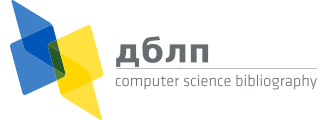


Остановите войну!
for scientists:


default search action
ICLR Workshop 2014: Banff, AB, Canada
- Yoshua Bengio, Yann LeCun:
2nd International Conference on Learning Representations, ICLR 2014, Banff, AB, Canada, April 14-16, 2014, Workshop Track Proceedings. 2014
Oral Presentations
- Sida I. Wang, Roy Frostig, Percy Liang, Christopher D. Manning:
Relaxations for inference in restricted Boltzmann machines. - Ashutosh Modi, Ivan Titov:
Learning Semantic Script Knowledge with Event Embeddings. - Yunlong He, Koray Kavukcuoglu, Yun Wang, Arthur Szlam, Yanjun Qi:
Unsupervised Feature Learning by Deep Sparse Coding. - Ouais Alsharif, Joelle Pineau:
End-to-End Text Recognition with Hybrid HMM Maxout Models.
Poster Presentations
- Wenpeng Yin, Hinrich Schütze:
Deep Learning Embeddings for Discontinuous Linguistic Units. - David Eigen, Marc'Aurelio Ranzato, Ilya Sutskever:
Learning Factored Representations in a Deep Mixture of Experts. - Cédric Lagnier, Simon Bourigault, Sylvain Lamprier, Ludovic Denoyer, Patrick Gallinari:
Learning Information Spread in Content Networks. - Gabriella Contardo, Ludovic Denoyer, Thierry Artières, Patrick Gallinari:
Learning States Representations in POMDP. - Irina Sergienya, Hinrich Schütze:
Distributional Models and Deep Learning Embeddings: Combining the Best of Both Worlds. - Mathias Berglund, Tapani Raiko:
Stochastic Gradient Estimate Variance in Contrastive Divergence and Persistent Contrastive Divergence. - David Buchaca Prats
, Enrique Romero, Ferran Mazzanti, Jordi Delgado
:
Stopping Criteria in Contrastive Divergence: Alternatives to the Reconstruction Error. - Michael Tetelman:
Continuous Learning: Engineering Super Features With Feature Algebras. - Sherjil Ozair, Li Yao, Yoshua Bengio:
Multimodal Transitions for Generative Stochastic Networks. - Anjan Nepal, Alexander Yates:
Factorial Hidden Markov Models for Learning Representations of Natural Language. - Samuel R. Bowman:
Can recursive neural tensor networks learn logical reasoning? - Jianshu Chen, Li Deng:
A New Method for Learning Deep Recurrent Neural Networks. - Dimitris Athanasakis, John Shawe-Taylor, Delmiro Fernandez-Reyes:
Learning Non-Linear Feature Maps, With An Application To Representation Learning. - Luis Gonzalo Sánchez Giraldo, José C. Príncipe:
Rate-Distortion Auto-Encoders. - Andrew S. Davis, Itamar Arel:
Low-Rank Approximations for Conditional Feedforward Computation in Deep Neural Networks. - Aleksandr Y. Aravkin, Anna Choromanska, Dimitri Kanevsky, Tony Jebara:
Semistochastic Quadratic Bound Methods for Convex and Nonconvex Learning Problems. - Patrick C. Connor, Thomas Trappenberg:
An Architecture for Distinguishing between Predictors and Inhibitors in Reinforcement Learning. - Omry Yadan, Keith Adams, Yaniv Taigman, Marc'Aurelio Ranzato:
Multi-GPU Training of ConvNets. - Thomas Paine, Hailin Jin, Jianchao Yang, Zhe Lin, Thomas S. Huang:
GPU Asynchronous Stochastic Gradient Descent to Speed Up Neural Network Training. - Edouard Oyallon, Stéphane Mallat, Laurent Sifre:
Generic Deep Networks with Wavelet Scattering. - Brody Huval, Adam Coates, Andrew Y. Ng:
Deep learning for class-generic object detection. - Karen Simonyan, Andrea Vedaldi, Andrew Zisserman:
Deep Inside Convolutional Networks: Visualising Image Classification Models and Saliency Maps. - Alexey Dosovitskiy, Jost Tobias Springenberg, Thomas Brox:
Unsupervised feature learning by augmenting single images. - Balázs Kégl:
Correlation-based construction of neighborhood and edge features. - Nan Wang, Laurenz Wiskott, Dirk Jancke:
Modeling correlations in spontaneous activity of visual cortex with centered Gaussian-binary deep Boltzmann machines. - David Eigen, Jason Tyler Rolfe, Rob Fergus, Yann LeCun:
Understanding Deep Architectures using a Recursive Convolutional Network. - Yalong Bai, Kuiyuan Yang, Wei Yu, Wei-Ying Ma, Tiejun Zhao:
Learning High-level Image Representation for Image Retrieval via Multi-Task DNN using Clickthrough Data. - Judy Hoffman, Eric Tzeng, Jeff Donahue, Yangqing Jia, Kate Saenko, Trevor Darrell:
One-Shot Adaptation of Supervised Deep Convolutional Models. - Jost Tobias Springenberg, Martin A. Riedmiller:
Improving Deep Neural Networks with Probabilistic Maxout Units. - Honghao Shan, Garrison W. Cottrell:
Efficient Visual Coding: From Retina To V2. - Sergey M. Plis, R. Devon Hjelm, Ruslan Salakhutdinov, Vince D. Calhoun:
Deep learning for neuroimaging: a validation study. - Yiyi Liao, Yue Wang, Yong Liu:
Image Representation Learning Using Graph Regularized Auto-Encoders. - Mohammad Ali Keyvanrad, Mohammad Pezeshki, Mohammad Ali Homayounpour:
Deep Belief Networks for Image Denoising. - Taichi Kiwaki, Takaki Makino, Kazuyuki Aihara:
Approximated Infomax Early Stopping: Revisiting Gaussian RBMs on Natural Images.

manage site settings
To protect your privacy, all features that rely on external API calls from your browser are turned off by default. You need to opt-in for them to become active. All settings here will be stored as cookies with your web browser. For more information see our F.A.Q.