembczynski, Arkadiusz Jachnik, Wojciech Kotlowski, Willem Waegeman, Eyke Hüllermeier:
Optimizing the F-Measure in Multi-Label Classification: Plug-in Rule Approach versus Structured Loss Minimization. ICML (3) 2013: 1130-1138 - [i3]Willem Waegeman, Krzysztof Dembczynski, Weiwei Cheng, Eyke Hüllermeier:
On the Bayes-optimality of F-measure maximizers. CoRR abs/1310.4849 (2013) - 2012
- [j15]Jan Verwaeren
, Willem Waegeman, Bernard De Baets
:
Learning partial ordinal class memberships with kernel-based proportional odds models. Comput. Stat. Data Anal. 56(4): 928-942 (2012) - [j14]Krzysztof Dembczynski
, Willem Waegeman, Weiwei Cheng, Eyke Hüllermeier:
On label dependence and loss minimization in multi-label classification. Mach. Learn. 88(1-2): 5-45 (2012) - [j13]Willem Waegeman, Tapio Pahikkala
, Antti Airola
, Tapio Salakoski, Michiel Stock, Bernard De Baets
:
A Kernel-Based Framework for Learning Graded Relations From Data. IEEE Trans. Fuzzy Syst. 20(6): 1090-1101 (2012) - [c13]Krzysztof Dembczynski
, Willem Waegeman, Eyke Hüllermeier:
An Analysis of Chaining in Multi-Label Classification. ECAI 2012: 294-299 - [c12]Weiwei Cheng, Eyke Hüllermeier, Willem Waegeman, Volkmar Welker:
Label Ranking with Partial Abstention based on Thresholded Probabilistic Models. NIPS 2012: 2510-2518 - [c11]Weiwei Cheng, Krzysztof Dembczynski
, Eyke Hüllermeier, Adrian Jaroszewicz, Willem Waegeman:
F-Measure Maximization in Topical Classification. RSCTC 2012: 439-446 - [i2]Tapio Pahikkala, Antti Airola, Michiel Stock, Bernard De Baets, Willem Waegeman:
Efficient Regularized Least-Squares Algorithms for Conditional Ranking on Relational Data. CoRR abs/1209.4825 (2012) - 2011
- [j12]Willem Waegeman, Bernard De Baets
:
On the ERA ranking representability of pairwise bipartite ranking functions. Artif. Intell. 175(7-8): 1223-1250 (2011) - [j11]Antti Airola
, Tapio Pahikkala
, Willem Waegeman, Bernard De Baets
, Tapio Salakoski:
An experimental comparison of cross-validation techniques for estimating the area under the ROC curve. Comput. Stat. Data Anal. 55(4): 1828-1844 (2011) - [j10]Willem Waegeman, Jan Verwaeren
, Bram Slabbinck, Bernard De Baets
:
Supervised learning algorithms for multi-class classification problems with partial class memberships. Fuzzy Sets Syst. 184(1): 106-125 (2011) - [c10]Willem Waegeman, Tapio Pahikkala
, Antti Airola
, Tapio Salakoski, Bernard De Baets
:
Learning Valued Relations from Data. Eurofuse 2011: 257-268 - [c9]Shinji Fukuda
, Willem Waegeman, Ans M. Mouton, Bernard De Baets
:
Modelling Fish Habitat Preference with a Genetic Algorithm-Optimized Takagi-Sugeno Model Based on Pairwise Comparisons. Eurofuse 2011: 375-387 - [c8]Shinji Fukuda
, Jun Nakajima, Bernard De Baets
, Willem Waegeman, Takahiko Mukai, Ans M. Mouton, Norio Onikura:
A discussion on the accuracy-complexity relationship in modelling fish habitat preference using genetic Takagi-Sugeno fuzzy systems. GEFS 2011: 81-86 - [c7]Willem Waegeman, Bernard De Baets
:
ERA ranking representability: The missing link between ordinal regression and multi-class classification. ISDA 2011: 1188-1193 - [c6]Krzysztof Dembczynski, Willem Waegeman, Weiwei Cheng, Eyke Hüllermeier:
An Exact Algorithm for F-Measure Maximization. NIPS 2011: 1404-1412 - [i1]Willem Waegeman, Tapio Pahikkala, Antti Airola, Tapio Salakoski, Michiel Stock, Bernard De Baets:
A kernel-based framework for learning graded relations from data. CoRR abs/1111.6473 (2011) - 2010
- [j9]Bram Slabbinck, Willem Waegeman, Peter Dawyndt
, Paul De Vos, Bernard De Baets
:
From learning taxonomies to phylogenetic learning: Integration of 16S rRNA gene data into FAME-based bacterial classification. BMC Bioinform. 11: 69 (2010) - [j8]Tapio Pahikkala
, Willem Waegeman, Evgeni Tsivtsivadze, Tapio Salakoski, Bernard De Baets
:
Learning intransitive reciprocal relations with kernel methods. Eur. J. Oper. Res. 206(3): 676-685 (2010) - [j7]Willem Waegeman, Bernard De Baets
:
A transitivity analysis of bipartite rankings in pairwise multi-class classification. Inf. Sci. 180(21): 4099-4117 (2010) - [c5]Dieter Devlaminck, Willem Waegeman, Bruno Bauwens, Bart Wyns, Georges Otte, Luc Boullart, Patrick Santens:
Directional predictions for 4-class BCI data. ESANN 2010 - [c4]Shinji Fukuda
, Norio Onikura, Bernard De Baets
, Willem Waegeman, Ans M. Mouton, Jun Nakajima, Takahiko Mukai:
A genetic Takagi-Sugeno fuzzy system for fish habitat preference modelling. NaBIC 2010: 274-279 - [c3]Krzysztof Dembczynski
, Willem Waegeman, Weiwei Cheng, Eyke Hüllermeier:
Regret Analysis for Performance Metrics in Multi-Label Classification: The Case of Hamming and Subset Zero-One Loss. ECML/PKDD (1) 2010: 280-295 - [c2]Tapio Pahikkala
, Willem Waegeman, Antti Airola, Tapio Salakoski, Bernard De Baets
:
Conditional Ranking on Relational Data. ECML/PKDD (2) 2010: 499-514 - [c1]Antti Airola, Tapio Pahikkala, Willem Waegeman, Bernard De Baets, Tapio Salakoski:
A comparison of AUC estimators in small-sample studies. MLSB 2010: 3-13 - [p1]Willem Waegeman, Bernard De Baets:
A Survey on ROC-based Ordinal Regression. Preference Learning 2010: 127-154
2000 – 2009
- 2009
- [j6]Willem Waegeman, Bernard De Baets
, Luc Boullart:
Kernel-based learning methods for preference aggregation. 4OR 7(2): 169-189 (2009) - [j5]Willem Waegeman:
Learning to rank: a ROC-based graph-theoretic approach. 4OR 7(4): 399-402 (2009) - 2008
- [j4]Willem Waegeman, Bernard De Baets
, Luc Boullart:
On the scalability of ordered multi-class ROC analysis. Comput. Stat. Data Anal. 52(7): 3371-3388 (2008) - [j3]Willem Waegeman, Johannes Cottyn
, Bart Wyns, Luc Boullart, Bernard De Baets
, Lieva Van Langenhove
, Jan Detand
:
Classifying carpets based on laser scanner data. Eng. Appl. Artif. Intell. 21(6): 907-918 (2008) - [j2]Willem Waegeman, Bernard De Baets
, Luc Boullart:
Learning layered ranking functions with structured support vector machines. Neural Networks 21(10): 1511-1523 (2008) - [j1]Willem Waegeman, Bernard De Baets
, Luc Boullart:
ROC analysis in ordinal regression learning. Pattern Recognit. Lett. 29(1): 1-9 (2008)
Coauthor Index

manage site settings
To protect your privacy, all features that rely on external API calls from your browser are turned off by default. You need to opt-in for them to become active. All settings here will be stored as cookies with your web browser. For more information see our F.A.Q.
Unpaywalled article links
Add open access links from to the list of external document links (if available).
Privacy notice: By enabling the option above, your browser will contact the API of unpaywall.org to load hyperlinks to open access articles. Although we do not have any reason to believe that your call will be tracked, we do not have any control over how the remote server uses your data. So please proceed with care and consider checking the Unpaywall privacy policy.
Archived links via Wayback Machine
For web page which are no longer available, try to retrieve content from the of the Internet Archive (if available).
Privacy notice: By enabling the option above, your browser will contact the API of archive.org to check for archived content of web pages that are no longer available. Although we do not have any reason to believe that your call will be tracked, we do not have any control over how the remote server uses your data. So please proceed with care and consider checking the Internet Archive privacy policy.
Reference lists
Add a list of references from ,
, and
to record detail pages.
load references from crossref.org and opencitations.net
Privacy notice: By enabling the option above, your browser will contact the APIs of crossref.org, opencitations.net, and semanticscholar.org to load article reference information. Although we do not have any reason to believe that your call will be tracked, we do not have any control over how the remote server uses your data. So please proceed with care and consider checking the Crossref privacy policy and the OpenCitations privacy policy, as well as the AI2 Privacy Policy covering Semantic Scholar.
Citation data
Add a list of citing articles from and
to record detail pages.
load citations from opencitations.net
Privacy notice: By enabling the option above, your browser will contact the API of opencitations.net and semanticscholar.org to load citation information. Although we do not have any reason to believe that your call will be tracked, we do not have any control over how the remote server uses your data. So please proceed with care and consider checking the OpenCitations privacy policy as well as the AI2 Privacy Policy covering Semantic Scholar.
OpenAlex data
Load additional information about publications from .
Privacy notice: By enabling the option above, your browser will contact the API of openalex.org to load additional information. Although we do not have any reason to believe that your call will be tracked, we do not have any control over how the remote server uses your data. So please proceed with care and consider checking the information given by OpenAlex.
last updated on 2024-10-07 22:16 CEST by the dblp team
all metadata released as open data under CC0 1.0 license
see also: Terms of Use | Privacy Policy | Imprint